Abstract
Perceptions of crime detection risk (e.g., risk of arrest) play an integral role in the criminal decision-making process. Yet, the sources of variation in those perceptions are not well understood. Do individuals respond to changes in legal policy or is perception of detection risk shaped like other perceptions—by experience, heuristics, and with biases? We applied a developmental perspective to study self-reported perception of detection risk. We test four hypotheses against data from the Dunedin Longitudinal Study (analytic sample of N = 985 New Zealanders), a study that spans 20 years of development (Ages 18–38, years 1990–2011). We reach four conclusions: (1) people form their perception of detection risk early in the life course; (2) perception of detection risk may be general rather than unique to each crime type; (3) population-level perceptions are stable between adolescence and adulthood; but (4) people update their perceptions when their life circumstances change. The importance of these findings for future theoretical and policy work is considered.
Experience teaches us that threatening someone with punishment is an effective way to influence their behavior. The idea is simple: Convince the other party the risk of detection is high enough to deter the unwanted behavior. As such, children refrain from reaching into the cookie jar when adults are watching and traffic slows on the motorway when a police car is present. It works at a macrolevel too—politicians use rhetoric like “getting tough on crime,” police set quotas for “offences brought to justice” (Sosa, 2012), and global leaders threaten “mutual assured destruction” in an attempt to deter others from untoward acts.
Accordingly, deterrence and rational choice theories have held a central position in studies of criminal decision making for more than 200 years (Apel & Nagin, 2017; Beccaria, 1764/1963; Wilson & Petersilia, 2010). Those theories operate under two main assumptions: (a) People make rational decisions by calculating the risks and benefits of their behaviors, and (b) it is possible to deter undesired behavior by making punishment (i.e., the risk) more certain, swift, or severe. Scholars have found support for many of the propositions stemming from these theories (Loughran et al., 2016; Piliavin et al., 1986; Pratt et al., 2006), which has encouraged policymakers to draw on the principles of deterrence to more effectively deploy criminal justice resources (Nagin et al., 2015).
Despite the amount of supportive evidence, a gap remains in our understanding of how deterrence “works” at an individual level. In theory, the mechanisms are clear: (a) the objective risk of being detected during or after the commission of a crime affects individuallevel perception of that risk; and (b) that perception of detection risk impacts the criminal decision-making process.
Empirical work supports the second proposition: A person’s perception of detection risk affects their willingness to commit a crime (see Apel, 2013, 2022, for a review). This evidence has inspired a new line of theory in criminology that delineates the factors that may deter individuals from specific crimes and those that might prevent crime at a population-level (Jacobs, 2010). A previous analysis of the Dunedin Longitudinal Study (the same data we employed here) reported an association between perception of detection risk and later involvement in criminal activity (Wright et al., 2004). It was shown that people who perceived higher detection risk at Age 18 reported lower involvement in crime 8 years later at Age 26. This association persisted even after adjusting for two of the strongest predictors of criminal behavior, socioeconomic status and self-control.
The gap in the knowledge base emerges when we consider the first proposition: Is perception of detection risk associated with objective detection risks? It seems obvious that these two variables would be strongly correlated, but a recent assessment of the literature found only limited support for that relationship (Pickett & Roche, 2016). A growing body of the literature identifies situations where a correlation between objective risks and perception of those risks would be expected but, when analyzed, there is none. For example, prior work failed to find a substantive relationship between objective measures of arrest risk and individuals’ perceptions of those risks (Kleck & Barnes, 2014; Kleck et al., 2005). Other studies reported statistically significant but substantively small associations between objective punishment risks and children’s perception of those risks in American schools (Apel et al., 2009; Nixon & Barnes, 2019).
What might explain these unexpected results? It could be the general public is simply not well informed about the prevailing punishment risks for any given criminal offense. Consider, for example, the ramifications for failing to appear in court. A recent study found that many defendants failed to appear in court because they did not realize they would face punishment for skipping (Fishbane et al., 2020).
Against this backdrop, we drew on work from the psychological study of risk perception to gain insight into the factors that affect perception of crime detection risk. For the purposes of this study, we define perception of crime detection risk as: the perceived likelihood of being caught during or after the commission of a crime. We applied a developmental lens to this body of work and derived four hypotheses. We then tested our hypotheses against observations from a representative and prospective longitudinal birth cohort of New Zealanders. We collected repeated measures of perception of detection risk over a 20-year span (from Age 18 to Age 38), allowing for the assessment of (in)stability in perception over the adolescence-to-adulthood transition; a time when criminal participation peaks and the desistance process begins.
This study advances the literature because research into criminal decision making typically relies on cross-sectional data or shortterm longitudinal data (e.g., Matsueda et al., 2006; Piquero et al., 2012). Additionally, the broader decision-making literature often focuses on decisions that are not as risky as getting arrested and incarcerated, with its subsequent potential to ruin one’s life opportunities. With this perspective, we submit that the study of crime decisions represents a kind of acid test for decision-making theories. Criminal decisions are high stakes, but objective risk information is ambiguous (Loughran et al., 2011) and emotions are running hot (van Gelder & De Vries, 2012). Rational decisions are unlikely to emerge in situations like that, opening the possibility that mechanisms outside of the deterrence/rational choice paradigm will be influential.
In the sections that follow, we review the literature that speaks to the developmental mechanisms that could play a role in the formation of perception of detection risk. As will be shown, relatively little attention has been paid to the factors that impact individual-level perception of detection risk. Moreover, few studies have attempted to discern which factors contribute to changes in perception of detection risk over the life course.
Research on Perception of Detection Risk
Although relatively little attention has been paid to the factors that influence variation in perception of detection risk, there is a small body of experimental and observational work that is informative. Terpstra et al. (2020) worked with police in the Netherlands to experimentally manipulate the rate of police traffic stops for moped riders. Police checkpoints are a common occurrence in the Netherlands, but during treatment periods the checkpoints were set up more frequently and moped riders were targeted to be stopped and checked for proper documentation (license, insurance). Findings indicated that perception of punishment risk was impacted by the intervention, suggesting formal interventions from government institutions can influence population-level perceptions of detection risk.
Supportive evidence also comes from observational studies that assess changes in perception of risk as a function of contact with the justice system—what criminologists call the “experiential effect.” This research shows that people tend to increase their perception of detection risk after they experience an arrest (Anwar & Loughran, 2011; Pogarsky et al., 2004). One study drew on data from two general population samples—the National Longitudinal Survey of Youth 1997 Cohort and the National Youth Survey—and concluded that “successful” crimes (i.e., evaded detection) led to reductions in perception of arrest risk while “unsuccessful” crimes (i.e., resulting in arrest) increased perception of arrest risk (Lochner, 2007). In other words, getting caught seems to serve one of its intended purposes: It makes people believe they are more likely to be caught in the future.
Collectively, this body of evidence reveals that perception of detection risk may be linked to various individual-level traits and experiences. Prior work has even shown that personality traits impact the criminal decision-making process (van Gelder & De Vries, 2012). Yet, few studies have analyzed the development of perception of detection risk over an extended period of time. Most of the available literature draws on either cross-sectional data or shortterm longitudinal data (e.g., Matsueda et al., 2006; Piquero et al., 2012). Thus, questions remain about how people develop their risk perception early in the life course and, once formed, how that perception changes as they engage in and ultimately desist from criminal activity. These observations inspired us to consider whether perception of detection risk is best understood through the lens of a developmental perspective.
Perception of Detection Risk: A Developmental Perspective
The ideas and principles that underpin modern deterrence and rational choice theories have been established since the Enlightenment (Beccaria, 1764/1963; Bentham, 1789/1988). Contemporary scholars expanded the scope of these theories by integrating insights from studies that reveal how people form perceptions about their world (Pickett et al., 2018; Pogarsky et al., 2018), how those perceptions affect the behavior of would-be offenders (Jacobs, 2010), and how this information could be used to inform policymakers (Nagin et al., 2015).
In keeping with this history of integration, we propose that perception of detection risk should be viewed like a trait that forms early in the life course and is subject to changes that occur during development. In this sense, we suggest that perception of detection risk is similar to other personal traits; it is relatively stable over time but it is sensitive to our environments.
How Does Perception of Detection Risk Form—Specifically or Globally?
We propose that perception of detection risk is a manifestation of a higher order factor. For example, perception of detection risk may be one component of a larger trait such as risk aversion (see generally, Frey et al., 2017). Under this model, people do not hold unique perceptions of risk for different criminal acts. Rather, people have a general perception of risk that drives their perceptions about the risks inherent in specific situations. This model allows for the possibility that risk perception will be influenced by other sources of information, such as affect (Johnson & Tversky, 1983; Slovic & Peters, 2006) and circumstances specific to a situation (Jacobs, 2010). In other words, we propose that perception of detection risk is a construct that falls somewhere in between a “pure trait” and a “pure state” (see generally, Anusic et al., 2012).
When it comes to estimating risk, research indicates a person’s affect may play a role. Using three independent survey studies, Pickett and Bushway (2015) assessed whether cognitive heuristics and dispositional attributes (e.g., positive and negative affect) predicted perception of arrest risk. There was no support for a link between negative affect and perception of arrest risk. But support was found for a link between positive affect and perceptions, such that individuals who had more positive affect tended to perceive a higher risk of arrest when a crime is committed. If affect drives risk perception, then it might be the case that people hold a general perception of detection risk rather than unique perceptions for different crimes.
Supportive evidence also comes from survey research showing that people tend to rate the level of risk across multiple domains (economic, health, and victimization) in a consistent manner (Dominitz & Manski, 1996). When one perceives a high risk of losing a job or becoming ill, she is also likely to perceive a high risk of being victimized in a crime event. If a person thinks the risk of punishment for crime A is high, he is also likely to report the risk of punishment for crime B is high (Pickett & Bushway, 2015).
Translating these findings to the present focus, we propose the following hypothesis.
The global factor hypothesis: Perceptions of detection risk are correlated across different crimes, such that there is a global perception of detection risk that represents each person’s views of the risk of detection for various criminal acts.
Is Perception of Detection Risk Age-Graded?
It fits theoretical logic to predict an age-graded increase in perception of detection risk during the adolescence-to-adulthood transition (e.g., Gartner & Piliavin, 1988; Sampson & Laub, 2003; Shover & Thompson, 1992). Insight can be drawn from the agecrime curve, which is characterized by a sharp increase in crime and delinquency in adolescence that peaks in the late teenage years and then follows a steady decline through middle adulthood (Farrington, 1986; Moffitt, 1993). Theoretical explanation of this phenomenon often points to the social connections, relationships, and major life events that come together in early adulthood (Giordano et al., 2002). These connections and relationships are risked whenever a crime is committed, which could indirectly impact perception of detection risk.
Thus, it is anticipated that perception of detection risk will: (a) reach its nadir prior to the peak-crime age of late-adolescence/earlyadulthood and (b) rise through adulthood. There is some tangentially supportive evidence to support this hypothesis. Hjalmarsson (2009) identified an increase in the perception of punishment risk when youth hit the “age of criminal majority,” which is the age at which a person can be tried in adult court. This suggests youth—particularly those who have had contact with the criminal justice system—may be aware of the increase in objective punishment risks that come with aging into adulthood. Similarly, a recent study found that perception of arrest risk increased by about 15% over a 7-year span that covered adolescence and early adulthood (Thomas & Vogel, 2019).
Although the age-graded nature of perception of detection risk has been assessed in a small group of studies (Lochner, 2007; Pogarsky et al., 2004), that work has relied on short follow-up periods or relatively small sample sizes. Our study will contribute to this area by analyzing longitudinal data that covers 20 years of development for nearly 1,000 participants. We will test the following hypothesis:
The age-graded hypothesis: Perception of detection risk is age-graded—it increases from adolescence to adulthood.
What Accounts for Changes in Perception of Detection Risk From Adolescence to Adulthood?
In an effort to understand why humans sometimes behave irrationally (Ariely, 2008; Thaler, 2014), scholars have identified many quirks about human perception formation. One finding has consistently emerged: people do not think probabilistically and, therefore, do not tend to make judgments based on the actual probability of an outcome. Instead, human decision-making relies on heuristics, or mental shortcuts, to estimate probabilities of life’s uncertain events (Tversky & Kahneman, 1974).
Attribute substitution is one heuristic that could affect perception of detection risk. Kahneman and Frederick (2002, p. 53) described attribute substitution: “ … when the individual assesses a specified target attribute of a judgment object by substituting another property of that object—the heuristic attribute—which comes more readily to mind” (emphasis in original). When asked about the detection risks of crime (an unknown), we anticipate individuals will substitute something easier to envision: the costs associated with crime detection. Those costs are the social bonds that accumulate during the adolescence-to-adulthood transition (see generally, Bleidorn et al., 2018).
Perception of the costs of crime should increase as the person ages into adulthood, accumulating social bonds (e.g., Sampson & Laub, 1993) and experiencing life events that cause changes to personality traits (Bleidorn et al., 2018). Attention to social bonds makes crime seem costlier, ultimately making detection risk seem higher. Thus, we expect:
The costs-risks hypothesis: People with more social bonds (costs to relationships with friends and family) will perceive greater risks of crime detection.
Finally, criminological research has shown that prior experience with the criminal justice system affects risk perceptions. People set their perception of detection risk based on their own arrest experiences as well as from vicarious experiences through their friends and family (Pogarsky et al., 2005). Getting arrested and convicted likely qualifies as a major life event that could lead to changes in affect or personality traits that ultimately impact perception of detection risk.
The experiential hypothesis: Individuals who experience a conviction will adjust upward their perception of detection risk.
Each of these four hypotheses was tested against observations gleaned from one of the longest-running cohort studies worldwide, the Dunedin Longitudinal Study. As we describe in the next section, the Dunedin Longitudinal Study began following more than 1,000 children who were born in Dunedin, New Zealand in 1972–1973. Since then, participants have been assessed and interviewed 13 more times, with the most recent follow-up occurring when study members had reached Age 45. The present study will draw data from interviews that took place on and between Ages 18 and 38 (covering the years 1990–2011), which is when relevant data were collected. With these data, we can probe the developmental nature of perception of detection risk in a way that no prior study has.
Method
Data
Data were drawn from the Dunedin Multidisciplinary Health and Development Study (Dunedin Longitudinal Study), which is a longitudinal investigation of health and behavior in a birth cohort. Dunedin participants (N = 1,037; 91% of eligible births; 52% male) were all individuals born between April 1972 and March 1973 in Dunedin, New Zealand, who were eligible based on residence in the province and who participated in the first assessment at Age 3. Full details about the sample are reported elsewhere (Poulton et al., 2015, 2023). The cohort represented the full range of socioeconomic status (SES) in the general population of New Zealand’s South Island. On adult health, the cohort matches the New Zealand National Health and Nutrition Surveys on key health indicators (e.g., body mass index, smoking, physical activity, visits to the doctor; Poulton et al., 2015) and the New Zealand Census of citizens of the same age on educational attainment (Richmond-Rakerd et al., 2020).
Assessments were carried out at birth and Ages 3, 5, 7, 9, 11, 13, 15, 18, 21, 26, 32, 38, and most recently, 45 years, when 94% of the living participants took part. Our analysis will capitalize on data drawn from Phases 18 (N = 993, 97% participation), 21 (N = 992, 97% participation), 26 (N = 980, 96% participation), and 38 (N = 961, 95% participation), which are the phases when relevant data were collected. At each assessment phase, participants were brought to the Dunedin research unit for a full day of interviews and examinations. Attrition analyses show no differences between those who remained in the study and the original cohort in regard to childhood IQ, childhood SES, and the Rutter Antisocial Scale (see Supplemental Material A). Data were supplemented by searches of official records and by questionnaires that were mailed, as developmentally appropriate, to parents, teachers, and peers nominated by the participants themselves.
Ethics Statement
The New Zealand Health and Disability Ethics Committee approved each phase of the study, and written informed consent was obtained from all participants.
Transparency and Openness
This study’s analysis plan was preregistered; see at https://sites.du ke.edu/moffittcaspiprojects/projects_2018/. Statistical code for all analyses are available; see at https://sites.duke.edu/moffittcaspiproje cts/projects_2018/. Analyses reported here were checked for reproducibility by an independent data analyst who recreated the code by working from the article and applied it to a fresh copy of the data set. The Dunedin Study data are not publicly available due to lack of informed consent and ethical approval but are available on request by qualified scientists. Requests require a concept article describing the purpose of data access, ethical approval at the applicant’s institution, and provision for secure data access. We offer secure access on the Duke University, Otago University, and King’s College London campuses.
Measures
In this section we report on every measure that will appear in the statistical analysis. To facilitate understanding the structure of our data, Figure 1 presents a timeline of the Dunedin Longitudinal Study, along with phase-indicators for each measure. For example, our focal outcome measure, perceived detection risk was measured at Phases 18, 21, 26, and 38. Descriptive statistics for all measures are available in Supplemental Material B and correlations between all the study variables are presented in Supplemental Material C.
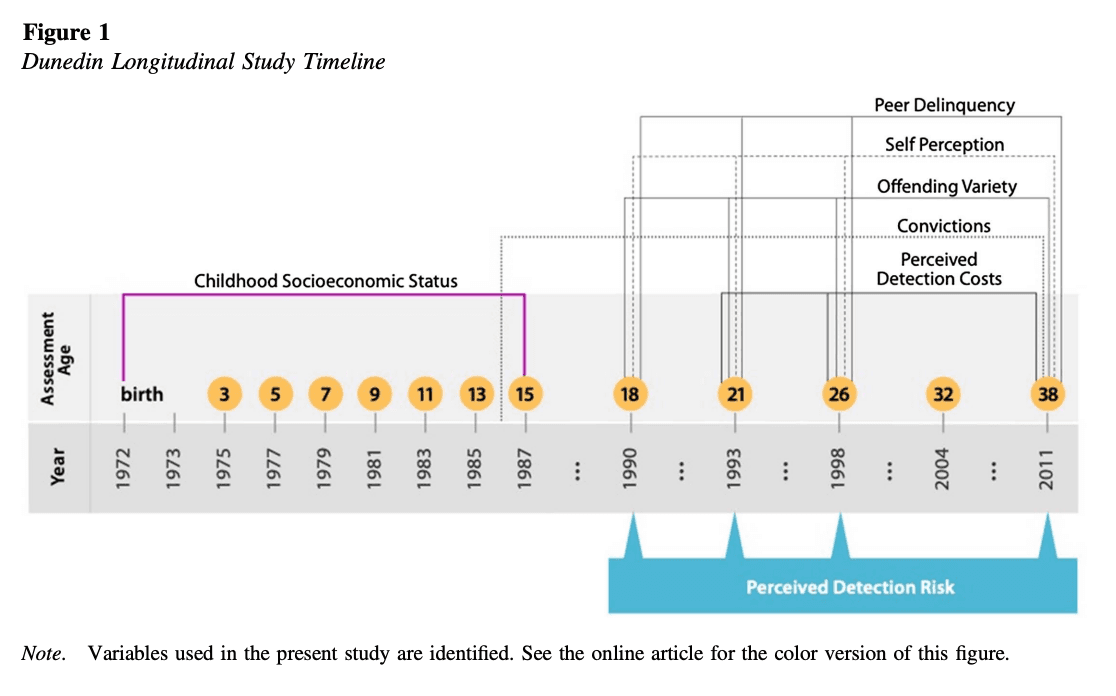
Perceived Detection Risk
During Phases 18, 21, 26, and 38, as part of an interview about illegal behavior, all participants were prompted by the interviewer with the following statement: “Now I will ask you about the chances of getting caught for some activities.” Next, they were presented with the following questions: “If you did {crime} on 10 different days, how many times do you think that you would get caught for {crime}”? Participants were then shown a card with the numbers 0 through 10 on it. They were asked this question with regard to eight focal crime types, including: shoplifting, car theft, burglary, fraud, marijuana use, assault, driving under the influence (DUI), and domestic violence. The focal crime types were not perfectly consistent across all phases, so we first analyzed all available items and then restricted our analysis to the crime types that were asked across all four phases (shoplifting, car theft, burglary, and fraud). More on this below.
Responses were originally recorded on a scale of 0 to 10, but for the analysis we divided the responses by 10, resulting in values that ranged between 0.00 and 1.00. These values can be interpreted as perceived probabilities of detection. Note that the questions were asked in such a way that would maximize participants’ understanding—we did not ask them to report probabilities as percentages, given that research suggests most people do not have a strong intuitive understanding of probability, which might bias responses (Manski, 2004). (Some prior studies have asked “what percent of the time do you think you would be caught for crime X,” but our pilot work showed that many respondents in the community do not understand percent.)1
Perceived Detection Costs From Social Bonds
Two measures of perceived detection costs were included in the analysis: perceived costs from friends and perceived costs from family. During Phases 21, 26, and 38, all respondents were asked the following question: “Would you lose the respect and good opinion of your close friends if they knew you … .” Participants were then given a list of items that included “shoplifted,” “stole a car,” “committed burglary,” “used a stolen bank card,” “used marijuana,” “hit someone else in a fight,” “drove while drunk,” and “beat your partner.” Responses were recorded as no = 0, maybe = 1, or yes = 2. We created the perceived costs from friends measure by summing responses to the above questions so that higher values reflected higher perceived costs from friends. Note the referenced crimes match those from the outcome measure, perceived detection risk. To measure perceived costs from family, we relied on a similar set of questions, but this time the question referenced “parents and relatives.” We combined these items into an index by summing the responses so that higher values reflected higher perceived costs from family.
Time-stable and time-varying versions of both measures were created for the analysis. The time-stable measures were created by grand-mean centering each variable so that participants who scored above the sample average were given positive values and those who scored below the sample average were given negative values. Timevarying versions of both variables (i.e., phase-specific measures) were created by person-mean centering each variable so that values indicated departures from that participant’s average score.
Conviction
Official conviction records were obtained through a search of the central computer system of the New Zealand police, which provides details of all New Zealand and Australian convictions communicated to the New Zealand police. Convictions were dated. Searches for all convictions occurring from the age by which conviction was permissible (14 years) were conducted after each assessment at Ages 21, 26, and 38 years (search completed in 2013).
Two variables were created for the analysis. First, we created a time-stable measure that captured any conviction (no conviction = 0 and at least one conviction = 1). By Age 38, 27% of participants had at least one conviction, matching exactly the rate of conviction in New Zealand’s national police records.
Second, we created a time-varying measure that corresponded to each phase of data collection. Receiving a conviction up to Age 18 was coded as 1 for Phase 18 conviction status, any conviction between Ages 19–21 was coded as a 1 for Phase 21 conviction status, and so on (see Beckley et al., 2018). When coded by phase, 10% of the participants were convicted by Phase 18, 15% received a conviction between Phase 18 and Phase 21, 14% received a conviction between Phase 21 and Phase 26, and 12% received a conviction between Phase 26 and Phase 38.
Offending Variety
During Phases 18, 21, 26, and 38, all respondents were asked whether they had engaged in specific offending behaviors in the past year. Four types of offending were probed. Property offenses included items such as vandalism, breaking and entering, motor vehicle theft, embezzlement, shoplifting, and fraud. Rule offenses included items such as reckless driving, public drunkenness, soliciting or selling sex, giving false information on a loan or job application, and disobeying court orders. Drug-related offenses included using and selling various types of illicit drugs. Violent offenses included items about simple and aggravated assault, gang fighting, robbery, arson, and forced sex. Responses were coded as no = 0 or yes = 1, and then summed to create a variety index of offending in the past year (see Beckley et al., 2018). A time-stable measure of the offending variety variable was created by grand-mean centering and a time-varying measure was created by person-mean centering.
Self-Perception
During Phases 18, 21, 26, and 38, all respondents were asked, “Compared to most people your age, how would you rate yourself on this scale from 0 to 10?” The scale shown to respondents was: “0 = you do a lot fewer illegal things than the average person; 5 = you are about average—like most people on illegal behavior; 10 = you do more illegal things than the average person.” A time-stable measure of the self-perception variable was created by grand-mean centering and a time-varying measure was creating by person-mean centering.
Peer Delinquency
During Phases 18, 21, 26, and 38, all respondents were asked several questions that probed their peers’ level of delinquency: “How many of your friends … .” Respondents were given a list of delinquent behaviors that included “are thought of as good citizens” (reverse coded), “do things that are against the law, such as stealing or vandalism,” “have problems due to the use of alcohol,” “have problems related to the use of marijuana or other drugs,” and “have problems with aggression, such as fighting or controlling anger” (note: the last three items were first asked during Phase 21). Responses were coded using a 5-point Likert scale, with none = 1, half = 3, and all = 5. These responses were averaged within each phase so that peer delinquency represents the average levels of peer delinquency across all behaviors for a given phase. A time-stable measure of the peer delinquency variable was created by grand-mean centering and a time-varying measure was creating by person-mean centering.
Childhood Socioeconomic Status
We used a composite measure of SES that captured the average SES of the Study member’s family of origin from birth to Age 15. This index was created by assessing the parents of the study respondents using the New Zealand Socioeconomic Index and averaging the highest occupational status from either parent since respondents’ birth, as well as 8 subsequent times through their 15th birthdays. Childhood SES was intended to capture the childhood environment and, therefore, was created as a time-stable measure.
Male
Respondent sex was coded female = 0 and male = 1, with females comprising 48% of the sample.
Analysis Plan
The analysis unfolded in four steps. First, we observed agespecific descriptive statistics and line plots as a way to gain insight into the profile of the Dunedin Longitudinal Study participants. Our aim in Step 1 was to assess the perceived detection risk measures and to spot potential trends that may have emerged over the 20-year span of the study.
Second, the phase-specific perceived detection risk items were subjected to correlation analysis, factor analysis, and reliability analysis (i.e., Cronbach’s α and McDonald’s ω). In this step, we tested the global factor hypothesis, which predicted our measures of perceived detection risk hang together on a single latent factor. We tested this hypothesis via confirmatory factor analysis.
The third step to the analysis used perceived detection risk as the outcome measure in a longitudinal analysis. We relied on multilevel modeling to carry out all statistical analyses in this part of the study (Singer & Willett, 2003). The first aim in this step was to estimate the impact of time on within-person changes in perceived detection risk. This provided a direct test of the age-graded hypothesis, which predicted an increase in perception of detection risk from Phase 18 to Phase 38. In order to test the age-graded hypothesis, we subjected our data to multilevel modeling, where the time-specific measures of perceived detection risk were nested within participants. This allowed us to estimate “growth” in perceived detection risk from Phase 18 to Phase 38. We anticipated a positive growth parameter.
The fourth step assessed whether within-person changes (over time) and between-person differences (time stable) in perceived detection risk were associated with changes/differences in the theoretical predictors outlined above. Again, we relied on multilevel modeling to estimate these relationships. As described in the previous section, each measure was coded as both time-stable (via grand-mean centering) and time-varying (via person-mean centering). The two exceptions were childhood SES and male, both of which were only coded as time-stable.
It was in this fourth step to the analysis that we tested the costsrisks hypothesis, which predicted that increases in social bonds (i.e., perceived costs from social bonds) over time will correspond with increases in perceptions of detection risk. We tested this hypothesis by focusing primarily on the time-varying influence of perceived costs from friends and perceived costs from family on perceived detection risk. We anticipated both costs variables will have a positive, time-varying, association with perceived detection risk.
We also tested the experiential hypothesis in step four. Recall this hypothesis predicted an increase in perception of detection risk following a criminal conviction. We tested this hypothesis by assessing the time-varying influence of conviction on perceived detection risk. We anticipated a positive association between the two variables.
Analyses were conducted in R 4.3.1 (R Core Team, 2023) and Stata 17 (StataCorp, 2021). We relied on two-tailed p values of .01 for statistical significance testing. We are also mindful of substantive effect size and, therefore, provide substantive benchmarks as a way to gauge the practical impact of the results (Funder & Ozer, 2019).
Results
Preliminary Analyses
Participants were asked to report their perceived detection risk at Ages 18, 21, 26, and 38. Summary statistics for these measures are presented in Table 1. Note that the focal crime types are not perfectly consistent across every phase. Phase 18 asked about shoplifting, car theft, burglary, and fraud. Phase 21 included those four, plus marijuana use, assault, and DUI. Domestic violence was added to Phases 26 and 38.
We began by observing mean scores for the perceived detection risk measures. In a later section, we will assess whether any observed changes over time were statistically significant. Here, we simply observed the mean values and assessed for possible trends.
Substantively, the mean values for the different risk measures did not appear to vary much over time. Take, for example, perceived detection risk for shoplifting, which was one of the four crime types that was consistently referenced at all four phases. On average, at the Phase 18 interview, participants rated the risk of detection as 0.525. This value can be interpreted as a probability, meaning when they were 18, participants perceived the probability of detection for shoplifting to be roughly equivalent to a coin flip. As participants aged, the average perceived probability of detection for shoplifting increased slightly: The average was 0.535 at Phase 21, 0.584 at Phase 26, and 0.623 at Phase 38. Turning to the other measures, we see that participants generally reported the highest perceived risk of detection when they reached Age 38.
As a preliminary assessment of trends over time, we produced line plots for each measure. Figure 2 has eight panels, one panel per crime referenced in the perceived detection risk questionnaire. The y-axis ranges between 0.00 and 1.00, reflecting the range of possible values on each measure. On the x-axis is time, which is represented by the four phases of data collection. The x-axis is spaced according to the time interval being represented, so the gap between Phase 26 and Phase 38 is larger than the others.
The top-left panel of Figure 2, for example, shows the values for the shoplifting question. The four black dots indicate the sample mean values observed at each phase. The bolded black line is the line of best fit for those values (i.e., a trend line). The light-grey lines in the background represent the trend lines for 20 randomly drawn Dunedin participants. We included these individual trend lines as a way to “bring life” to the analysis. It is a reminder that the means (the bold dots) can mask meaningful individual-level variation.
There are two takeaway messages from Figure 2. First, regarding perceived detection risk, there is substantively meaningful variation in the population. Variation exists in both the specific values that are reported, as well as the trend that exists over time. Many participants followed a “growth” trajectory, where they reported increasing perceptions of detection risk with each successive phase. But some participants report just the opposite, they perceive that detection risks decrease as they age.
The second takeaway is that, on average, there is minimal-to-small “growth” in perceived detection risk at the population level. The bolded black line is relatively flat or only has a slight incline in most of the panels of Figure 2. These observations are only preliminary; however, we will assess for statistically significant changes over time in a later section. Correlations between the perceived detection risk items over time are provided (see Supplemental Material C).
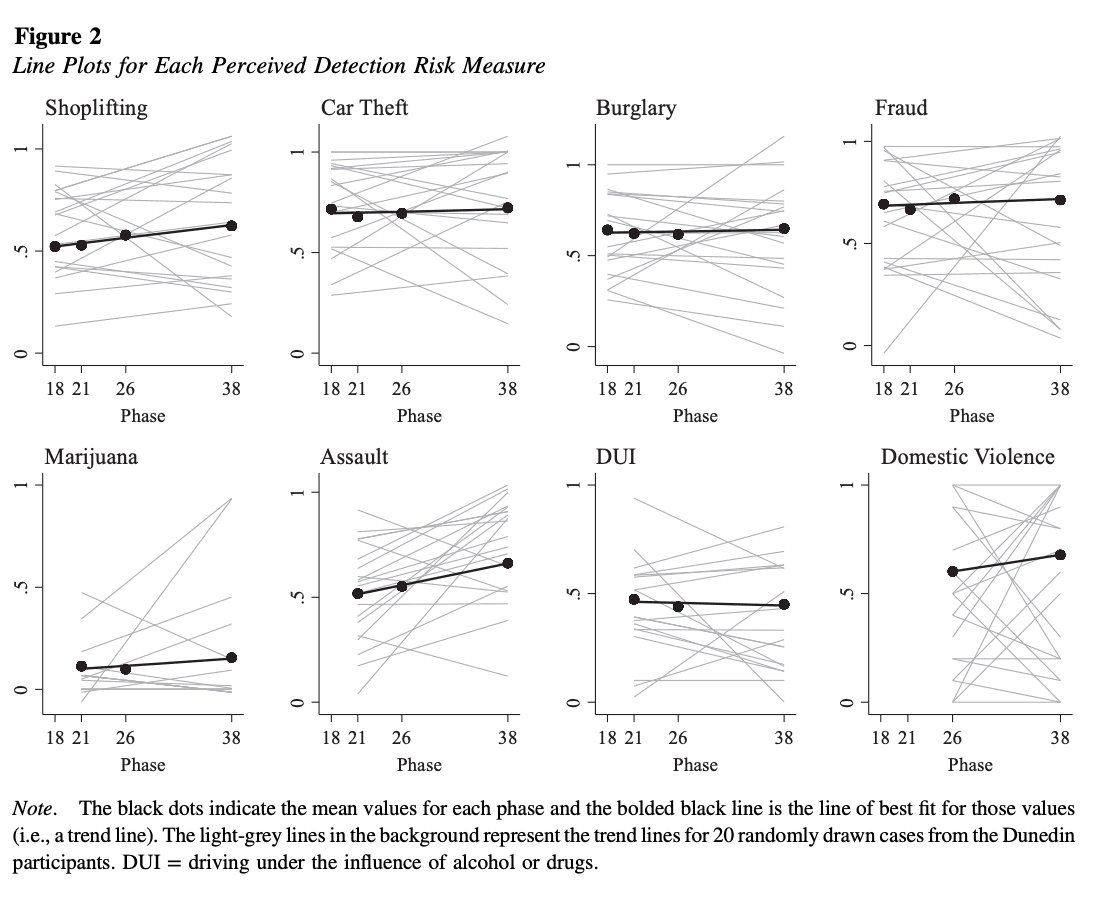
Note. The black dots indicate the mean values for each phase and the bolded black line is the line of best fit for those values (i.e., a trend line). The light-grey lines in the background represent the trend lines for 20 randomly drawn cases from the Dunedin participants. DUI = driving under the influence of alcohol or drugs.
Testing the Global Factor Hypothesis
Recall the global factor hypothesis predicted the measures of perceived detection risk for the different crimes would “hang together.” To test this hypothesis, we carried out correlation analyses, confirmatory factor analyses, and reliability analyses (i.e., Cronbach’s α and McDonald’s ω).
Bivariate correlations for all possible combinations of the focal variables are presented in Table 2. The table is arranged by phase and crime type. For example, the first four rows show the correlations between the four measures that were available at Phase 18. The next block shows the correlations for Phase 21, and so on.
For Phase 18, recall that four crime types were referenced: shoplifting, car theft, burglary, and fraud. These correlations fell in a moderate-to-strong range with values at Phase 18 reaching a low of r = 0.25 (shoplifting and fraud) and a high of r = 0.54 (car theft and burglary). These results reveal that if we were to select two participants at random and compare their scores on the perceived detection risk measures, the person who rated shoplifting as more risky than her peers would also be more likely to rate car theft and burglary as more risky.
A similar pattern and range of correlation values were observed for the other phases, though there were some notable exceptions, such as the correlation between car theft and domestic violence at Phase 26 (r = 0.07) and at Phase 38 (r = 0.05).
The next step was to carry out confirmatory factor analysis. Results from time-specific factor analyses are presented in Table 3. Standardized path coefficients are shown—this was achieved by constraining the variance of the latent factor to 1.00 in each model.
The results from the Phase 18 analysis indicated that all four items loaded together on a single latent factor with standardized path coefficients ranging between 0.47 (p < .001, 99% confidence interval [0.40, 0.53]) for the fraud item and 0.80 (p < .001, 99% confidence interval [0.74, 0.85]) for the burglary item. Reliability analysis of the four items revealed Cronbach’s α = .70 and McDonald’s ω = 0.70. Model fit statistics indicated a moderate fit to the data (X2 = 13.69, p = .001, root-mean-square error of approximation = 0.08, comparative fit index = 0.98). We also assessed the four items with exploratory factor analysis as a way to investigate whether more than one factor was necessary to capture the common variance. Only one factor emerged for the Phase 18 items in the exploratory factor analysis (see Supplemental Materials D and E). The single-factor solution was the best-fitting model for the Phase 18 data.
Phase 21 included the same four items from Phase 18 and added three new ones (Marijuana, Assault, and DUI). Confirmatory factor analysis (Table 3) indicated that all seven items loaded on a single factor with factor loadings ranging from 0.35 (p < .001, 99% confidence interval [0.28, 0.42]) for marijuana to 0.78 (p < .001, 99% confidence interval [0.73, 0.82]) for burglary. Reliability analysis of the seven items revealed Cronbach’s α = .73 and McDonald’s ω = 0.74. As with the Phase 18 results, we estimated exploratory factor analyses as a sensitivity check for a two- and three-factor solution (see Supplemental Materials D and E). Those analyses indicated a single-factor solution was the best-fitting model.
A similar pattern of findings emerged at Phase 26. The one-factor solution shown in Table 3 revealed that all eight items loaded on a single latent factor. But note there was variability in the strength of the loading coefficients. The Burglary item loaded on the factor at 0.82 (p < .001, 99% confidence interval [0.78, 0.85]) while the domestic violence item loaded at 0.24 (p < .001, 99% confidence interval [0.17, 0.30]). Reliability analysis of the eight items revealed Cronbach’s α = .76 and McDonald’s ω = 0.77. The exploratory factor analysis results (see Supplemental Materials D and E) also indicated a single-factor solution was the best-fitting model.
Finally, analyzing Phase 38 data led to a similar set of conclusions. The confirmatory factor analysis results in Table 3 revealed all items load on a single factor with loadings ranging from 0.31 (p < .001, 99% confidence interval [0.24, 0.37]) for the domestic violence item to 0.78 (p < .001, 99% confidence interval [0.75, 0.82]) for the burglary item. Reliability analysis of the eight items revealed Cronbach’s α = .80 and McDonald’s ω = 0.80. We assessed for a two-factor solution with exploratory factor analysis (see Supplemental Materials D and E). Careful scrutiny of the loadings revealed the first factor captured common variance in seven of the eight items. The domestic violence item was unique in that it loaded only on a second factor. There may be something unique about risk perceptions for domestic violence. This is consistent with the correlation analyses, which revealed low correlations between the domestic violence item and the other items. We encourage future work to explore this possibility with different data and with a wider variety of risk perception items. With the nuance about the domestic violence item in mind, we conclude the Phase 38 data provide partial support for the global factor hypothesis.
Before moving to the next step of the analysis, we created a scale tapping into global perceived detection risk for each phase of data collection. To calculate these global measures, we computed the mean of the four items that were consistently measured across all phases of data collection (shoplifting, car theft, burglary, and fraud).2 We restricted our analysis to these four items in order to harmonize the perceived detection risk measure for the longitudinal analysis. Calculating the mean of these four items allowed the scale to retain its interpretation as a probability value. Importantly, we found that the mean scores did not statistically differ from a scale created by extracting the factor estimated by the factor analysis (correlations between the mean scores and the scores derived from the factor analysis were greater than r = 0.95 for all four time points).
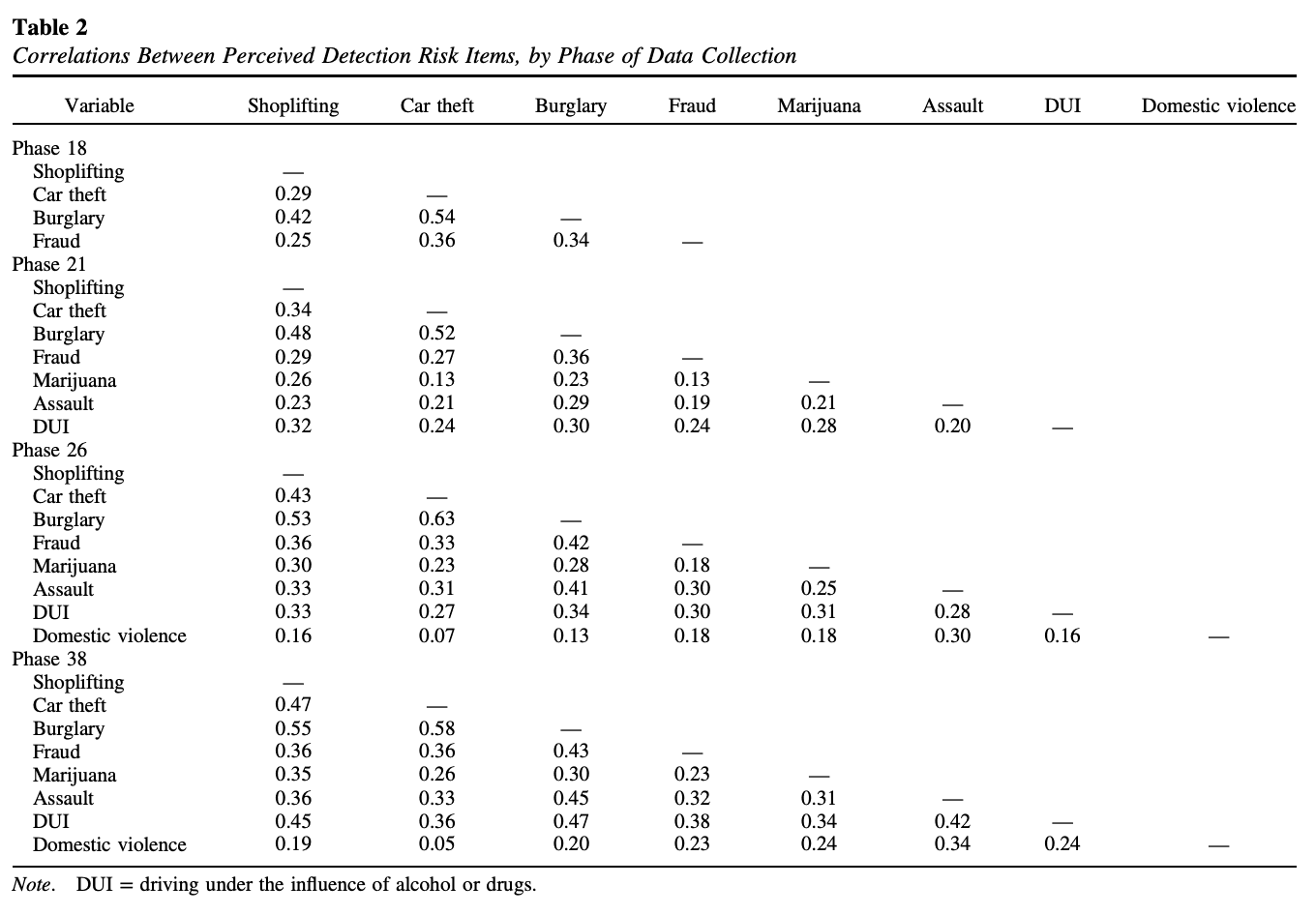
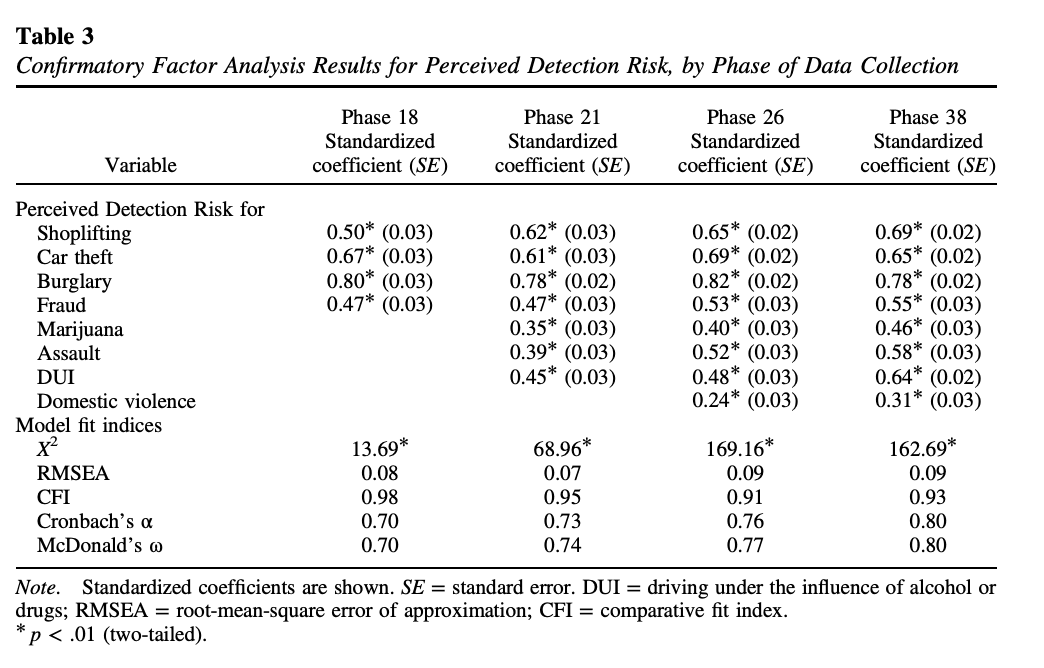
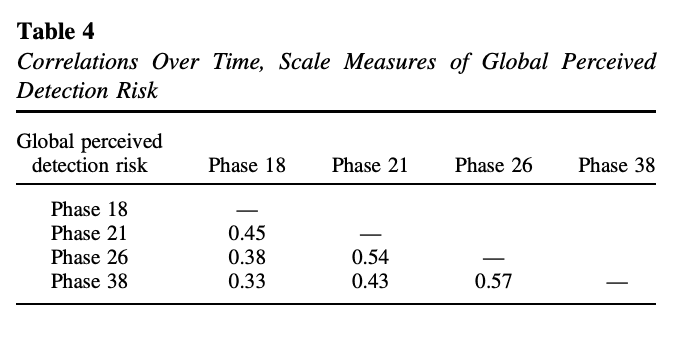
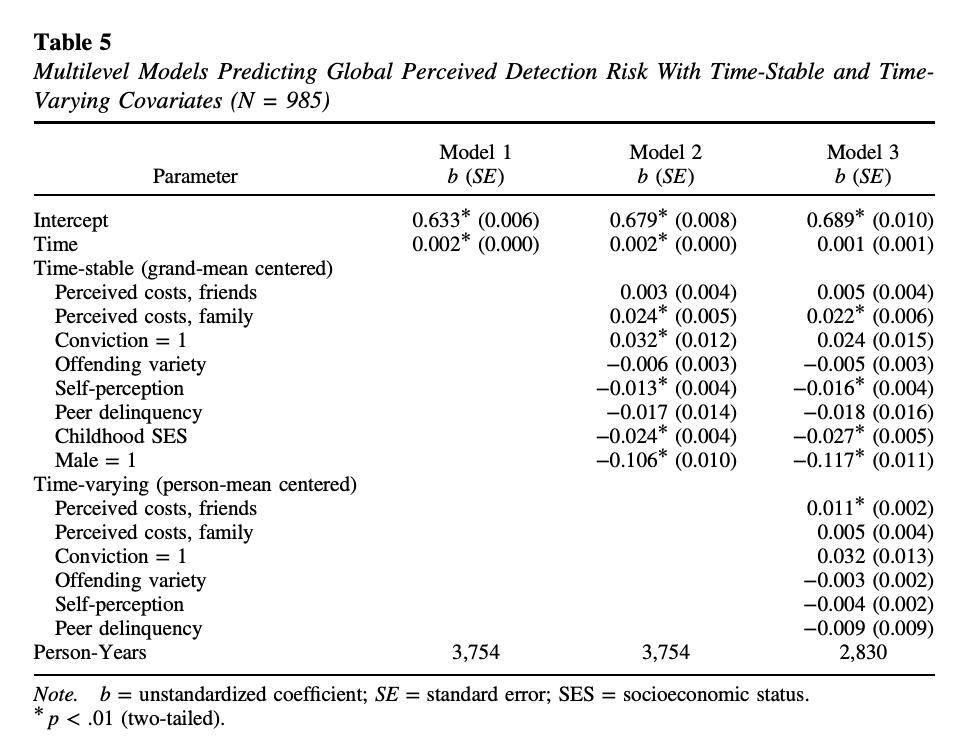
Testing the Age-Graded Hypothesis
Table 4 shows the cross-time correlations for the scale measures of global perceived detection risk. These correlations follow a familiar pattern observed in longitudinal personality research: The closer two measures are in time, the higher the correlations between them, and the earlier the measures are taken in the life course, the lower the correlations across time. Cross-time correlations for the individual domain items for perceived detection risk are provided (see Supplemental Material F).
The next step to the analysis was to test the age-graded hypothesis. This hypothesis anticipated population-level increases in perception of detection risk over time. We estimated a multilevel model for change (Singer & Willett, 2003) to assess the impact of time on the global measure of perceived detection risk. We began the multilevel analysis by estimating a random-intercept model, where the global perceived detection risk measure served as the outcome (results not presented in a table). This model revealed the average perception of detection risk (i.e., the intercept) was 0.650 (p < .001, 99% confidence interval [0.639, 0.661]) and there was a substantively significant amount of variation around that value in the sample (standard deviation = 0.149, 99% confidence interval [0.141, 0.159]).
Next, we entered a measure of time that reflected the number of years since Phase 18. The results from that model are presented in Table 5, Model 1. The intercept now reflects the average value of global perceived detection risk at Phase 18 (b = 0.633, p < .001, 99% confidence interval [0.621, 0.645]) and the time variable reveals the amount of increase in global perceived detection risk that occurs, on average, every year (b = 0.002, p < .001, 99% confidence interval [0.001, 0.003]).3
This result supports the age-graded hypothesis, which predicted that perceived detection risk would increase from adolescence to adulthood. However, the yearly increase is substantively trivial. Recall the outcome can still be interpreted on the probability scale. Thus, the average increase in perceived detection risk is estimated to be 0.002% points, or about 0.3% (100 × 0.002/0.633) each year.
Over the 20 year course of the study, participants increased their perception of detection risk by about 4% points (from about 0.66 to about 0.70) on average.
Testing the Costs-Risks Hypothesis
The next step to the analysis was to test the costs-risks hypothesis. This hypothesis anticipated individual differences in perceived detection risk would emerge over time as a function of changing social bonds, which were operationalized as perceived detection costs from family and perceived detection costs from friends. To test the costs-risks hypothesis, we again estimated a multilevel model. This time, we included the grand-mean centered time-stable variables as predictors of the intercept value for the global perceived detection risk outcome variable. Results from this model are presented in Table 5, Model 2. Recall that the time variable was centered on Phase 18, so the intercept reflects the predicted risk perception at Phase 18 for a female participant who scored the sample mean value (i.e., 0.00, because of grand-mean centering) on the timestable predictors.
Relevant to the costs-risks hypothesis, we see that respondents who perceived greater costs from their family also perceived higher detection risks of offending (b = 0.024, p < .001, 99% confidence interval [0.014, 0.034]). The time-stable measure of perceived costs from friends was not statistically significant.
In Model 3, we entered the time-varying versions of each predictor. Here, we see that respondents reported a higher perception of detection risk during times when they perceived a greater cost from friends (b = 0.011, p < .001, 99% confidence interval [0.006, 0.016]). This result indicates that participants increased their perception of detection risk by 0.011% points, or about 1.6% (100 × 0.011/0.689), during times when they increased their perception of cost from friends by one unit (e.g., moving from a response of “no” [coded 0] to “maybe” [coded 1] for just one item in the scale). There was no such relationship with the perceived costs from family variable.4
Testing the Experiential Hypothesis
To test the experiential hypothesis, we entered the time-varying indicator of conviction status into the multilevel model. As shown in Model 3 of Table 5, the conviction variable had a positive association, but it failed to reach statistical significance (b = 0.032, p = .015, 99% confidence interval [0.006, 0.057]). Thus, our results fail to support the experiential hypothesis.
Discussion
We sought to integrate the psychological study of perception of detection risk into a developmental/life-course framework. This is an important area of study because perceived detection risk is known to be a robust predictor of future criminal behavior (Apel, 2022) and prior analysis of the Dunedin Longitudinal Study—the data we relied on in the present analysis—confirmed that this relationship exists for the Dunedin participants (Wright et al., 2004). Our data spanned 20 years of development from Age 18 to Age 38. Our analysis found support for three of the four theoretically informed hypotheses, though the degree of support varied. Below, we will contextualize the contributions this study makes to the literature and theory. We will then consider the policy impact that might stem from these data.
Contributions to the Literature and Theory
Several informative results emerged from this study. First, the data suggest that, on the whole, it is likely that a global factor explains much of the variance in the perception items. Rather than holding a unique perception of detection risk for individual crime types, our analysis of the Dunedin Longitudinal Study participants suggested that people may have a personal “baseline” perception and this perception informs their views about the risks of detection for most types of offending. One way to conceptualize this is to draw parallels to the setpoint model in the personality literature (Caspi et al., 2005). That model suggests environmental factors can cause fluctuations in traits, but over time many people will regress to their baseline personality.
None of this excludes the possibility that at particular ages, for some particular crimes, there are perceptions that stand on their own, separate from the “global” factor. We have no theory to guide us on this possibility, but this post hoc explanation could offer insight into the reason the correlations between the perception measures were lower than is typically reported in studies of the psychometric properties of personality traits. Similarly, model fit statistics from our confirmatory factor analyses do not achieve the level of fit that is common in research on personality traits.
It could also be that there is, in fact, a single factor, but it is not measured well in our data set. A validated and standardized interview protocol for measuring perceptions of detection risk does not yet exist. The perceptions of detection risk items are innovative and the interview protocol developed to measure them is unique to the Dunedin Longitudinal Study. Measurement error could have attenuated the correlation values and factor analysis fit indices. Future work is encouraged to explore the development of standardized questionnaires for risk perceptions.
The second finding to emerge from this study was that perceived detection risk increases, but only slightly, from Age 18 to Age 38. Between-person variation is mostly attributable to factors, experiences, and influences that occur in peoples’ lives prior to Age 18. Whether those cues come from the prevailing risks of crime from the childhood and adolescent environment (i.e., the actual detection risks) or something else (e.g., personality factors, family or cultural values) remains to be studied. Regardless, our findings suggest that adolescents do not systematically underestimate the risks of crime compared to their “older selves,” meaning the late-adolescent peak of the age-crime curve is not attributable to youthful ignorance.
The third finding from our study emerged when we analyzed within-person changes in perceived detection risk between lateadolescence and adulthood. There may have been only slight increases in perceived detection risk at the population level, but that does not rule out the possibility that some people show withinperson changes over time (Anusic & Schimmack, 2016; Schwaba & Bleidorn, 2018). Drawing on developmental perspectives, we hypothesized that people who perceived greater costs to offending from family and friends would also perceive greater risks of crime detection. Supporting that hypothesis, we found that individuals who carried a general sense that they could lose family if they were caught committing a crime tended to perceive higher detection risks throughout the study period. But we did not find a corresponding relationship as a time-varying effect. We did, however, find a timevarying influence for the perceived costs from friends. Specifically, during times when perceived costs from friends spiked, people tended to report higher perceived detection risks.
There are at least two explanations for this pattern. First, it could be that individuals perceive family of origin is forever. Thus, the risk of losing family is not something that varies over time, meaning it does not contribute to changes over the life course. The second explanation could be that individuals accept that their family may, at times, become upset with them but they know it will not last forever and therefore it does not have a transient influence on their perceptions. Friends, and the risk of losing them, can change, so this potential cost to engaging in crime affects other risk perceptions at any given time.
The fourth finding from this study has bearing on the experiential hypothesis, which predicted individuals who are convicted of a new crime will subsequently increase their perception of detection risk. Although 27% of the cohort experienced conviction, and the substantive pattern of results for this part of the analysis fit our expectation, the influence of conviction on perceived detection risk did not pass our threshold for statistical significance.
This study has several strengths that are worth noting. Much of the literature on perceived detection risk relies on cross-sectional data, so ours is among the first studies to take a long view of decision making and perception formation. Additionally, as we noted earlier, the broader decision-making literature often focuses on decisions that are not as risky as getting arrested and incarcerated. Criminal decisions are high stakes and, therefore, might invoke unique cognitive mechanisms compared to those invoked by many of life’s routine decisions. Crimes are also dated events, which allowed us to pin down the timing of these life events (especially conviction status) to better align them with changes in perception.
Implications for Policy
There are at least two points from this study that are worth underlining vis-à-vis policy discussions. First, our results suggest individuals form a perception of detection risk sometime during the first two decades of the life course and, at least at the population level, those perceptions do not change much over the next two decades. This means that attempts to affect perceptions of detection risk are unlikely to have much of an impact on adults’ perceptions. But such efforts may impact youth and that effect could be carried forward as those youth age. Recognizing this point might shift how policymakers think about the expected returns to policy changes; crime prevention strategies that work by changing perceptions may take a generation to show up in crime statistics. Supportive evidence comes from the study of “crime debuts,” which suggests that life-course-persistent offending can be deterred by small environmental changes—such as parking-lot lighting or video-camera surveillance—that makes it harder or discourages youth from beginning their criminal career (Farrell et al., 2015).
Second, it may be possible to “move the needle” on crime prevention by focusing on the risk of losing connections with friends. Our results suggest that individuals who perceive higher risks of losing ties with friends if they were caught committing a crime also perceived higher risks of getting caught. If this relationship turns out to be causal—even if it is a bidirectional causal relationship—then it may be possible to affect perceived detection risk by drawing attention to friendship ties that are wagered by criminal behavior. We can envision leveraging this finding, as just one example, with advertisements that highlight the risk of losing friends because of drunk driving. Rather than “friends don’t let friends drive drunk” it may be more effective to advertise “friends don’t stay friends with drunk drivers.” But any deterrent effect realized by such a campaign may prove to be temporary—recall the perceived risk of costs from friends was time-varying so it may have only a transient impact on perceptions of detection risk.
Limitations
Results from our analysis, and therefore the subsequent policy considerations offered here, should be assessed in light of the following limitations. First, our findings are limited to one birth cohort from the 1970s who were born in one city in one country. Despite the potential for selection bias, the Dunedin Longitudinal Study has proven to be a robust and consistent source of information that results in findings that are in keeping with American studies of crime and delinquency (Caspi et al., 1994). Nonetheless, we need more longitudinal studies that can unpack the role of perception formation in the criminal decision-making process. An interesting question is whether different historical relationships with the justice system impact risk perception formation for people from different ancestral groups. Unfortunately, our data do not allow us to speak to differential group outcomes as more than 90% of the Dunedin Longitudinal Study sample identify as White. We highlight this point for future work to consider.
Second, our data were observational, meaning we were unable to sort out causal relationships. We encourage future work to rely on research designs, such as experiments, that can address causal effects.
Third, our measures of perception were limited to mostly nonviolent acts, the exceptions being assault and domestic violence. Future work should aim to confirm our results—especially those that assessed whether perceptions could be summarized by a single factor—with a larger pool of more diverse offense types.
Fourth, our study drew from four phases of data that span 20 years of development. This represents, simultaneously, an advancement over the prior literature but also presents questions that should be addressed in other studies. The Dunedin Longitudinal Study allowed us to advance the literature because no prior work had been conducted on a longitudinal sample that spanned more than a few years of a segmented period of development. But it could be the case that the long time periods between our observation phases impacted the results. For example, there is some literature that suggests the “experiential effect” of contact with the criminal justice “wears off” over time (Kaiser et al., 2022). Like a vaccine that loses potency, it could be that the boost to our risk perceptions is eventually overwhelmed by all the other inputs that life has to offer. This might explain our lack of support for the experiential effect in this study.
Fifth, our study began when participants were Age 18. There are both strengths and limitations to studying an early adult population. The strengths for a study like this is that most people have already begun to engage in crime and deviance by Age 18. Thus, we had enough variation on the measures of interest to allow for the types of analyses that were conducted. A corresponding limitation, though, is that we are unable to disentangle the origins of our participants’ perception of detection risk. We encourage future work to study younger populations to better identify the individual traits, experiences, and environmental exposures that are the sources of variation in perception of detection risk.
Future Directions and Conclusions
This study found that individuals form perceptions of detection risk early in the life course, that those perceptions are more likely to be “general” rather than unique to specific crimes, that perceptions are largely stable at the population-level between late-adolescence and adulthood, but that individuals will update their perceptions when life circumstances change (e.g., after social bonds change). These findings are relevant to social psychology because they help us better understand the decision-making mechanisms that underlie the choice to commit (or not) a criminal act. But there is still work to be done to sort out questions about how perceptions form early in life. With this in mind, it will be important for future work to explore the role of personality and social psychological factors in the development of perception of detection risk during the first two decades of the life course.