Abstract
Research has highlighted the relevance of biological measures in explaining antisocial behavior, but the inclusion of such measures in clinical practice is lagging behind. According to the integrative biopsychosocial model, biological measures should be studied together with psychological and social-environmental factors. In this data-driven study, we applied this comprehensive model to explain non-violent and violent delinquency of 876 at-risk youth (715 male, 9–27 years), by combining nine biological (autonomic-nervous-system; endocrinological), nine psychological, and seven social-environmental measures. Using latent-class-regression analysis we uncovered four distinct psychologically-driven biological clusters, which differed in non-violent and violent delinquency-risk, moderated by social-environmental variables: a biological–psychopathic traits; low problem; high problem; and biological–reactive group. Individual vulnerabilities to (non-)violent delinquency depended on social-environmental context that differed between clusters. These findings highlight the importance of biological and psychological factors, in the context of social-environmental factors, in explaining (non)-violent delinquency.
1. Introduction
Adolescents who show delinquency, such as non-violent and violent offending, are at high risk of developing problems throughout their lifetime, and cause major societal costs (Brazil et al., 2018). Interventions aimed to reduce or prevent such antisocial behaviors often take a ‘one size fits all’ approach, meaning that interventions are the same across individuals. However, there is considerable heterogeneity in antisocial behavior and its biological, psychological, and social-environmental underpinnings. Each of these factors may account for a significant part of the variation in the course, development, and prognosis of antisocial behavior (Moffitt, 2018). Therefore, in the current study, we apply the integrative ‘biopsychosocial’ model to examine how biological and psychological factors, impacted by social-environmental factors, explain the risk of non-violent and violent delinquency in a heterogeneous group of at-risk youth.
Delinquent behavior is not uncommon in adolescence, and can vary from mild and temporary delinquent behavior to severe and sometimes persistent delinquent behavior (Moffitt, 2018). An important distinction to make in this respect is between non-violent and violent delinquency. Non-violent delinquency includes behaviors such as (petty) theft or vandalism. Such behaviors thus do not include force or do not physically injure other individuals. Violent delinquency, however, includes an aggressive component such as force or physical injury. Examples included armed robbery, threatening someone with a weapon, or forcing someone into sexual activity. Gaining more knowledge on both non-violent and violent delinquency is highly relevant both on a societal and scientific level. While violent delinquency has a costly impact on society primarily because of serious physical and psychological harm inflicted upon victims, non-violent delinquency has a costly impact on society primarily due to its impact on general sense of safety and trust and consequences on a monetary level (Brand and Price, 2000, Cohen and Cohen, 2004). Moreover, because of these differences in the nature and the consequences of non-violent and violent delinquency, it is likely that these delinquency types are differentially driven by underlying mechanisms. That is, to predict which adolescents are at risk of showing non-violent and violent delinquency, it is important to understand how biological, psychological, and social factors contribute to these two behaviors. This allows to gauge whether different biopsychosocial ‘profiles’ may be distinguished for youth showing non-violent and/or violent delinquency.
The biopsychosocial model is a leading model to understand adolescent antisocial behavior in general. This model states that antisocial behavior arises from an interplay between biological, psychological, and social factors (Dodge and Pettit, 2003, Jansen, 2022, Van Goozen et al., 2022). Prior and more recent calls stress the importance of considering neurobiological factors together with psychological and social and environmental factors, to personalize intervention and prevention efforts for youth who show delinquency (Beauchaine et al., 2008, Glenn, 2019, Glenn and McCauley, 2019, Jansen, 2022, Popma and Raine, 2006). Below we give a brief background on the most studied neurobiological, psychological, and social and environmental factors related to antisocial behavior in general. However, it is important to realize that in the biopsychosocial model, these measures are considered interrelated and can influence each other (Dodge and Pettit, 2003, Jansen, 2022, Van Goozen et al., 2022).
A wealth of research has already shown the relevance of neurobiological measures in explaining antisocial tendencies. Aggression, delinquency, and conduct problems have consistently been associated with resting heart rate (HR), as well as parasympathetic and sympathetic-nervous system-specific measures such as respiratory sinus arrythmia (RSA; a parasympathetic measure) and the pre-ejection period (PEP; a sympathetic measure), respiration rate (RR; a general measure of arousal), and skin conductance levels (SCL; a sympathetic measure; Beauchaine et al., 2007; Beauchaine et al., 2001; Blankenstein et al., 2021; Blankenstein et al., 2022; Cornet et al., 2014; MacDougall et al., 2019; Marsh et al., 2008; Oldenhof et al., 2018; Ortiz and Raine, 2004; Popma et al., 2006; Portnoy and Farrington, 2015; Raine et al., 1990, Raine et al., 1995). Likewise, basal testosterone has been related to aggression and social dominance (Archer, 2006, Blankenstein et al., 2021, Carré and Archer, 2018, Dekkers, 2018, Geniole et al., 2019, Peper et al., 2018, Rowe et al., 2004), and basal cortisol and the cortisol awakening response have been linked to aggression and conduct problems (Blankenstein et al., 2021, Blankenstein et al., 2022, Dekkers, 2018, McBurnett et al., 2000, Mehta and Josephs, 2010, Yi-Zhen and Jun-Xia, 2009). To understand the risk of displaying non-violent and violent delinquency in youth, these basal autonomic nervous system and neuroenocrinological measures are thus relevant to consider. However, it is important to note that in a vacuum, neurobiological measures have limited predictive value (Alink et al., 2008), and in isolation these measures cannot distinguish between adolescents who are at risk for non-violent and violent delinquency.
Psychological characteristics that relate to delinquency in youth include psychopathic traits such as callous-unemotionel traits, a lack of affective empathy, and reactive and proactive aggression (Marsee et al., 2005, van Zonneveld et al., 2019, van Zonneveld et al., 2017, Vaughn et al., 2008). Other relevant characteristics include clinical phenomena such as comorbid internalizing problems, inattentiveness, and hyperactivity (Pardini et al., 2006, Wallander, 1988). It has been proposed that such psychological characteristics increases susceptibility towards antisocial behavior when combined with aberrant biological functioning, such as physiological under- or over-arousal (for review, see van Hazebroek et al., 2019). That is, some displays of antisocial behavior can arise from under-arousal such that the biological stress system is attenuated under novel, threatening, or stressful situations. This insensitivity to stress may lead individuals to increase their arousal to more comfortable levels, leading to heightened levels of sensation seeking or fearlessness, and consequently, antisocial behavior (Blair, 2013, Raine and Liu, 1998, Zuckerman, 1990, Zuckerman and Riskind, 2000). On the other hand, biological over-arousal, reflected in a heightened sensitivity to stressful events, may lead individuals to more quickly engage in antisocial behaviors when provoked (Blair, 2013, Fanti, 2018, Fanti et al., 2019, Scarpa and Raine, 1997). According to arousal theories, psychological characteristics such as callous-unemotional traits, proactive aggression and lack of empathy have been related to low levels of biological arousal, while characteristics such as internalizing problems, impulsivity, and reactive aggression have been related to high levels of biological arousal (for reviews, see Blair, 2013; van Hazebroek et al., 2019). Finally, in addition to arousal, higher levels of testosterone have been related to higher levels of social dominance and aggressive conduct (Archer, 2006, Blankenstein et al., 2021, Carré and Archer, 2018, Dekkers, 2018, Geniole et al., 2019, Peper et al., 2018, Rowe et al., 2004). Thus, certain combinations of psychological characteristics and biological measures may make one susceptible to show delinquent behavior.
Importantly, in line with the biopsychosocial and many related models, delinquency may also depend on social and environmental factors (for review, see Belsky et al., 2007; Blankenstein et al., 2022; Jansen, 2022; McCrory et al., 2022; van Hazebroek et al., 2019; Zuckerman and Riskind, 2000). For example, an adolescent may have low levels of empathy, high levels of testosterone, and a low resting heart rate, but this combination may result in (non-)violent delinquency under socially adverse circumstances only such as living in a disadvantaged neighborhood. Yet, in more fortunate circumstances, delinquency may not present itself. Important social and environmental factors that put adolescents at risk for delinquency are disadvantaged (socio-demographic) circumstances, such as a history of maltreatment (neglect, abuse), low socio-economic status, being part of a marginalized group, lower intelligence, and other external influences such as substance use (D’Amico et al., 2008, McCrory et al., 2022, Moffitt, 2018, Paradise and Mari Cauce, 2003, Shaw and McKay, 1942). Thus, whether a psychological-biological susceptibility for antisocial behavior manifests in actual non-violent or violent delinquent behavior, may depend on social and environmental circumstances.
Finally, it is important to realize that biological, psychological, and social and environmental factors are reciprocally interrelated and can impact each other. For instance, substance use can be seen as an environmental factor, but is also linked to alterations in biological functioning and psychological functioning. Thus, to predict which adolescents are at risk of showing non-violent and violent delinquency, it is necessary to integrate biological, psychological, and social and environmental factors, as well as examine individual differences in these factors and delinquency risk. The biopsychosocial model proposes such an integrative approach.
A valuable technique to apply the biopsychosocial model for explaining the heterogeneity in delinquency is to use a cluster-based method. Prior research has found relevant clusters of individuals who vary in severity of antisocial behavior and psychological and social-environmental indicators (Chng et al., 2016, Decuyper et al., 2013, Dembo et al., 2011, Geluk et al., 2014, Wareham et al., 2009). The integration of several risk factors for antisocial behavior has proven to be especially relevant, as adolescents with a greater number of risk factors were found to show more severe antisocial behavior (Mulder et al., 2012, Vincent et al., 2003). A recent study successfully integrated biological, psychological, and social environmental factors, to define subgroups of detained juveniles, in the context of predicting reoffending risk following release from a juvenile justice institution (de Ruigh et al., 2021). By using latent class regression analysis (LCRA; Magidson and Vermunt, 2002), three relevant subgroups in relation to future reoffending behavior were identified, for which relationships between neurobiological factors and type of reoffending (non, non-violent, or violent recidivism) differed. Moreover, based on this LCRA model individual risk profiles could be reliably estimated. This integration of neurobiological measures in the context of psychological and social measures has thus been shown to improve prediction of recidivism.
In the current study we aim to extend this research by combining five independent study samples of youth (total N = 876; including participants from de Ruigh et al., 2021) that include neurobiological, psychological, and social environmental measures. In addition our samples are heterogeneous, ranging from adolescents referred to a diversion program for a minor offense (such as petty theft) to adolescents referred to closed youth care or juvenile justice institutions due to serious antisocial behavior. Rather than focusing on future reoffending risk, this research is cross-sectional and focuses on explaining delinquency, and thus focuses on the probability of non, non-violent, and violent delinquency. We took an explorative, data-driven approach using LCRA to create psychologically-driven biological clusters of youth, which differ in delinquency outcomes, moderated by social and environmental variables (Belsky et al., 2007, Blankenstein et al., 2022, Jansen, 2022, McCrory et al., 2022, van Hazebroek et al., 2019, Zuckerman and Riskind, 2000). We expected to uncover a number of clusters of youth differing in biological-psychological characteristics; and that these clusters of youth would show different probabilities of displaying non-violent and violent delinquency. Finally, we expected that these probabilities would be impacted by the social and environmental factors.
2. Methods
2.1. Participants
Participants came from five independent study samples. Data were collected between 2003 and 2016 by our department in urban areas of the Netherlands. All studies were approved by their respective ethical committees and all participants and caregivers (in the case of minors) gave written informed consent. The complete sample included 876 participants between 9 and 27 years old (although the majority was 12 years and older, Mage = 17.67, SDage = 3.20, 161 females, 715 males). See Table 1 for age and sex statistics for the total sample and each sample separately.
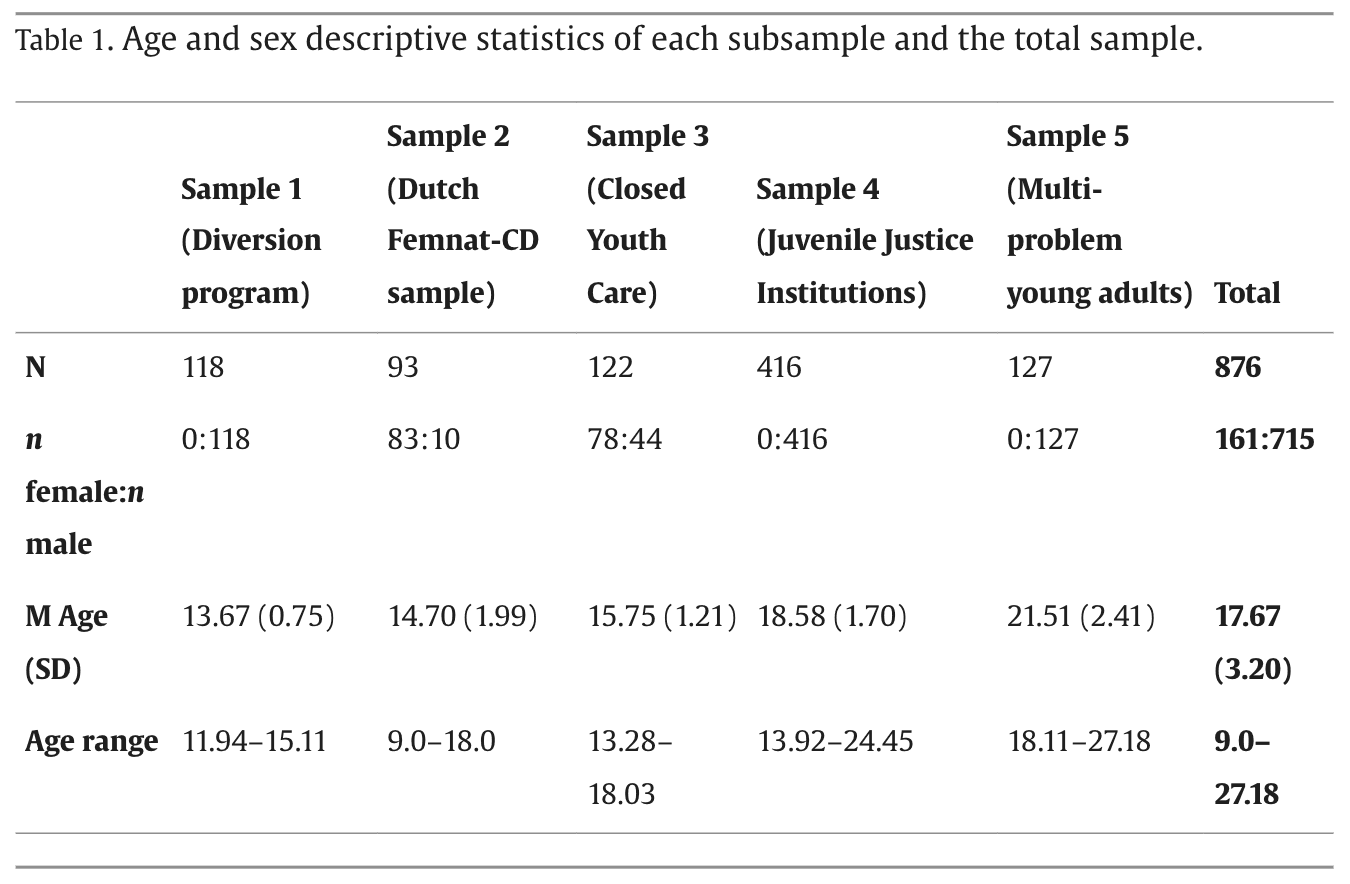
Sample 1 included 118 male adolescents who were referred to a delinquency diversion program after having committed a minor offense (and non-delinquent controls who were not included in the current study; Popma et al., 2006; Popma et al., 2007). Sample 2 consisted of 93 adolescents (girls and boys) with conduct disorder from the Dutch portion of a European multi-center study (Freitag, 2014, Oldenhof et al., 2018). Sample 3 consisted of 122 adolescents (girls and boys) in a closed treatment facility for compulsory treatment due to severe antisocial behavior (Jambroes et al., 2018). Sample 4 included 416 adolescent boys in juvenile justice institutions, referred because of severe behavioral problems or criminal offenses (de Ruigh et al., 2021). Finally, sample 5 included 127 multi-problem adolescent and young adult males who struggle with a variety of psychosocial problems and have a history of juvenile justice problems (Zijlmans et al., 2019, Zijlmans et al., 2018).
2.2. Measures
There was considerable overlap in measures included in each sample. Nonetheless, for some psychological and social factors measurement instruments differed across samples. When different samples assessed the same construct but with different measures we fostered comparability across samples. Table S1 shows data assessment strategy per variable and sample and Table S2 provides data availability per variable and sample. Independent variables were selected based on scientific literature review but constrained to their availability in the datasets. We followed a pragmatic approach necessary for practical implementation, and thus included variables readily available and simple to evaluate. Finally, in case of missing data in the independent variables we applied multiple imputations to yield a complete dataset to run our LCRA on. See Appendix B in the supplementary materials for details on the multiple imputation procedure.
2.3. Outcome variable: delinquency
Delinquency was assessed using self-report questionnaires, semi-structured diagnostics interviews, or official registrations of offenses. For our main outcome variable, we created a categorical measure which was the same across samples, with three levels: non delinquency, non-violent delinquency, and violent delinquency, assessed over an extended period of time. If a participant had never committed an offense in their lifetime, this was coded as non-delinquency. If a participant committed a non-violent offense, this was coded as non-violent delinquency, and if a participant ever committed a violent offense this was coded as violent delinquency. If participant committed both a non-violent and violent offense, this was coded as violent delinquency, because the heaviest offense was leading. This was the case for the majority of individuals (94 %), meaning there were very few participants who engaged exclusively in violent, but not non-violent, delinquency.
2.4. Predictor variables
2.4.1. Neurobiological measures
Neurobiological measures included resting heart rate (HR), respiratory sinus arrhythmia (RSA), pre-ejection periods (PEP), respiration rate (RR), skin conductance levels (SCL), basal testosterone, basal cortisol, and the cortisol awakening response (CAR; total volume and reactivity measure). Assessment was comparable across samples: the VU-AMS system was used to assess ANS functioning and afternoon saliva was used to assess testosterone and cortisol. Morning saliva samples were used to assess the CAR. Specific data collection procedures and analysis methods for each sample can be found in the original respective papers (see Participants for references). In the Supplementary Materials (Appendix A; see also Blankenstein et al., 2021) we briefly describe assessment protocols across samples.
2.4.2. Psychological variables
Reactive and proactive aggression were assessed with the Reactive and Proactive Aggression questionnaire (RPQ; Raine et al., 2006), The RPQ assesses 23 proactive and reactive aggressive actions on a three-point scale (0: never, 1: sometimes, 2: often; examples: ‘Got angry when I did not get my way’ (reactive), ‘Taken things from other students’ (proactive).
Externalizing problems, Internalizing problems and Attention problems were assessed via Achenbach self-report questionnaires (Achenbach et al., 2011, Achenbach and Rescorla, 2001, Rescorla and Achenbach, 2004), which are scored on a three-point Likert scale (0: never, 1: sometimes, 2: often; examples: ‘I don’t keep by the rules at school/work or somewhere else’ (Externalizing), ‘I am too anxious or scared’ (Internalizing), ‘I have trouble concentrating or paying attention’ (Attention). We used the categorical variables Typical, Borderline, and Clinical.
Psychopathic traits were assessed via the Youth Psychopathic Index – short version (YPI-sv; samples 2, 3, 4, 5; van Baardewijk et al., 2010), The YPI-sv assessed 18 psychopathic traits, on a four-point scale (ranging from 0: Does not apply at all, to 3: Applies very well). The YPI-sv includes three subscales: Interpersonal (example: ‘I have the ability to con people by using my charm and smile’), Affective (example: ‘I think that crying is a sign of weakness even if no one sees you’) and Behavioral (example: ‘It often happens that I do things without thinking ahead’).
Empathy was measured with the Empathic Concern subscale of the Interpersonal Reactivity Index (IRI-EC; Davis, 1983) or with the Index of Empathy in Children and Adolescents (IECA; Bryant, 1982). The IRI-EC assesses "other-oriented" feelings of sympathy and concern for unfortunate others and includes six items on a five-point scale ranging from 1 (does not describe me well) to 5 (describes me very well; example: ‘I often have tender, concerned feelings for people less fortunate than me’). The IECA includes 7 items such as 'Seeing a (girl/boy) cry makes me feel like crying' on a four-point scale ranging from 1 (not correct at all) to 4 (completely correct). We created an Empathy categorical variable with three levels: Low, Medium, and High Empathy, by calculating fractional rank percentages across samples. Low empathy reflects the lowest 33.3 % empathy scores, Medium empathy the 33.4 %–66.6 % range of empathy scores, and High the ≥66.7 % range of empathy scores.
2.4.3. Social-environmental variables
IQ was estimated from the Vocabulary and Block Design subscales of the WISC-III or WAIS. IQ was categorized under social and environmental variables because research suggest that variation in IQ is significantly affected by typical environmental differences throughout one’s life, such as the availability of learning resources, parenting, social class, healthcare, and nutrition (for a review, see Sauce and Matzel, 2018). Substance use (specifically, alcohol, tobacco, and cannabis use) was assessed using self-report questionnaires or semi-structured diagnostic interviews. Variables included in the data harmonization included whether 1) individuals ever used alcohol, tobacco, and cannabis, and if so, 2) how frequently they used these substances. These variables were submitted to a latent class analyses to harmonize data across samples, by creating groups of individuals differing in substance use. See supplementary materials (Appendix C; Table S3) for details on this LCA. The LCA revealed three groups of users: Sporadic users, Moderate users, and Frequent users. The resulting categorical substance use variable with these three levels were used in subsequent analyses.
Childhood maltreatment was assessed via retrospective questionnaires or semi-structured diagnostic interviews. Data were harmonized by structuring items into four categories: Neglect, Physical Abuse, Sexual Abuse, and Emotional Abuse. Based on these categories, for each sample we assessed whether participants were ever neglected, physically abused, sexually abused, and emotionally abused in their lifetime. These dichotomous variables were used in the subsequent analyses.
Ethnicity was based on participants’ biological parents’ country of birth. Ethnicity was coded as Dutch (both parents born in the Netherlands), Western (at least one parent born in a Western country), and Non-Western (at least one parent born in a non-Western country). Non-Western ethnicity was considered a reflection of marginalized groups in the Netherlands.
Socio-economic status (SES) was estimated by recoding the four digits of participants’ postal codes of the address where they had lived the longest into low, middle, or high SES, based on neighborhood income data from the Central Bureau of Statistics of the Netherlands. These postal codes consist of a number of connected streets that generally attract the same type of households. Hence, the current measure is a crude estimation of participants’ SES based on their neighborhood.
2.5. Latent class regression analysis
To meet our research aims we used a comprehensive model to create latent clusters, which differ on their psychological indicators and biological markers. These latent clusters may be differentially related to delinquency outcomes (non, non-violent, violent delinquency) and moderated by social environmental factors (see Fig. 1 for a conceptual overview of this approach). Analyses were conducted in Latent Gold 4.0 (Vermunt and Magidson, 2002).
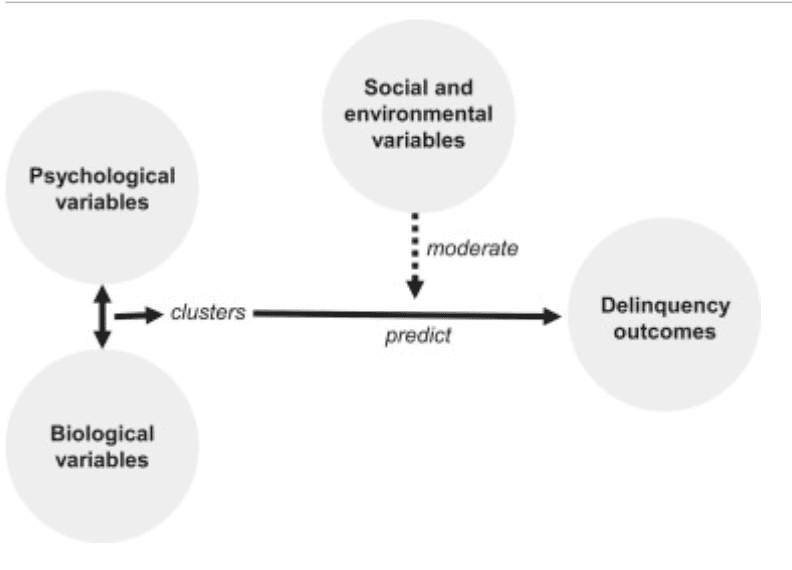
Fig. 1. Conceptual, schematic overview of the one-step Latent Class Regression Analysis. We predicted delinquency risk (non-delinquency, non-violent delinquency, violent delinquency) from psychologically-driven biological clusters, moderated by social and environmental variables.
We used a latent class regression analysis for three reasons (see also de Ruigh et al., 2021). First, our main aim is to predict delinquency risk by a set of biological markers, informed by psychological variables. We expected that this prediction is moderated by combinations of social and environmental variables. The LCRA is such a moderation analysis. Instead of several separate univariate moderation analyses, the LCRA reveals latent clusters characterized by combinations of social and environmental variables that optimally moderate the different relationships between psychologically-informed biological markers and delinquency categories.
Second, by using LCRA because in this approach we can form clusters with explanatory value for delinquency in a one-step model. This approach differs from a classic latent class analysis model, where one first forms clusters, and then tests whether they predict delinquency. In this former LCA approach, the clusters that are created may not be related to delinquency, or may not differ in delinquency risk. With LCRA, by including delinquency as an outcome variable, we immediately form clusters that are optimized to predict delinquency risk.
Third, we used LCRA because we can form clusters based on biological markers and incorporate psychological variables within those clusters in the same model. The advantage of this is that one can take into account that the influence of psychological variables may differ within the neurobiological clusters.
Finally, it should be noted that latent class regression analysis is an exploratory data analysis in which the emphasis is on describing differences between latent clusters on a combination of variables. Therefore, the focus is not on the significance level of individual parameters and no corrections for multiple testing were applied.
The biological markers were the nine continuous measures Heart Rate, Pre-Ejection Period, Respiratory Sinus Arrythmia, Respiration Rate, Skin Conductance Level, Testosterone, Cortisol, and the Cortisol Awakening Response (CAR; total secretion of cortisol during awakening: Area Under the Curve with respect to the ground [CAR AUCg]; and cortisol reactivity: Area Under the Curve with respect to the increase [CAR AUCi]). The psychological variables were the continuous measures YPI Behavioral, YPI Interpersonal, YPI Affective, RPQ Proactive aggression, RPQ Reactive aggression, and the categorical measures Empathy, Externalizing, Internalizing, and Attention problems. The social and environmental variables were Age (continuous), Sex (dichotomous), IQ (continuous), Ethnicity, SES (categorical), Neglect, Physical Abuse, Sexual Abuse, Emotional Abuse (dichotomous), and Substance Use (categorical). In the selection of indicators for the latent class regression model, we consciously chose indicators that measure different psychological constructs. Naturally, these constructs are theoretically related, but we expect, and as far as known from the literature, this relationship to be weak to moderate and not strong enough to cause multicollinearity in the latent class regression analysis. Indeed, none of the correlations between the indicators (pre-imputation) approached or exceeded r =.8. Moreover, because LCRA uses Bayesian statistics (unlike frequentist statistics which is usually applied in multiple regression), potential multicollinearity problems would be encountered in estimating the model. This was not the case.
The LCRA model is depicted in equation 1 below. We fitted a latent class model in which the scores on the biological markers of the participants, i, i=1,…,N, were covariates collected in vector, z, that affect the latent class variable, x, x=1,…, K. The latent classes are in turn regressed on the psychological indicators collected in vector, y, yt=1,…,T. The social and environmental variables may function as moderating variables, protecting or exacerbating the effect of cluster membership to delinquency. Since delinquency is a categorical variable consisting of three levels, a multinomial regression model with effect coding was used. This means that a regression weight was estimated for each category with the restriction that the three effects sum to zero. We preferred effect coding over, for example, contrast or dummy coding because the regression weights show how much the respective category deviates from the average. This allows for the interpretation of each regression weight individually.

We fitted models with one to six classes and compared the relative fit indices, the entropy, the bootstrapped −2LL and the interpretation of the classes to choose the number of classes. For syntax, see Appendix D in the supplementary materials.
3. Results
3.1. Latent classes representing differentiated psychological subgroups based on biological markers
First we compared models to decide on the number of clusters. Choosing the number of clusters is often delicate, and it is here. The relative fit indices, BIC, AIC, AIC3, CAIC, and SABIC all use the log likelihood and put – although in a different way – a penalty on the number of the parameters of the model (Sen and Bradshaw, 2017). In our case these indices did not lead to a clear, unambiguous solution, as if often the case (Sinha et al., 2021; see Table S4 for overview of fit indices for the one-to-six cluster models). The BIC and consistent AIC (CAIC) had lowest values for the four-cluster model. The sample size adjusted BIC (SABIC), had the lowest value in the six-cluster model. The bootstrapped −2LL differences were all significant for subsequent models. The entropy was.86 for the four-cluster model and.81 for the six-cluster model, favoring the four-cluster solution. Together, we proceeded with the four-cluster model which showed the best balance between model complexity and interpretability.
Next we examined the biological covariates and psychological indicators of each cluster in the four-cluster solution (Fig. 2; see Table S5 for covariate and indicator means, and Table S6 for covariate and indicator parameter estimates). The results of the biological covariates show that there were significant effects for PEP, Testosterone, and the CAR AUCg (total volume). This means that these variables differed significantly between two or more clusters (see Fig. 3A-C for raw biological data per cluster, with box plots superimposed). For PEP, the mean was significantly higher in cluster 4 (compared to the other clusters). For Testosterone, the mean was significantly higher in cluster 1 and significantly lower in cluster 4. Finally the mean of CAR AUCg (total volume) was significantly lower in cluster 1 and 2 and significantly higher in cluster 4. The psychological indicators, together with the biological covariates define the clusters (see Fig. 4D-L) for raw psychological data with box plots superimposed, see Table S5, Table S6). All psychological indicators were significant as indicated by the explained variance of the classes (R2). Based on the clusters’ biological and psychological characteristics, we decided to label the clusters (see also Fig. 2):
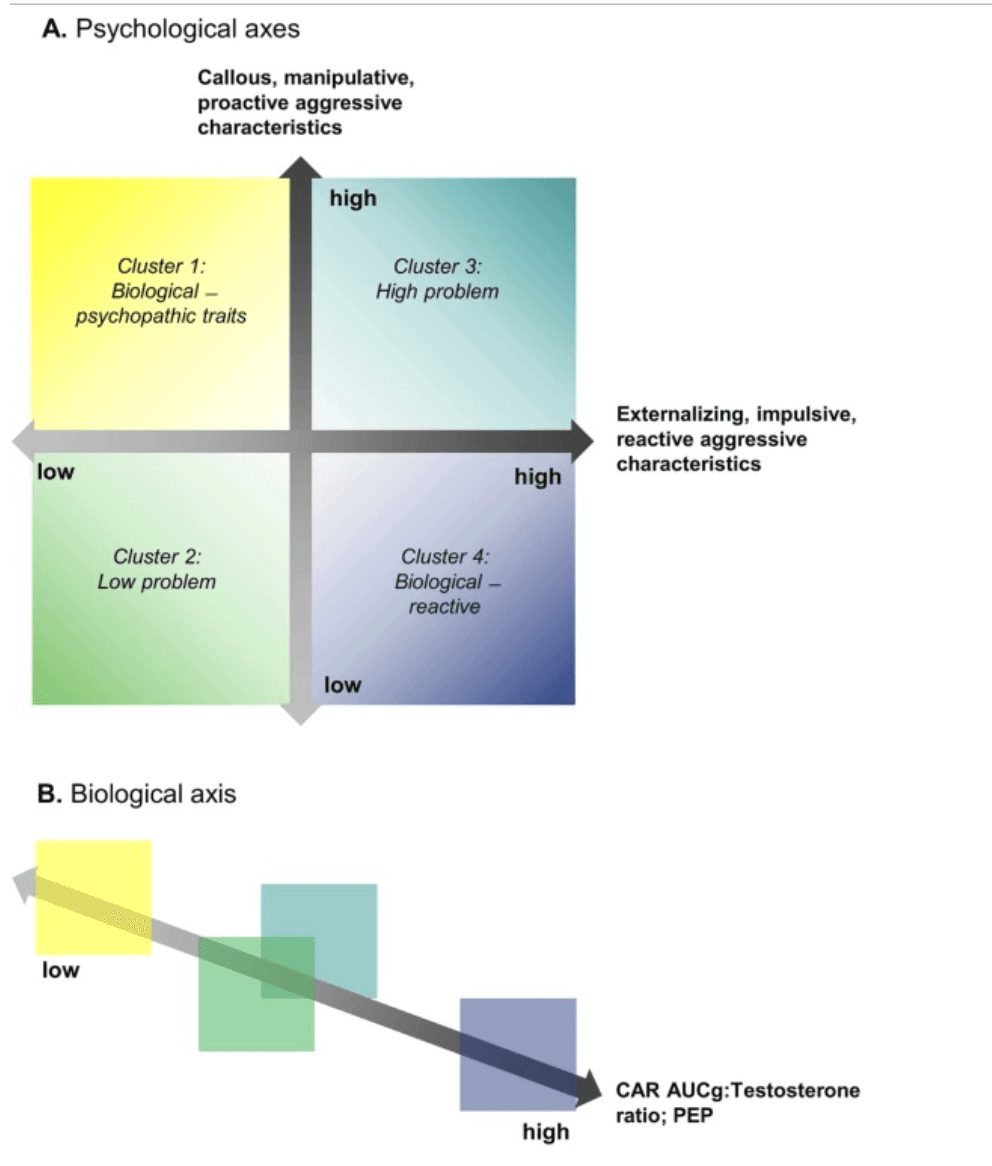
Fig. 2. Simplified, schematic representation of the four clusters based on their psychological (A) and biological (B) axes. A. The four clusters are depicted in a quadrant with (roughly considered) two dimensions (for interpretive purposes only), with callous-manipulative, proactive aggressive tendencies on the y-axis (i.e., YPI Interpersonal, YPI affective, low Empathy, RPQ Proactive aggression scales) and externalizing, reactive-aggressive characteristics on the x-axis (YPI Behavioral, RPQ Reactive aggression, Externalizing problems). Each cluster has their own color throughout the results to ease interpretation: yellow (cluster 1: biological – psychopathic traits, green (cluster 2: low problem), turquoise (cluster 3: high problem) and purple (cluster 4: biological – reactive). The shading/gradient within each cluster reflects the heterogeneity (inter-individual differences) within each cluster. B. The clusters on a biological axis (for interpretative purposes only), considering levels of the Cortisol Awakening Response – total volumes, and Testosterone (here indicated as a CAR AUCg↑:Testosterone↓ ratio), and pre-ejection period (PEP).
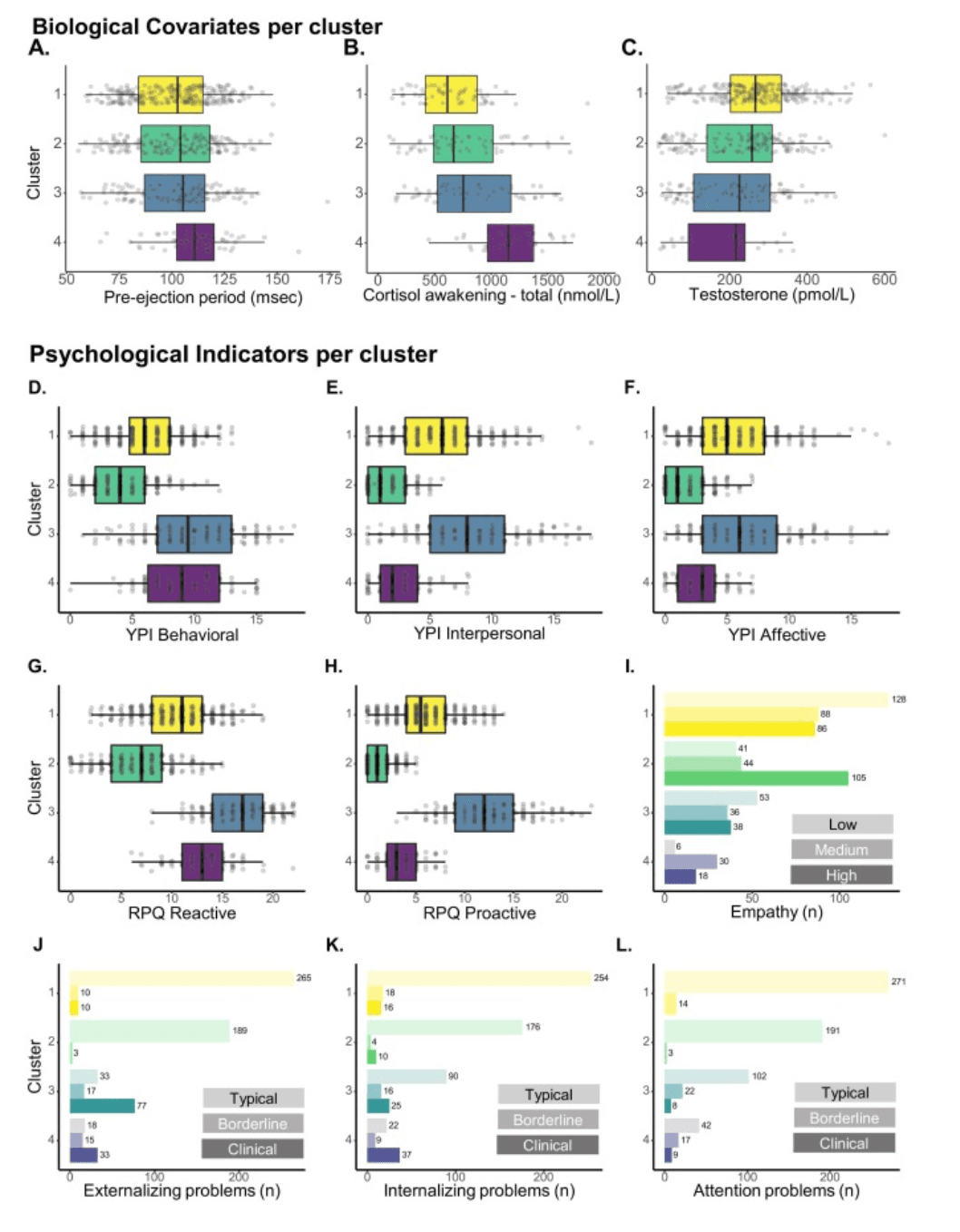
Fig. 3. Raw available data of the significant biological covariates (A-C) and the significant psychological indicators (D-L) per cluster, with box plots superimposed (for continuous data). For the biological covariates only significant variables are shown. For all biological plots, included non-significant variables, see Fig. S2. Categorical variables are shown in number of participants per category.
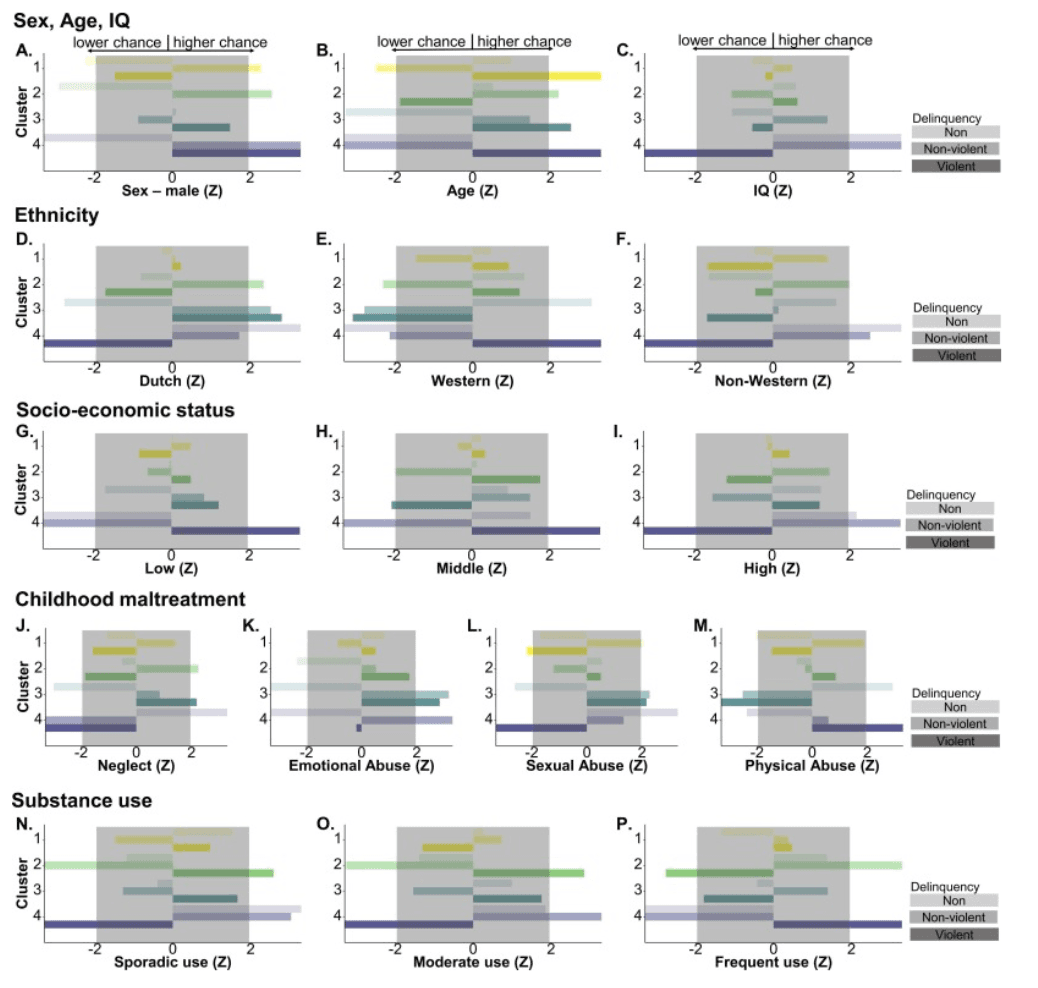
Fig. 4. Effects of the social and environmental variables on the chance of non-delinquency (light-shaded colors), non-violent delinquency (medium-shaded colors), and violent delinquency (dark-shaded colors), per cluster (cluster 1: yellow, cluster 2: green, cluster 3: turquoise, cluster 4: purple). When value exceed the shaded sections, this indicates a significant effect. Values within the shaded sections thus represent non-significant effects. The bars represent Z-scores. As such, values lower than −1.96 (lower chance) or higher than +1.96 (higher chance) indicate a significant effect.
Cluster 1: Biological – psychopathic traits group. This cluster, with a class size of.40, indicating that in our population 40 % of the people would be assigned to this cluster, was characterized by above average YPI Interpersonal scores and YPI Affective scores, below average YPI behavioral scores, just above average proactive aggression scores, just below average reactive aggression scores, a relative low empathy level, and typical Externalizing, Internalizing, and Attention scores. Because of this clusters’ relatively higher levels of testosterone, and lower CAR AUCg (total levels of cortisol during awakening, we added the biological label to this group.
Cluster 2: Low problem group. Cluster 2, class size.27, showed low YPI scores, low Proactive and Reactive aggression scores, high empathy scores, and typical Externalizing, Internalizing and Attention scores.
Cluster 3: High problem group. Cluster 3, class size.21, showed high scores on all YPI scales (Interpersonal, Affective, Behavioral), high Proactive and Reactive aggression scores, had the highest probability of low empathy scores, showed clinical Externalizing scores, borderline and clinical Internalizing scores, and typical Attention scores.
Cluster 4: Biological – reactive group. Cluster 4, class size.12, showed mostly medium to high empathy scores, below average YPI interpersonal and affective scores and high behavioral scores, clinical Externalizing and Internalizing scores, mostly typical Attention scores, below average Proactive and above average Reactive aggression scores. This cluster includes ‘biological’ its label due to their below average testosterone and above average PEP and CAR (total volume).
In the supplements we show how the different samples – which varied in severity of antisocial background – were differentially represented across clusters (Figure S1). The probability of participants being in the assigned cluster was high (cluster membership probability: cluster 1: 84 %; cluster 2: 86 %; cluster 3: 89 %, cluster 4: 85 %, see also Table S7). Figures S2 and S3 shows raw data of all biological and all social-environmental variables (respectively) for each cluster, with box plots superimposed (where applicable).
3.2. Predicting delinquency by the classes and the social-environmental variables within the latent classes
The probability of violent delinquency was highest in clusters 1 (biological – psychopathic traits) and 3 (high problem) and lowest in cluster 2 (low problem group), while the probability of non-violent delinquency was highest in cluster 2. Specifically, probabilities of non-delinquency were 0.14 (cluster 1), 0.23 (cluster 2), 0.18 (cluster 3), 0.21 (cluster 4). Probabilities of non-violent delinquency were 0.17 (cluster 1), 0.31 (cluster 2), 0.13 (cluster 3), 0.20 (cluster 4). The probabilities of violent delinquency were 0.69 (cluster 1), 0.47 (cluster 2), 0.66 (cluster 3), and 0.59 (cluster 4; see Table S8 for an overview).
Importantly our main interest was in how the clusters related to delinquency probabilities moderated by the social and environmental measures. The results show that the latent class variable and all background variables within the classes contribute significantly to predicting delinquency. In short, the delinquency risk for cluster 1 (biological – high psychopathic traits) was barely impacted by social and environmental variables (only age, sex, and sexual abuse). In cluster 2 (low problem), substance use played a particular prominent role in predicting delinquency, while in cluster 3 (high problem – high psychopathic traits group), particularly childhood maltreatment variables played a significant role. Finally, in cluster 4 (reactive group) all social and environmental variables impacted delinquency. Below we describe these associations per cluster in further detail (and see Appendix G for a full description). Table S9 shows all parameter estimates for the regression model predicting delinquency, for all clusters and all delinquency categories, and Table S10 shows Wald statistics. Fig. 4 visualizes, with Z-scores, how the social variables impact the relation between cluster membership and the probability of the three delinquency categories.
In cluster 1, the biological - psychopathic traits group only age, sex, and physical and sexual abuse impact the risk of delinquency. Specifically, the chance of non-delinquency is lower for males and for those who have experienced Physical abuse. Furthermore, the chance of non-violent delinquency is higher for males, and for those individuals who have experienced Sexual abuse, while the chance of non-violent delinquency is lower for older individuals. In contrast, the chance of violent delinquency is higher for older individuals, and lower for individuals who have experienced Sexual abuse.
In cluster 2, the low problem group Sex, Age, Ethnicity, Neglect, Emotional abuse, and in particular, Substance use, play a significant role. Specifically, the chance of non-delinquency is lower for males and for those who have experienced Emotional abuse. The chance of non-violent delinquency is higher for males, older individuals, individuals of Dutch descent, individuals who experienced Neglect, and Frequent substance users, while this chance is lower for individuals from Western descent, and for Sporadic and Moderate substance users. In contrast, the chance of violent delinquency is higher for Sporadic and Moderate substance users, but lower for Frequent substance users.
In cluster 3, the high problem group Age, Ethnicity, SES, and particularly childhood maltreatment significantly impact the chances of delinquency categories. Specifically, the chance of non-delinquency is higher for individuals from Western descent and for those who have experienced Physical abuse, while this chance is lower for older individuals, individuals from Dutch descent, and those who have experienced Neglect, Sexual abuse, and Emotional abuse. Second, the chance of non-violent delinquency is higher for individuals from Dutch descent, for those who have experience Sexual abuse, and for those who have experienced Emotional abuse. In contrast, the chance of non-violent delinquency is lower for those from Western descent, and for those who have experienced physical abuse. Furthermore, the chance of violent delinquency is higher for older individuals, individuals from Dutch descent, and individuals who have experienced Neglect, Sexual abuse, and Emotional abuse. Finally, the chance of violent delinquency is lower for individuals from Western descent, for individuals from middle SES, and individuals who have experienced physical abuse.
Finally, in cluster 4, the biological – reactive group, all social and environmental variables significantly impact delinquency probabilities. First, the chance of non-delinquency is higher for individuals from Dutch and Non-Western descent, individuals from high SES, higher IQ, those who have experienced Neglect and Sexual Abuse, and for Sporadic substance users. In contrast, the chance of non-delinquency is lower for males, older individuals, those from low SES, individual who have experienced Physical and Emotional abuse, and for Frequent substance users. Furthermore, the chance of non-violent delinquency is higher for males, individuals from a high SES, individuals with a higher estimated IQ, those who have experienced Neglect and Emotional abuse, and Sporadic and Moderate substance users. The chance of non-violent delinquency is lower for older individuals, individuals from low and middle SES, individuals from Western and Non-Western descent, and Frequent substance users. The chance of violent delinquency is higher for males, older individuals, individuals from low and middle SES, individuals from Western and Non-Western descent, individuals who have experienced Neglect and Physical abuse, and Frequent substance users. The chance of violent delinquency is lower for individuals from high SES, individuals from Dutch descent, those with higher IQ, those who have experienced Sexual abuse, and Sporadic and Moderate substance users.
4. Discussion
This study applied latent-class regression analysis to explain non, non-violent, and violent delinquency in a heterogeneous group of at-risk youth (N = 876). Following the biopsychosocial model, we defined biological clusters of at-risk youth, incorporating psychological measures, and examined how these clusters differed in non-violent and violent delinquency risk moderated by social and environmental variables. We observed four distinct clusters of youth, each with their unique profile: a biological – psychopathic traits group, a low problem group, a high problem group, and a biological – reactive group. These clusters differed in their probability of non, non-violent, and violent delinquency, but importantly, these probabilities depended on social and environmental measures. Below we describe how these cluster-specific findings relate to relevant theories regarding risk factors for delinquent behavior, and how the current biopsychosocial approach may aid in explaining these findings in a comprehensive manner. We end the discussion with implications for clinical and forensic practice.
In concordance with the theory of Blair (2013) and resonating with primary versus secondary psychopathy distinctions (Fowles, 1980, Lykken, 1995) we found both a psychopathic/proactive aggressive and an impulsive/reactive aggressive group with largely opposing biological profiles. It has been proposed that specific aspects of antisocial behavior such as psychopathic traits in youth can be explained by a reduced empathic response to distress in others (Blair, 2013). In the current sample this was represented in our biological-psychopathic traits cluster. However, as proposed, other youths show high levels of externalizing and internalizing behavior, impulsivity, and high reactive aggression (Blair, 2013), a pattern we observed in our biological-reactive group. These opposing psychological profiles were paralleled by differences in biological stress attunement, specifically, in the total level of cortisol secretion during awakening, and in testosterone levels. Whereas the biological-psychopathic traits group was characterized by particular low total levels of cortisol during awakening, the biological-reactive group showed high total levels of cortisol during awakening. This coincides with biological arousal theories reflecting under-arousal and over-arousal, respectively (for review, see van Hazebroek et al., 2019) (Blair, 2013, Raine and Liu, 1998, Zuckerman, 1990, Zuckerman and Riskind, 2000) (Blair, 2013, Fanti, 2018, Fanti et al., 2019, Scarpa and Raine, 1997). However, we also observed longer PEPs in the reactive group (indicating lower sympathetic arousal) which does not fit the cortisol awakening finding within this group (indicating heightened arousal). Future research should therefore confirm these results and examine to what extent biological under- and over-arousal is specific to different clusters of youth. Finally, we observed high levels of testosterone in the biological-psychopathic traits group, and low levels of testosterone in the biological-reactive group. The high levels of testosterone combined with low levels of CAR observed in the psychopathic traits group matches well with prior work on testosterone as a key marker of status and dominance-related behaviors, in particular in combination with low levels of cortisol, also known as the dual-hormone hypothesis (Archer, 2006, Carré and Archer, 2018, Dabbs et al., 1991, Dekkers, 2018, Geniole et al., 2019, Mehta and Josephs, 2010, Rowe et al., 2004, Terburg et al., 2009).
It is important to note that biology only partly explains antisocial behavior, and that social and environmental measures impact the relation between biology and psychology contributing to antisocial behavior (for review, see Belsky et al., 2007; Blankenstein et al., 2022; Jansen, 2022; McCrory et al., 2022; Susman, 2006; van Hazebroek et al., 2019; Zuckerman and Riskind, 2000). The biological-psychopathic traits group showed the highest probability of violent delinquency, but delinquency probabilities were influenced by hardly any of the social and environmental variables. Conversely, although the biological-reactive group showed a similar high risk of violent delinquency, all social and environmental measures appeared to have an accumulated impact on the risk of non-violent and violent delinquency. For instance, the probability of violent delinquency was increased by a multitude of variables, including low or middle SES, a (non-Dutch) Western ethnicity, lower IQ, physical abuse, and frequent substance use. In this group, the probability of non-violent delinquency was increased for high SES, a higher IQ, non-Western ethnicity, emotional abuse, and sporadic and moderate substance users. Although not directly tested, these cluster-specific findings may partially be interpreted in light of diathesis stress/dual-risk model, which state that individuals with a biological sensitivity are high at risk of developing antisocial behavior when exposed to certain adverse social and environmental contexts (Monroe and Simons, 1991). These findings can also be interpreted in an extension of this model, the differential susceptibility model (Belsky et al., 2007, Boyce and Ellis, 2005, Ellis et al., 2011). This model suggests that biological vulnerabilities sensitize individuals towards both negative and positive social environmental contexts. The differential susceptibility model proposes that when exposed to positive life experiences, individuals with a biological sensitivity have a chance of more positive life outcomes than their non-sensitive peers. If so, this would make the reactive group in the current sample particularly malleable for changes in social and environmental circumstances. Future research may examine whether this malleability exists and whether this provides a window of opportunity for youths characterized by reactivity and biological sensitivity, for instance with regard to treatment selection.
Importantly, we did not find striking biological differences in the low problem group (except for a lower total CAR volume) and the high problem group. This shows that biology may not play a significant role in all types of youth. The low problem group was characterized by high levels of empathy, and low overall levels of psychopathic traits and reactivity, and had the lowest probability of violent delinquency and the highest probability of non-violent delinquency. In this group we observed that frequent substance use in particular increased the probability of non-violent delinquency. This group, characterized by few risk factors, aligns with theories suggesting that for some youth, delinquency is limited to adolescence and is part of a normative development characterized by boundary-seeking and rule-breaking behavior (Moffitt, 2017). Tentatively, the low problem group reflects such a normative adolescent group which is not as affected by psychological or biological factors, and may be prone to delinquency under the influence of substances. Nonetheless, future studies should further test this.
Conversely, the high problem group was most affected in their psychological functioning. This group was characterized by both high levels of psychopathic traits and high levels of reactivity, and this group showed a high probability of violent delinquency, comparable to the biological-psychopathic traits group. In this group, having experienced sexual and emotional abuse increased the probability of non-violent and violent offending, while neglect increased the probability of violent offending only. This echoes prior work showing that experienced childhood maltreatment increases the risk of delinquency in adolescence and adulthood (Maas et al., 2008, Maschi, 2006, Mersky et al., 2012, Savage et al., 2014). Given that biological factors nor other social and environmental measures played a prominent role, the findings in the high problem group suggest that psychopathology and childhood maltreatment may override other potential risk factors.
Together, several influential theories described above are tied together under the biopsychosocial model by combining biological, psychological, and social and environmental factors and creating the clusters using a data-driven approach. Moreover, we were able to better explain the heterogeneity of delinquent behavior, by identifying specific clusters with different profiles of risk factors. Thus, we suggest that the biopsychosocial model may function as an overarching model which converges insights from previous influential theories. Future research integrating biopsychosocial factors in even larger samples may further confirm this hypothesis.
4.1. Implications
The biopsychosocial framework has already been widely adopted as a useful concept in practice. Prior work showed that while psychosocial risk factors provided moderate predictive validity for recidivism in delinquent youth, including protective factors and biological functioning (specifically, heart rate variability) improved prediction models (de Ruigh et al., 2020). Developmental crime prevention programs that consider neurobiological, psychological, and social factors together have been shown to reduce crime better than programs that do not include neurobiological factors (de Kogel and Alberda, 2018, Rocque et al., 2012). Moreover, resting/basal measures as proxies of the autonomic nervous system and the neuroendocrinological system, can be easily assessed in clinical and forensic practice. In addition, our cluster-specific findings raise insights for prevention and intervention programs aimed to reduce crime in youth, depending on their biopsychosocial ‘profiles’. This allows to differentiate between specific subtypes of youth depending on their vulnerabilities, which have implications for practice. Below we provide suggestions for how these biopsychosocial profiles may translate to intervention and prevention decision-making in practice. It is important to note that the current findings should be corroborated and finetuned with longitudinal studies, including samples that generalize beyond the participant group of the current study, of whom the behavior was identified and recognized by authorities. This makes the suggestions below tentative and a starting point for future studies.
In the first cluster hardly any of the social and environmental variables – as included in the current study – impacted the chance of the different delinquency categories. This suggest these individual biological and psychological characteristics as observed in this cluster may show a direct link with non-violent and violent delinquency and may be less malleable by social and environmental factors. In this particular cluster intervening in social and environmental context may be less effective, while psychiatric intervention may be more appropriate. Second, in the low problem group non-violent and violent delinquency risk was impacted most pronounced by substance use. For youths characterized by this profile, prevention and intervention programs aimed at substance use may be most effective. Third, the high problem group was characterized by profound psychological problems which may be best tackled with adequate psychological treatment (e.g., behavioral therapy). Moreover, the most pronounced impact on the delinquency probabilities for individuals in this cluster were childhood maltreatment variables. For youth characterized by this high problem profile, a heightened role for trauma treatment may be recommended. Finally, in the biological–reactive group all social and environmental factors impacted the risk of (non-)violent delinquency. This combination of adverse social and environmental contextual factors may put these individuals particularly at risk for antisocial behavior. At the same time, in line with the differential susceptibility model, these youths may particularly benefit from prevention and intervention programs targeted to improve social and environmental circumstances, which provides a window of opportunity to steer these youths towards positive life outcomes.
Finally, it should be stressed that although each cluster was characterized by their unique profiles, within each cluster there was considerable heterogeneity, as illustrated by our raw data plots. This heterogeneity is important to consider in clinical and forensic practice. Although treatment may be adapted towards biopsychosocial profiles, treatment should ideally be tuned towards individual strengths and weaknesses. Future research may further examine how we move from a subgroup-based approach to an even more individualized approach and highly personalized risk taxation and treatment selection, for instance using single-case experimental designs.
4.2. Strengths and limitations
This study has a number of strengths such as a large, heterogeneous sample size across a broad age range (9–27 years, although the majority was at least 12 years old), and a comprehensive biopsychosocial approach. To attain a large sample size we combined data from five independent study samples. A strength of this approach is that these samples differed in their antisocial background, resulting in a heterogeneous group of participants. By harmonizing data between samples, we were able to combine a large number of participants despite different assessment strategies for the same construct. However, a drawback of this approach was that for some constructs this resulted in crude categorical measures, specifically for childhood maltreatment variables (which included experienced maltreatment only and not observed maltreatment), substance use, and delinquency. Preferably a study would include more sensitive, continuous, data on these variables. Despite these drawbacks this approach allowed to include a large number of variables: the resulting combined dataset included nine resting biological (ANS and neuroendocrinological) measures, nine psychological measures, and seven social and environmental measures. This enabled us to test the biopsychosocial model from a broad perspective.
Second, to the best of our ability we have organized measures under either biological factors, psychological factors, or social and environmental factors. This division is however artificial and we acknowledge that other divisions may also possible and meaningful. It is important to realize that biological, psychological, and social and environmental measures factors are interrelated and can impact each other, which we applied in our current LCRA approach. Relatedly, we compared models using fit indices to decide the numbers of clusters. Model selection is often delicate and often does not lead to a clear unambiguous solution (Sinha et al., 2021), which was the case here. Nonetheless, we believe that the chosen four-cluster solution best balanced model complexity and interpretability.
Third, overall our sample was imbalanced regarding sex, including more males (n = 715) than females (n = 161). This is unsurprising given the relative overrepresentation of males in overt delinquent behavior (e.g., see Fontaine et al., 2009). Cluster-specifically we found that whereas in the low–problem, high–problem, and biological – psychopathic traits groups males were over-represented, sex was more evenly distributed in the biological – reactive group. This provides an indication for differences in biopsychosocial profiles for boys and girls, which future research including more female participants may further unravel. For the current research the imbalance in sexes should be acknowledged when interpreting the results, especially with regards to associations between biological measures and antisocial behavior of which knowledge on sex-specific associations is limited (Freitag et al., 2018).Finally, although we included a measure of affective empathy, we could not assess cognitive empathy (social perspective taking) because this measure was unavailable in the majority of samples. Relatedly, we did not have information on the role of the social networks of adolescents, such as neighborhood density, caregivers, peers, teachers, and additional significant others in adolescents’ delinquency risk (Bronfenbrenner, 2000). In addition, future studies may also include measures of impulse control, sensation-seeking, and future orientation (Zuckerman, 1990, 2000). To capture these additional psychological and social environmental measures would result in an even broader biopsychosocial perspective. A novel question would be to test not only how these factors increase vulnerability towards an antisocial developmental pathway, but also which factors steer adolescents towards positive life outcomes. This aligns well with the increased consideration of protective factors rather than focusing solely on risk factors (de Ruigh et al., 2020, Goodwin et al., 2022, Kleeven et al., 2022).
5. Conclusions
This data-driven study applied the biopsychosocial model to test how psychological-driven biological clusters of at-risk youth differed in their non-violent and violent delinquency risk. Four clusters of youth were observed, each with their unique profiles and vulnerabilities to non-violent and violent delinquency: a biological – psychopathic traits, low problem, high problem, and biological – reactive group. Importantly, vulnerabilities to non-violent and violent delinquency depended on social and environmental context. The current data-driven results provide important insights into each clusters’ unique windows of opportunity for assessment and treatment selection and outcome. Together, we show how the biopsychosocial model converges insights from prior models and hence explains the risk of non-violent and violent delinquency in a heterogeneous population of youth. These insights may ultimately optimize intervention in antisocial youth based on individual factors.