Abstract
Sexual selection theory predicts that males, especially in their prime reproductive years, are more risk-prone than females. Risk-taking is a means to convey mate quality or “good genes” to members of the opposite sex or competitive ability to members of the same sex. Therefore, risk-taking should be more common in the presence of potential mates (intersexual selection or female choice) or in the presence of male competitors (intrasexual selection or male–male competition). Risk-taking can also be situated within a life history/ecological perspective, according to which environmental unpredictability promotes more present-oriented and risky behavioral strategies. Therefore, people living in areas of low socioeconomic status are predicted to be more risk-prone. We conducted an observational study on risk-taking in two everyday situations: crossing the road and riding a bicycle. A total of 1,030 participants, 906 road-crossers and 124 cyclists, were recorded at various intersections throughout Perth, Western Australia. In line with predictions from sexual selection, males were more likely to cross a road in high-risk conditions and to ride a bicycle without wearing a helmet. These behaviors were also most common in younger adult males. Female and male onlookers had no effect on male risk-taking, which suggests that norm adherence is a more powerful force than sexual selection in shaping risk-related behaviors. Area-level socioeconomic status was strongly associated with the frequency of risk-taking, implying that environmental stressors can affect risk attitude. Elucidating the ultimate drivers behind everyday risk-taking has important practical implications for risk intervention strategies.
Human males are the more competitive sex, especially in the realm of mate competition (Archer, 2009; Trivers, 1972). This fundamental sex difference derives from anisogamy (i.e., the observation that females generally produce a few but large gametes, whereas males produce large numbers of much smaller gametes) and males’ greater reproductive potential and variance in reproductive success (Bateman, 1948; Betzig, 2012). Sexual selection theory predicts that males, especially in prime reproductive condition, will also be more risk-prone than females because of the potential reproductive payoffs associated with this behavior (Wilson & Daly, 1985; but see also Salas-Rodríguez et al., 2021). Indeed, risk-taking is strongly sexually dimorphic in a variety of domains such as conflict and competition, outdoor activities, gambling, and financial decision making (Apicella et al., 2017; Byrnes et al., 1999; Howland et al., 1996; Powell & Ansic, 1997; Rieger et al., 2015; Wang et al., 2009; Wilke et al., 2014; Wilson & Daly, 1993; Xiang & Stallones, 2003). Males also report deriving more pleasure from engaging in risky activities (Harris & Jenkins, 2006), with adolescent and young adult males being particularly likely to undertake physically risky behaviors (Byrnes et al., 1999).
From a sexual selection perspective, risktaking with nonlethal outcomes can be interpreted as a means of demonstrating one’s competitive ability or suitability as an ally to members of the same sex; in this case, risk-taking is an outcome of intrasexual competition and is used in the arena of male–male competition (M. D. Baker & Maner, 2008; Farthing, 2005; Wilson & Daly, 1985). Risk-taking can also serve to convey reliable information about one’s genetic quality and skill competence to members of the opposite sex and thus be an attractant to potential suitors (intersexual selection or female choice; M. D. Baker & Maner, 2008, 2009; Hawkes, 1991; Kelly & Dunbar, 2001; Wilke et al., 2006; Wilson & Daly, 1993). Risk-taking has a sexual signaling function, which is supported by studies showing that men are more likely to display risky behaviors in the presence of females generally (McAlvanah, 2009; Pawlowski et al., 2008) or, more specifically, attractive (M. D. Baker & Maner, 2008; Greitemeyer et al., 2013; Ronay & Hippel, 2010) and romantically available females (M. D. Baker & Maner, 2009). In a gambling experiment using hypothetical money, McAlvanah (2009) demonstrated that viewing pictures of opposite-sex faces increased male risk tolerance. Similarly, reproductively motivated males made riskier decisions in a blackjack task after being exposed to pictures of very attractive females (M. D. Baker & Maner, 2008). This pattern is also seen in physical risk-taking. Ronay and Hippel (2010) showed that male skateboarders took greater physical risks when watched by an attractive female observer. In another study, activating a mating motive increased men’s propensity to risk-taking in a wide variety of domains (Greitemeyer et al., 2013). M. D. Baker and Maner (2009) reported a strong positive relationship between sexual arousal and risk-taking in men who were experimentally exposed to a romantically receptive female. These risk-taking behaviors appear to be proximately mediated by testosterone (Ronay & Hippel, 2010). Even in a virtual reality environment, males acted less risk averse in the presence of a female experimenter and female virtual observer (Frankenhuis et al., 2010).
Risk-taking can also be situated within a life history/ecological perspective, according to which environmental unpredictability or adversity can promote more present-oriented and risky behavioral strategies (Chisholm, 1999; Chisholm et al., 2005; Daly & Wilson, 2005; Griskevicius et al., 2013; Hill et al., 1997; Mishra et al., 2017; Wang et al., 2009). When environmental volatility prevails and the probability of attaining distant rewards diminishes, seeking more immediate rewards can be adaptive. Risky behavioral strategies can be a means to this end (Boon-Falleur et al., 2021; Chisholm, 1999). Risky behavioral strategies can also be the outcome of greater competition for resources and mates. Therefore, people living in areas of low socioeconomic status (SES; with lower environmental stability as a result of factors such as increased crime and poor health care) are predicted to be more risk-prone. By contrast, in a more stable and predictable environment where survival challenges are less of a concern and distant rewards are more realistic, the optimal strategy is to prioritize future planning and risk aversion.
One area where risk-taking is very common is traffic, which includes pedestrians, cyclists, and motor vehicle drivers. Traffic-related risk-taking is generally strongly male-biased. Male drivers are more likely than female drivers to speed and incur speeding infringements (Harré et al., 1996; Harris & Jenkins, 2006), drink and drive (Harré et al., 1996), not wear a seatbelt (Lerner et al., 2001), run red lights (Konecni et al., 1976), and be involved in (fatal) car accidents (Jonah, 1986; Turner & McClure, 2003; Waylen & McKenna, 2008; Wilson & Daly, 1985). Harrell (1991) found that male pedestrians were less cautious when waiting to cross signal-controlled intersections. Pawlowski et al. (2008) demonstrated that male pedestrians were more likely to cross a road when vehicles were on the crossing. Other studies reported a greater propensity for males to cross on red pedestrian lights (Rosenbloom, 2009; Tom & Granié, 2011). Traffic-related mortality is also higher among male than female pedestrians (Joly et al., 1992). Male cyclists were more likely than females to cross a railway track when it was dangerous to do so, that is, before the end of warning signals after a train had recently passed (Cobey et al., 2013). The same study also showed that men were more likely to violate the law by riding a bike without lights. Male cyclists are more prone to red light violations than female cyclists (M. Johnson et al., 2011, 2013) and less likely to use a helmet while riding a bicycle than women (Bolen et al., 1998; Harlos et al., 1999, but see Ritter & Vance, 2011).
Traffic-related risk-taking is not only sexdependent but also age-dependent. Young drivers (typically in their teens and twenties) have a higher probability of getting involved in a crash than older age groups (Turner & McClure, 2003). This translates into higher mortality for young male drivers (Wilson & Daly, 1985). Young drivers in particular are prone to take risks such as driving without a seatbelt (D. Baker et al., 2000; Nabipour et al., 2014) and driving aggressively (Jonah, 1990; Krahé & Fenske, 2002; Wickens et al., 2011). Harrell (1991) reported that younger pedestrians were less perceptive of risks and less likely to exhibit cautionary behavior prior to crossing signalcontrolled intersections. As for cyclists, young people are more disposed to red light violations than older people (M. Johnson et al., 2011, 2013).
A few findings from observational studies on traffic-related behavior suggest that risk-taking is modulated by the presence of an audience and thus serves a signaling function, as outlined above. For example, motor vehicle fatalities occur at higher rates when young people are accompanied by copassengers (Chen et al., 2000). The sex of the spectator has also been shown to influence male susceptibility to risk-taking in traffic-related situations. Male pedestrians are more likely to initiate a risky road crossing when there are females nearby (Pawlowski et al., 2008), a behavior consonant with a mate attraction motive. Jackson and Gray (1976) reported that male drivers turned more quickly on a busy street when they had a male passenger compared to when they were alone. Similarly, Nuyts and Vesentini (2005) showed that male drivers were less likely to wear a seatbelt in the company of a male passenger than when driving alone. These latter findings are congruent with the hypothesis that male physical risk-taking is evolutionarily intended to show off to other males.
We conducted an observational study on risk-taking in two everyday traffic-related situations: crossing a road and riding a bike. We first aimed to replicate the finding that males take greater risks than females when crossing a road (O’Dowd & Pollet, 2018; Pawlowski et al., 2008). We then also predicted that males would be less likely to wear a helmet while riding a bike than females. In line with sexual selection reasoning, that is, that young men have a disposition toward competitive risk-taking (Wilson & Daly, 1985), we predicted that young males would be the most risk-prone demographic group (both while crossing a road and riding a bike). To understand whether male risk-taking has a signaling function, we examined if crossing a road under unsafe conditions is more likely when there are male or female spectators in the immediate vicinity. Greater risk-taking in the presence of male onlookers could be interpreted as a manifestation of intrasexual selection or male–male competition. Greater risk-taking in the presence of female onlookers could be interpreted as a manifestation of intersexual selection or female choice. We also explored how risktaking by males is mediated by the presence of children (see Fischer & Hills, 2012); male sexual signaling may be dampened when children are present (because there is less to gain in terms of mate attraction) and males may model good behavior in such situations (e.g., because of strong norm-conforming forces).
Next, using a life history or ecological framework, we tested for environment-contingent variation in risk-taking. Specifically, we predicted that people in socioeconomically deprived areas are more inclined toward risk-taking than people in high-end suburbs. We also contrasted solo road crossers with group crossers. The prediction here was that group crossers would take greater risks than solo crossers. That is because individuals crossing alongside other individuals experience safety in numbers or dilution effect, whereby the per capita risk of traffic-related accidents (e.g., collision) declines as pedestrian volume increases (Bhatia & Wier, 2011). This argument is reminiscent of a well-known phenomenon in predator– prey ecology (see Lehtonen & Jaatinen, 2016) although the underlying mechanisms are likely different. Lastly, we explored the role of two contextual variables in moderating risk-taking: time of day and weather. We predicted that pedestrians would be more likely to jaywalk in rainy weather (to avoid getting wet) and when they are in a rush for work (typically in the morning).
Method
Data Collection
The observations on risk-taking in the context of crossing a road and riding a bike were conducted at intersections in the Perth metropolitan area. Intersections were stratified by suburb SES from the Australian Bureau of Statistics’ (ABS) Socioeconomic Indexes for Areas, specifically the Index of Relative Socioeconomic Advantage and Disadvantage (IRSAD). The index ranks suburbs on a scale from 1 = most disadvantaged to 5 = most advantaged (ABS, 2018). The data used to create this index were taken from the 2016 Census of Population and Housing, using income, education, and occupation variables (ABS, 2018). Thirteen suburbs in total were selected for the study, seven with a ranking of 1 (low SES) and six with a ranking of 5 (high SES). IRSAD values for these suburbs are detailed in Table S1 in the online supplemental materials.
There were several criteria that an intersection had to meet to ensure that observations were comparable across suburbs. The intersection was required to have at least one dual carriageway, speed limits of at least 50 km/hr, and be in a suburb(s) of low or high SES. Intersections in proximity to residential (rather than retail or business) areas were preferred to ensure the sample of participants was more likely to be local and therefore reflect the SES of the area. The intersection was required to have an acceptable level of foot traffic to ensure efficient collection of data. Discreet observing was paramount to this investigation; thus, each intersection was selected based on its proximity to an area where the observer’s location was not obvious.
Observational data were collected between the months of April and August 2021. Observations were conducted either from a café, park, or car. Observational sessions varied in length, with the average session lasting approximately 1 hr. The investigator ensured that sessions were conducted across different times of day and in differing weather conditions to enable these differences to be tested for in later statistical analyses. Individuals who visibly noticed the investigator were not included in the data. Participants were not approached for questioning of their motives. All data were collected in daylight to exclude visibility as a potential extrinsic confounder. A total of 906 road-crossing individuals were recorded.
Risk was defined as the likelihood of an individual being hit by an oncoming vehicle when crossing the traffic intersection. Three levels of risk (low, medium, and high) were then derived from this definition. Factors affecting the level of risk included the distance vehicles (moving or stationary) were from the intersection and the color of the traffic and pedestrian lights. These risk levels are detailed in Table S2 in the online supplemental materials.
Data Analysis
Prior to analyzing the data, we collapsed high and medium risk into one high-risk category because we had only 33 observations of high-risk crossings.
Chi-square tests were used to determine if risk level varied as a function of age, sex, presence of child, SES, weather, time of day, and group size. We then restricted the sample to males only and quantified associations between risk level and age, presence of child, and arealevel SES, respectively. We used Cramér’s V as a χ2 -based measure of association between two variables.
For the analysis examining the association between spectators and risk-taking, we excluded individuals judged to be older than 40 years of age (because sexual selection is at its strongest at younger ages). The predictor variable used here was the number of similar-aged spectators, with similar age defined as all individuals falling into the same age bracket as the male crossing the road. Differences in spectator numbers between the two risk levels were analyzed with a one-way analysis of variance. η2 was used to measure effect sizes in the analysis of variance models.
For the helmet-wearing analysis, we fitted a generalized linear mixed effects model with a binomial error structure and logit link function. Helmet use (yes vs. no) was modeled as the dependent variable. Fixed effects were age (perceived), sex, and SES. Perceived age was treated as a numeric variable; we used the lower end of the bracket as a continuous measure for age in the regressions. When age was treated as a dummy coded (ordered) factor, the results were comparable. To account for certain locations having a disproportionate effect on the dependent variable, we included location as a random effect. The two-way interaction between age and sex was not significant, and the model was thus rerun without the interaction effect. To establish the statistical significance of the combined set of predictor variables, we ran a likelihood ratio test comparing the full model with a respective null model containing only the intercept and random effect (Forstmeier & Schielzeth, 2011). We calculated marginal (variance explained by the fixed effects) and conditional (variance explained by the entire model, including both fixed and random effects) coefficients of determination following Nakagawa and Schielzeth (2013) and P. C. Johnson (2014).
Models were fitted in R Version 3.6.0 (R Core Team, 2019) using the function “glmer” of the package lme4 (Version 1.1-21; Bates et al., 2015). Confidence intervals were computed using the function “confint” and likelihood ratio tests using the function “anova”. R2 was calculated using the function “r.squaredGLMM” from the R package MuMIn (Barton, 2013). We used the package lmer Test (Kuznetsova et al., 2017) to calculate p values for fixed effect coefficients using Satterthwaite’s degrees of freedom method. We did not use an arbitrary threshold such as p ≤ .05 to designate a result as “statistically significant” (Amrhein et al., 2019; Valeggia & Fernández-Duque, 2021); instead, we report exact p values.
Results
Road Crossing—Males and Females Combined
Males were more likely to cross the road during risky times than females, χ2 (1) = 5.900, p = .015, N = 906, Cramér’s V = 0.081 (Figure 1a). Age was weakly associated with risky crossings, χ2 (5) = 10.005, p = .075, N = 906, Cramér’s V = 0.105 (Figure 1b); there was no linear increase between age and risk proneness, but people in their 60s and above were the most risk averse. SES showed a strong association with risktaking, with people in low-SES areas engaging in risk-taking more frequently than people in high-SES areas, χ2 (1) = 29.763, p , .001, N = 906, Cramér’s V = 0.181 (Figure 1c). When a child was present at the intersection, pedestrians were less inclined to take risks, χ2 (1) = 9.331, p= .002, N= 906, Cramér’s V= 0.101 (Figure 1d). There was no difference in the frequency of risktaking between people crossing solo and people crossing in a group, χ2 (1) = 0.735, p = .391, N = 906, Cramér’s V = 0.028. Weather (dry vs. rainy) was not associated with risk-taking, χ2 (1) = 0.323, p = .570, N = 906, Cramér’s V = 0.019. Time of day (afternoon vs. evening vs. morning) did affect risk-taking, χ2 (2) = 9.834, p = .007, N = 906, Cramér’s V = 0.104; the highest frequency of risk-taking was recorded in the morning (Figure 1e).
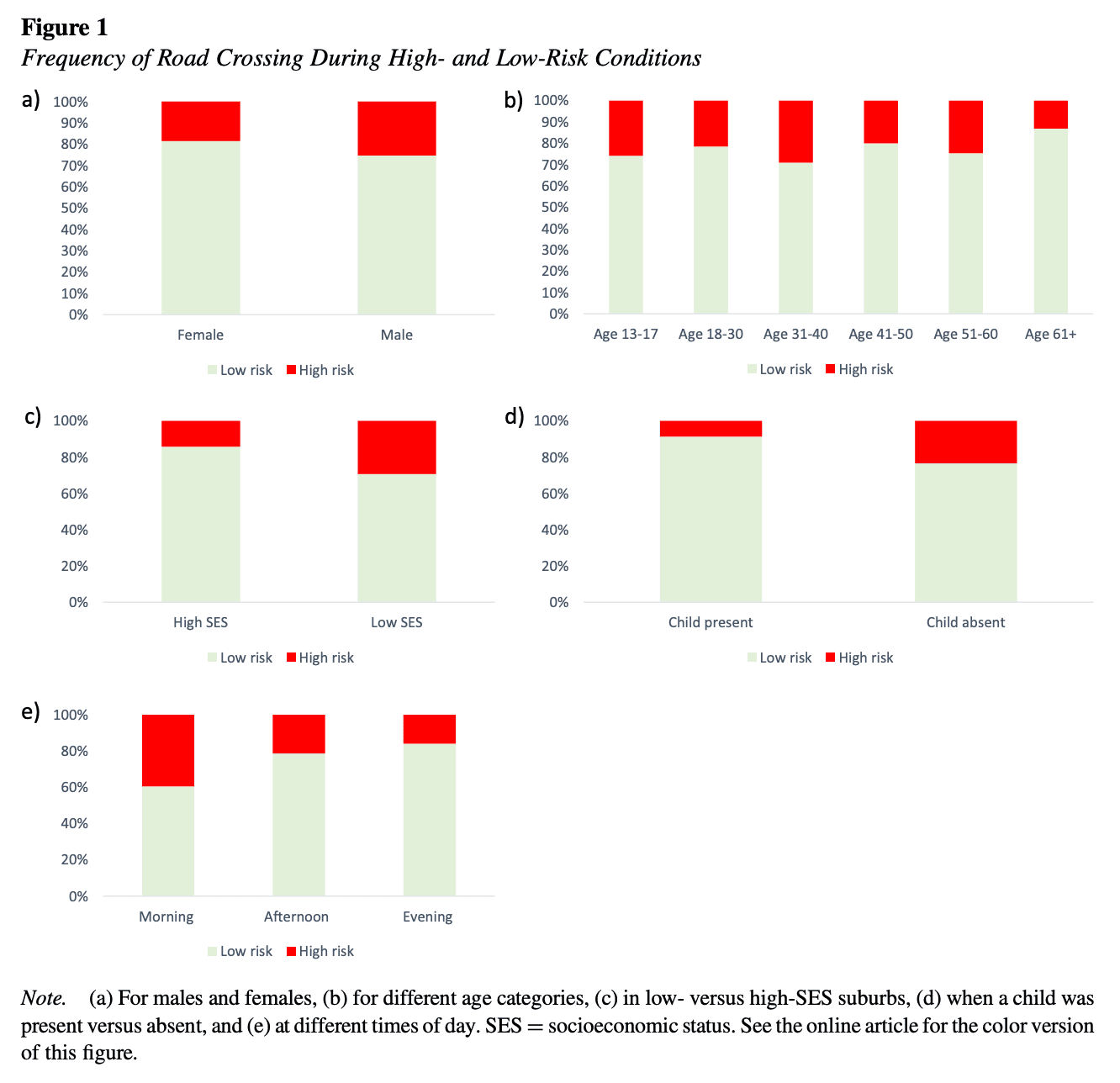
Road Crossing—Males Only
Risk-taking by males in the context of crossing a road showed variation across age groups, χ2 (5) = 12.721, p = .026, N = 475, Cramér’s V = 0.164 (Figure 2a), with males in the age group 13–17 years being the most riskprone. Risk-taking was also strongly stratified by SES, χ2 (1) = 8.908, p = .003, N = 475, Cramér’s V = 0.137 (Figure 2b); it was much more prevalent in low-SES suburbs than in high-SES suburbs. When a child was present, males were less prone to taking risks, χ2 (1) = 5.39, p = .020, Cramér’s V = 0.107 (Figure 2c).
There was no evidence for male risk-taking while crossing a road to vary as a function of the number of male or female spectators, male, F(1) = 2.512, p = .114, η2 = 0.007; female, F(1) = 0.072, p = .789, η2 = 2.132e−4.
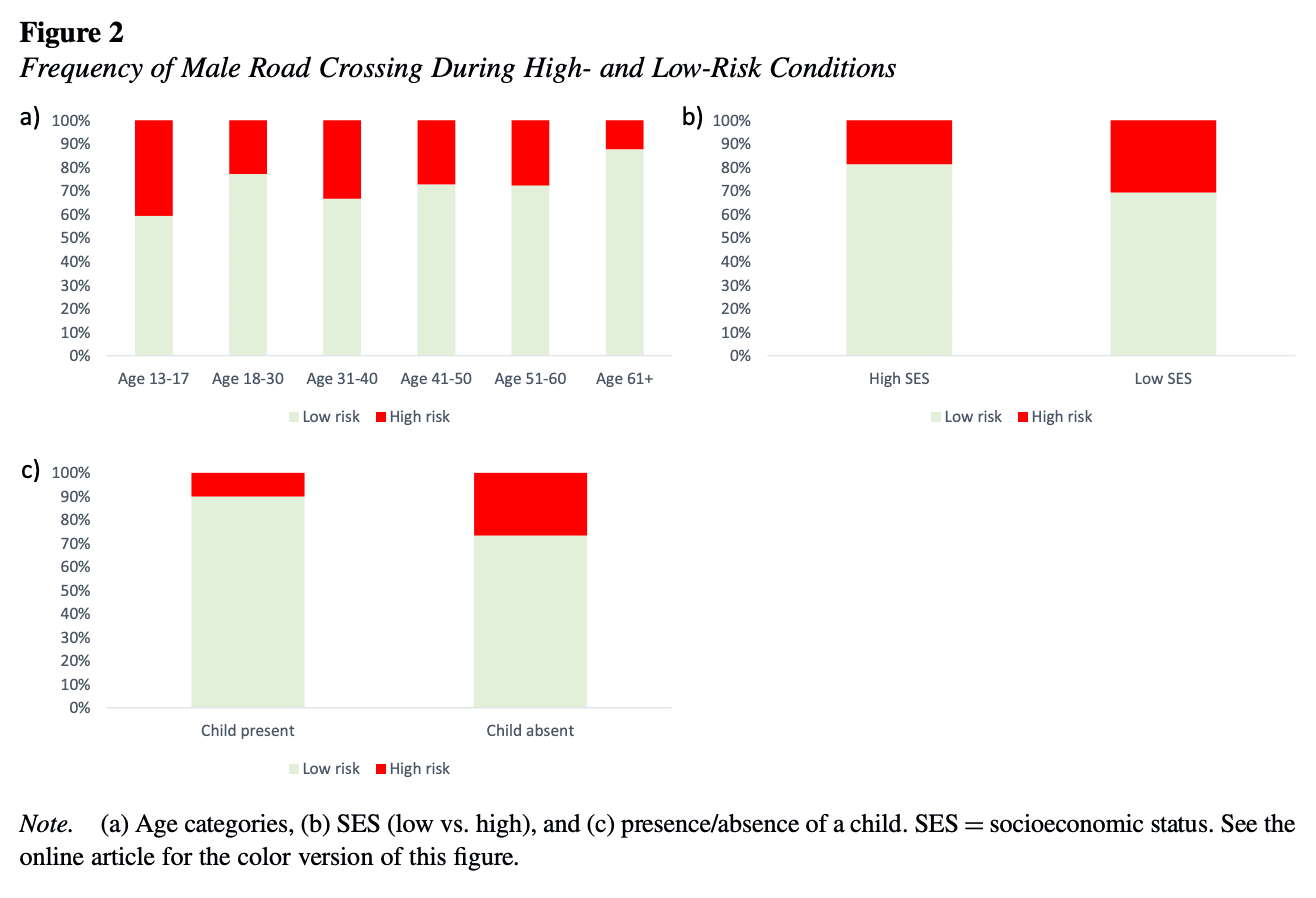
Helmet Wearing
The full generalized linear mixed model with SES, age, and sex as fixed effects was significantly different from the null model (χ2 = 33.958, p = 2.022e−07), with a marginal R2 of .312 and a conditional R2 of .425. The variance of the random effect (suburb), that is, the contribution of the random effect to the variation in the response variable, was 0.645, with a between suburb standard deviation of 0.803. The estimates, standard errors, p values, and 95% confidence intervals for the fixed effects are shown in Table 1. Male cyclists were less likely to wear a helmet than female cyclists (Figure 3a). The data revealed strong evidence that age was associated with helmet wearing (Figure 3b), with .70% of people in the youngest age category not wearing a helmet. Lastly, helmet wearing was much more common in high-SES neighborhoods than in low-SES areas (Figure 3c).
Discussion
This study revealed a pronounced association between sex and risk-taking both in the context of riding a bike and crossing a road. Males were much more likely to not wear a helmet when riding a bike and to cross a road under high-risk conditions. This finding is in keeping with the existing body of research on this topic and corroborates the view that there may be a predilection for risk-taking ingrained in the male psyche. Evolutionarily speaking, risk-taking reflects a manifestation of inter-male competition or female choice and is a tool in the arsenal of male fitness maximization. A possible proximate or mechanistic explanation for greater male risktaking (particularly when crossing a road) revolves around sex differences in impulsivity or patience (Bauer & Chytilová, 2013). While the sexual selection forces on male behavior likely account for significant variance in sex differences in risk-taking, it is also plausible that the results have been shaped by lower risk-taking in women. Lower risk-taking in women may reflect an adaptive fear response aimed at preventing bodily harm to women and their descendants (Campbell, 1999).
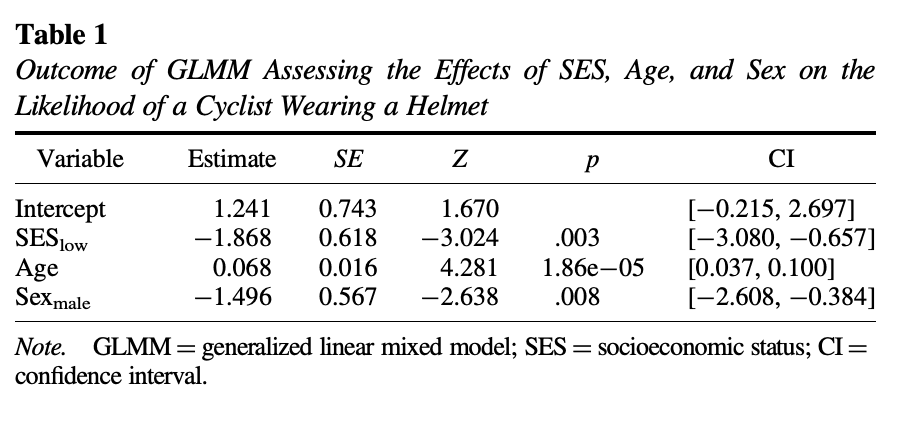
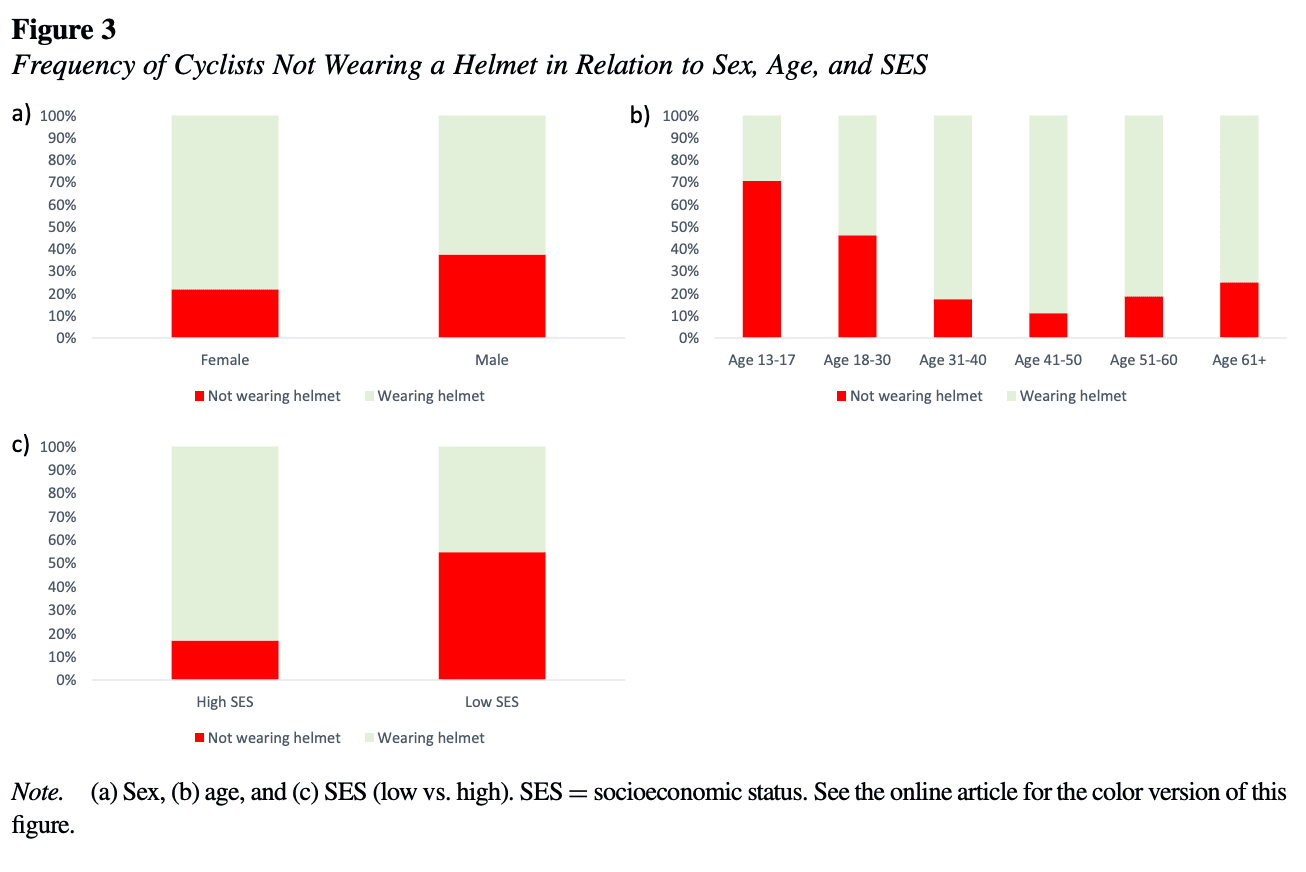
Another variable that was strongly predictive of risk-taking was age. Males and females in the oldest age category were least likely to cross a road during unsafe conditions. Males in the youngest age bracket were the most risk-prone when crossing an intersection. Riding a bike without a helmet was also mainly seen in the youngest age group. The greater representation of young males among risk-takers is consistent with the young male syndrome, which postulates elevated risk-taking during ontogenetic periods of peak reproductive competition (Wilson & Daly, 1985).
We also examined the validity of the argument that risk-taking has a self-presentational function. We found that risk-taking did not vary as a function of the number of male and female spectators of roughly the same age as the risk-taker. This does not rule out the possibility that risk-taking serves a communicative function in that it reveals information about a perpetrator’s “fitness profile.” The presence of similarly aged onlookers is a relatively crude measure of a male’s perceived pressure to engage in sexual signaling. For example, in this observational study, we cannot factor in the attractiveness of the female onlookers which may well influence male behavior. M. D. Baker and Maner (2008) report that exposure to attractive female faces elevated men’s risk-taking as long as a man’s mating motivation was high. Furthermore, we cannot deduce the relationship status of the pedestrians; partnered or invested men may be less incentivized to take risks in the presence of a female because the potential rewards may be limited (Frankenhuis & Karremans, 2012) and the disadvantages of risk-taking may be considerable. Moreover, there is longitudinal evidence that testosterone levels fall in males who become partnered (Gettler et al., 2011), which would likely be reflected in lower risk-taking inclinations. Since the presence of female onlookers had no effect on male risk-taking, this may mean that norm adherence trumps sexual selection in molding risk attitudes. Future studies should investigate if norm adherence in the context of risk avoidance is indeed an attractive trait.
The socioeconomic profile of a neighborhood (as measured by the IRSAD) emerged as a powerful correlate of risk-taking across situational contexts (road crossing for both sexes combined and for males only and helmet wearing). In the harsher and more unpredictable environments of socioeconomically disadvantaged areas, people’s time horizons are shortened, and behavior is biased toward impulsivity, reward-seeking, and risk-taking (Chisholm, 1999; Daly & Wilson, 2005; Hill et al., 1997). In such low-resource environments, where mortality is higher and lifespans are shorter and more unpredictable, risk-taking may be a viable strategy (Chisholm et al., 2005; Hill et al., 1997). It is possible that individuals from low-SES areas are more likely to engage in risky situations because they cannot afford to arrive late at work. A supplementary mechanism underlying the link between SES and risk-taking is that individuals of low SES have also been associated with increased health problems and higher alcohol consumption levels (Van Oers et al., 1999), which may affect or further reflect their risk judgment. It is unlikely to be the cost of a helmet that is prohibitive, as they are relatively cheap (compared to a bicycle), and infringements exist for jaywalking and not wearing helmets. One limitation of using area-level SES is that we do not know if the people we observed in a particular suburb are residents of that suburb. While people in low-SES suburbs are, on average, less well off than people in high-SES suburbs, exceptions are, of course, possible. Therefore, extrapolating from area-level SES to individual-level SES is not infallible. Another variable that may correlate positively with risk-taking but was not explicitly measured in this study is income inequality (Payne et al., 2017).
We also found that when a child was present at an intersection, both male and female pedestrians were less likely to take risks. Male sexual signaling may become deprioritized when children are present, either because there is less to gain in terms of mate attraction or inclinations to norm-oriented behavior take center stage. Previous studies have also shown greater caution at signal-controlled intersections when pedestrian volumes were low (Harrell, 1991). We, however, found no evidence for a difference in risk-taking between solo and group crossers. Another variable that was not associated with risk-taking was the prevailing weather. Perhaps weather conditions during observational sessions never reached a level of severity that made jaywalking “necessary.” Another finding of this study is that risky pedestrian behaviors were shown more frequently in the mornings, presumably because people were in a hurry to get to work, school, coffee, and appointments.
Given that risk-taking can result in severe physical injuries or even death, a deeper understanding of its correlates and determinants has implications for public safety education and urban planning around traffic intersections and is critical for effective and targeted intervention initiatives. For example, enforcement of helmet-wearing regulations should be prioritized in suburbs where helmet wearing is less frequently seen, that is, low SES. These data also show that continued public health campaigns and family messaging targeting risk-taking in young males are warranted. Our findings reinforce the need for government to continually strive to improve the environment in low-SES areas because the impact on risk-taking is so strong.
Conclusion
In conclusion, the present study corroborates predictions from sexual selection theory in that younger males were most likely to cross a road in high-risk conditions and to ride a bicycle without wearing a helmet. However, male risk-taking was not elevated when spectators were present. Area-level SES was strongly associated with the frequency of risk-taking, suggesting that risk attitudes are moderated by environmental context. To determine the generalizability of these findings and identify potential cross-societal or crossnational variation in risk-taking behavior, it is imperative that further replications in different urban settings are conducted.