Abstract
Development and learning are powerful agents of change across the lifespan that induce robust structural and functional plasticity in neural systems. An unresolved question in developmental cognitive neuroscience is whether development and learning share the same neural mechanisms associated with experience-related neural plasticity. In this article, I outline the conceptual and practical challenges of this question, review insights gleaned from adult studies, and describe recent strides toward examining this topic across development using neuroimaging methods. I suggest that development and learning are not two completely separate constructs and instead, that they exist on a continuum. While progressive and regressive changes are central to both, the behavioral consequences associated with these changes are closely tied to the existing neural architecture of maturity of the system. Eventually, a deeper, more mechanistic understanding of neural plasticity will shed light on behavioral changes across development and, more broadly, about the underlying neural basis of cognition.
INTRODUCTION
The brain undergoes dramatic changes early in life that coincide with both normative development and learning or experience. Both of these agents of change are supported by emergent neural processes that reflect and support behavioral modifications. As such, developmental cognitive neuroscientists have had a long-standing interest in understanding whether the biological substrates underlying learning and development are the same. While there is not yet a definitive answer to this theoretical, empirical, and philosophical question, technological advances in cognitive neuroscience tools, mainly magnetic resonance imaging (MRI), functional MRI (fMRI), and electroencephalogram (EEG), have provided a unique opportunity to examine neural and behavioral changes in tandem.
Development and Learning: The Problem of Definition
Development and learning are tightly interwoven constructs. A definition for each that distinguishes it from the other remains elusive, but I will attempt to do so here for the purposes of clarity in this article. Here, development refers to change in an organism as a result of growth, maturation, and/or experience while learning refers to the acquisition of a skill or gain of knowledge through study, instruction, and/or experience. As experience is common to both constructs, disentangling the two has been an intellectual and practical challenge. In fact, some developmentalists have argued that the two could be considered inseparable, as learning occurs within a developmental framework [Casey et al., 2006]. Others have attempted to distinguish between the two by noting that individuals learn skills after hours, days, and months of practice while developmental change occurs over weeks, months, and years, and evolution over a much longer time period [Smith and Thelan, 2003]. I would argue for an additional conceptualization of the two that development and learning exist on a continuum, with each endpoint receiving inputs from experience-expectant and experience-dependent processes (see Fig. 1 for a working model of this idea). In this view, while both development and learning are mechanisms that induce neural and behavioral plasticity, development is an emergent phenotype largely influenced by experience-expectant mechanisms while learning receives greater experience-dependent influence. As defined by Greenough et al. [ 1987], experience-expectant mechanisms utilize environmental information that has been common to all members of a species across evolutionary history. That is, the neural system comes to “expect” an experience under normal development, such as seeing contrast borders or receiving language input, to shape sensory and motor neural systems. Experience-dependent mechanisms, in contrast, are sensitive to the specific inputs the individual experiences. While experience-expectant mechanisms share common developmental time points across individuals (e.g. visual experience is expected at roughly the same developmental time point, shortly after birth), experience-dependent mechanisms are more fluid in timing, as unique experiences and learning opportunities differ in developmental timing among individuals. As such, plasticity that emerges from typical development represents neural change that is following the phylogenetic norm; in contrast, plasticity that emerges following learning represents neural changes associated with experience that is specific to the individual. Although one or the other mechanism may have greater influence on the organism at different points in life, that organism is ultimately the product of its developmental and experiential environment, both of which are shaped by experience-expectant and experience-dependent mechanisms of change. Language learning is a classic example of the shift from plasticity based on experience-expectant mechanisms to plasticity shaped by experience-dependent mechanisms [Doupe and Kuhl, 2008]. Kuhl et al. have demonstrated this phenomenon in a plethora of experiments. They have shown that young infants can discriminate phonetic speech sounds from all languages [Eimas et al., 1976; Kuhl and Meltzoff, 1982]. However, with exposure to language, the infant eventually loses this ability while simultaneously becoming increasingly proficient at their native language [Kuhl et al., 1997]. Their data elegantly show how biological predispositions (experience-expectant development) are subsequently modified by experience (experience-dependent learning).
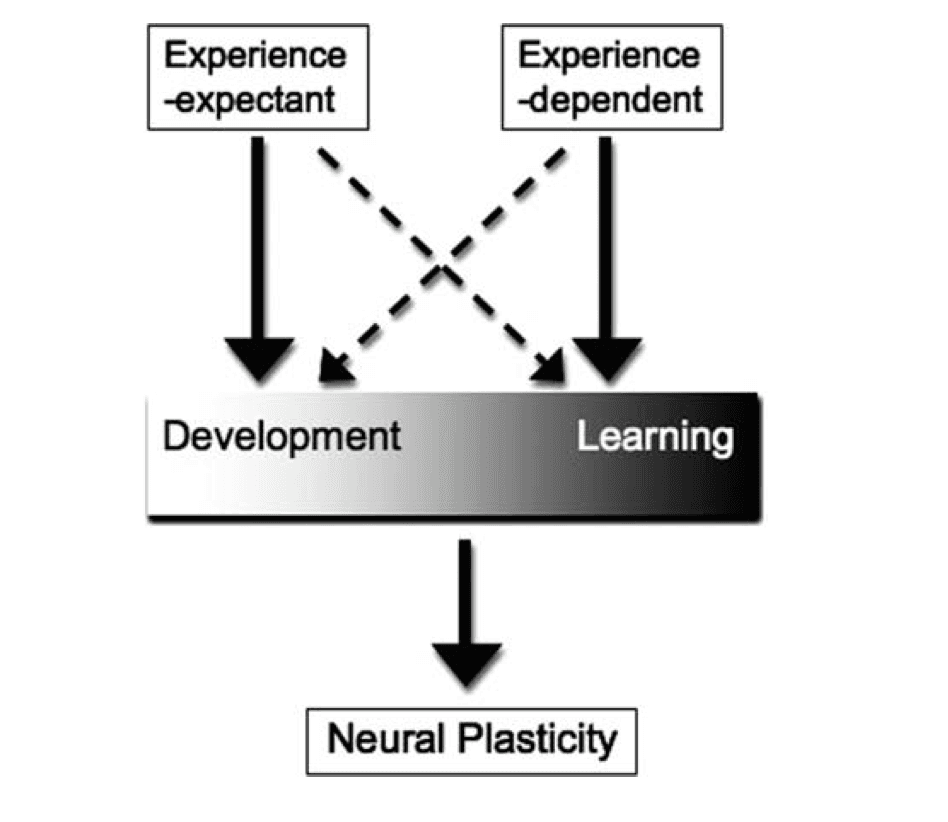
Figure 1: This working model illustrates that development and learning exist on a continuum, as each independently and simultaneously influence neural plasticity. While development is largely guided by experience-expectant mechanisms, it also receives input from experience-dependent mechanisms. Similarly, learning is mostly guided by experience-dependent mechanisms, but also receives experience-expectant input (72 × 72 DPI).
The notion that development and learning are complementary processes is not new. Karmiloff-Smith [ 1994] introduced the idea that together, they involve the gradual process of making behaviors more habitual while simultaneously, increasing explicit accessibility (as when learning a new task or skill). Another reason to believe that these constructs are not entirely separable, but rather that neural mechanisms are shared between the two, is that evolution tends to modify and borrow from existing systems, as opposed to creating entirely new ones to serve a similar purpose [Greenough et al., 1987]. As Karmiloff-Smith has argued, “A specifically human way to gain knowledge is for the mind to exploit internally the information that it has already stored, by redescribing its representations or, more precisely, by iteratively rerepresenting in different representational formats what its internal representations represent” [Karmiloff-Smith, 1994]. What will of course differ between the immature and adult system are the internal representations already available to them when new experiences are introduced into the system; the mature system will have a broader repertoire of previous experience. For instance, an axon that is growing toward a target has a very different landscape to navigate in the infant than in the adult brain. In this review, the terms learning and development are not used interchangeably but their linked effects on the developing system are implicitly acknowledged and appreciated.
Neural Plasticity
Neural plasticity is one of the most fascinating and challenging questions in neuroscience. Almost five decades ago, Hebb established a theoretical framework describing the phenomenon that the brain adapts to its environment based on experience and development [Hebb, 1949]. According to the theories of neuroplasticity, thinking and learning change both the brain's physical structure and functional organization. Basic mechanisms that are involved in plasticity include neurogenesis, programmed cell death, and activity-dependent synaptic plasticity. Repetitive stimulation of synapses can cause long-term potentiation or long-term depression of neurotransmission. Together, these changes are associated with physical changes in dendritic spines and neuronal circuits that eventually influence behavior. These same mechanisms stand out as important contributors to the developing brain's ability to acquire new information, adapt to the rapidly changing environment and recover from injury [Johnston, 2009]. As reviewed in several other articles in this issue, neuroimaging has significantly contributed to the study of neural development. It will undoubtedly serve important roles in disentangling neural substrates of development and learning.
Neuroimaging tools to study human neural plasticity
In previous studies of plasticity using neuroimaging, two main experimental approaches have been employed. While structural and functional approaches measure distinct neural attributes (delineated below), examples from studies which measure each are intermingled here to highlight examples of the two experimental approaches, cross-sectional and longitudinal, most commonly used to examine neural plasticity.
The first approach is a cross-sectional approach, in which individuals with varying levels of a given skill are compared and differences in neural structure or function related to their skill level are identified. For instance, in a study measuring structural change, Elbert et al. [ 1995] examined the relation between increased cortical representation of the fingers of musicians relative to those of nonmusicians. They found that musicians showed larger cortical representations and that the extent of this representation was correlated with the age at which the person had begun to play. Similar findings have been reported in the auditory cortex of musicians relative to nonmusicians [Pantev et al., 1998], suggesting that neural plasticity of relevant cortices depends on use and changes to accommodate the needs and experiences of the individual. The cross-sectional approach is often used in developmental and/or clinical developmental studies. There are numerous examples of this approach in the functional imaging literature, showing distinct neural activation patterns across age that correspond to differences in cognitive ability [Bunge et al. 2002; Casey et al., 1997; Durston et al., 2002; Luna et al., 2001; van Leijenhorst et al., 2007]. While their statistical power limitations are often acknowledged, it is argued that the major savings in time and cost of cross-sectional studies commonly used with fMRI, relative to longitudinal studies, make them an attractive alternative [Kraemer et al., 2000]. However, cross-sectional analyses may falsely suggest changes over time through confounds accidentally introduced into the study design, such as a time scale related to the variables of interest [Kraemer et al., 2000]. In addition, cross-sectional studies may suffer from cohort effects, in which different groups (e.g. children and adults; musicians; and nonmusicians) differ from each other by factors other than the factor of interest. For example, children growing up in the last 10 years will have received much more experience with computers than children growing up 30 years ago [Poldrack, 2000].
The second strategy to study neural plasticity is the longitudinal method. In this approach, participants are examined multiple times over the course of learning or development. In essence, training is a way to flood the organism with experience-dependent processes by saturating it with one particular experience. In the first experiment of this sort, structural changes were examined in rodents. Rats were trained on a changing series of patters in the Hebb-Williams maze during a period of 25 days [Greenough et al., 1979]. They found that in the visual cortex of trained animals, two types of neurons had more dendrites than in nontrained animals, while a third neuron type was unaffected. Thus, these findings demonstrated the specific and robust effects of training on synaptic connectivity. In humans, functional neural activation is assessed in a cognitive task before and after (and sometimes during) training on a task, in comparison with a baseline task that is not practiced [e.g., Karni et al., 1995; Karni and Sagi, 1993]. Then it is determined whether brain activity has changed in association with training on the task. The main advantage to this approach is that it provides optimal power to identify neural changes associated with the experience of interest because it is within-subject. However, in addition to the logistically challenging and expensive nature of longitudinal work, practice effects could potentially introduce confounds, as participants may acquire skills related to participation in the study.
Recent advances in the use of transcranial magnetic stimulation (TMS) have demonstrated the utility of this tool in the study of neural plasticity as well. Since its introduction, it has been known that repeated TMS (rTMS) of the motor cortex in healthy adult human participants can lead to relatively lasting effects (usually of the order of 30–60 minutes) on the excitability of the corticospinal output [Rothwell, 2007; Siebner and Rothwell, 2003]. For instance, a recent study showed that rTMS delivered to the superior temporal cortex causes macroscopic cortical changes in gray matter in the auditory cortex as early as within 5 days of continuous intervention [May et al., 2007] and work in patient populations has also uncovered the utility of this tool in investigating plasticity in the diseased brain [Rothwell, 2007]. Collectively, these approaches highlight the utility of neuroimaging tools in studying human neural plasticity.
The distinction between structural and functional changes
While experience can influence both structural and functional changes, it is important to note that there are clear distinctions between the two. Structural changes, as measured with MRI, typically refer to how experience influences anatomical changes. This change is indexed as volumetric differences in morphometry of particular brain region. Functional changes instead refer to differences in neural activation patterns following a particular experience. The functional methodology measures changes in blood oxygenation in the brain that are assumed to reflect changes in brain activity [Logothetis et al., 2001]. Structural and functional changes are not synonymous with each other, as different mechanisms likely underlie the two. Further, each can and do occur without the other. For instance, it is quite possible to observe functional remapping without any significant structural changes. Similarly, morphometric changes in a particular brain region do not always confer functional and/or behavioral changes.
Progressive and Regressive Changes With Learning
Most studies on neural plasticity have been conducted in adults and have yielded important insights that help inform developmental work. At the structural level, one of the first studies demonstrating the effects of experience on the brain showed that London taxi drivers had posterior hippocampi that were larger than controls in proportion to the length of driving experience [Maguire et al., 2000]. This study showed that the hippocampus, which is critical for spatial representation, is structurally altered by increased navigational experience. Subsequent studies showed structural changes using a variety of training paradigms, including complex visuomotor tasks such as juggling, [Draganski and May, 2008; Draganski et al., 2004] and music training [Zatorre et al., 2007], which each yielded changes in grey matter in motor cortex and regions in the parietal sulci. Together, these studies provide evidence that regions relevant for the task at hand are directly influenced by experience.
Using functional MRI, several groups have shown that training or experience is related to functional neural changes. However, these studies have yielded mixed results and interpretations. Numerous articles have addressed this topic [e.g. Draganski and May, 2008; Poldrack, 2000], so only a brief review of these findings will be provided here [for review, see Kelly and Garavan, 2005]. While some studies have shown general decreases in neural activity following training [e.g. Chein and Schneider, 2005], others have shown general increases with training or experience [e.g. Karni et al., 1995; Westerberg and Klingberg, 2007]. One explanation for the observed decreases in cortical activity with training or learning is based on the dual-processing framework of learning, which posits that a set of central resources mediating controlled processing is assumed to play a critical role in scaffolding novice performance, but becomes less essential as skilled, automatic processing emerges [Chein and Schneider, 2005]. With training, therefore, changes in fMRI signal are generalized as decreases in the extent and/or magnitude of activity, which may reflect local changes in synaptic efficacy [Haier et al., 1992]. Further, synaptic changes are assumed to affect the strength of local associations by tightening connections between neurons that contribute effectively toward task processing and weakening connections between those that do not. Across development, this phenomenon results in selective pruning of synapses that are overproduced early in development [e.g. Boothe et al., 1979; Huttenlocher, 1979]. As development collides with experience, extra synapses are lost, such that the final system consists of synapses that were selectively retained [Changeux and Danchin, 1977].
In contrast, several reports have shown general increases in activation with learning in both developmental and adult studies. For instance, Klingberg et al. reported that increased prefrontal and parietal activation was related to working memory capacity in children [Klingberg et al., 2002]. In adults, several groups have reported increased activation following skill learning [Karni et al., 1995] and other interventions such as meditation [Davidson et al., 2003], suggesting that these findings reflect the recruitment of additional cortical units with practice. This view is in agreement with the constructivist manifesto proposed by Quartz and Sejnowski [ 1997], which posits that there is a ‘progressive increase in the representational properties of cortex’ and that connectivity progresses from fewer to greater connections.
While strong evidence exists for both progressive and regressive changes (i.e. increasing and decreasing neural activation involved in cognitive tasks), change in a system from an immature to mature state is a product of both. This dual influence directly stems from the contribution of experience-expectant and experience-dependent mechanisms. While experience-expectant mechanisms encourage the elimination of unnecessary synapses or neural units (regressive change, presumably indexed by decreases in fMRI signal activity), experience-dependent mechanisms will guide activity-dependent creation and strengthening of synapses based on the individual organism's experience and needs (progressive changes, presumably indexed by increases in fMRI signal). Experience-expectant processes found in early development seem to assemble an excess of synapses, which are then selectively pruned back by experience to a functional subset. In later development and learning in adulthood, synapses appear to be generated in response to events that provide (learned) information to be encoded in the nervous system. At the synaptic level, this phenomenon is called metaplasticity, and refers to the notion that properties of synaptic plasticity can change as a function of previous plasticity and previous activation of synapses [Kalantzis and Shouval, 2009]. In other words, plasticity itself is plastic and the way the brain changes in response to its environment is contingent on the existing neural environment, the cause of (i.e. learning or development or both) and the behavioral consequences of the change.
What are the mechanisms of structural and functional neural changes?
The pronounced contributions of neuroimaging have been documented extensively. However, the conflicting findings reviewed above demonstrate how limited resolution continues to constrain interpretations of structural and functional neuroimaging data. This restriction is further complicated when examining developmental data, as described in more detail in this issue [Poldrack, 2010]. Our interpretations of increases and/or decreases in neural activity and grey matter are merely informed speculations of how they relate to neural plasticity, most of which are deduced from insights in molecular and cellular investigations.
Animal studies suggest that increases in cortical grey matter are the result of a complex array of morphological changes including synaptic events such as the formation of new connections by dendritic spine growth and alterations in the strength of existing connections [Chklovskii et al., 2004; Draganski and May, 2008; Hirata et al., 2004; Holtmaat et al., 2006; Trachtenberg et al., 2002]. In animals exposed to enriched environments, increased size of the soma and nucleus of neurons, glia and capillary dimensions have also been shown to influence cortical morphology [Kozorovitskiy et al., 2005; Muotri and Gage, 2006]. In addition, further mechanisms linked to training and experience-related plasticity include changes in the synaptic contacts known to be the morphological substrates of long-term potentiation and long-term depression [Draganski and May, 2008], synaptic pruning [Huttenlocher, 1979], changes in gene expression [Kleim et al., 1996], protein synthesis [e.g. McAllister et al., 1995], and dendritic density [Comery et al., 1995].
The Plasticity of Developmental Timing
Understanding whether the same neural mechanisms underlie both development and learning will address larger questions about developmental timing and experience-expectant processes. Are there certain cognitive processes that can be “sped up” with training or developmentally prolonged with experience? Animal work has suggested that the length of time that the developing nervous system remains sensitive to experience-expectant events can be manipulated. Cynader and Mitchell [ 1980] found that kittens reared in the dark until 6, 8, or 10 months of age remained highly sensitive to monocular deprivation effects. In contrast, kittens reared normally (i.e. reared in the light), show peak sensitivity to monocular deprivation within the first 2 months of life [Hubel and Wiesel, 1970]. In humans, the most insightful experiments to address these questions have been conducted in infants. In these experiments, researchers introduce tools that facilitate motor skills early in development, before the age at which these behaviors are typically observed. Although most of these studies do not have associated measures of neural activity, they provide considerable insight into how experience-expectant processes can be manipulated to occur earlier that is developmentally expected.
Needam et al. [ 2002] have shown that early, simulated experience serves to jump-start processes that are considered to be developmentally constrained. That is, by providing infants with scaffolding tools that they are not typically exposed to before a certain age, they can be trained to exhibit certain characteristics earlier than normal. In general, infants do not systematically reach for objects until ∼ 5 months of age [Butterworth and Hopkins, 1988; Rochat, 1993]; this is likely a reflection of their relatively immature gross motor skills (e.g. arm and hand strength, fine motor control) before this time [Halverson, 1933; Jeannerod, 1984]. Needham et al. [ 2002] tested 3-month-old infants (before spontaneous effective reaching and grasping) using an “enrichment experience.” The enrichment experience consisted of 12 to 14 brief parent-led object play sessions held at the infant's home. During the play sessions, the infant sat on a parent's lap at a table and wore mittens with the soft side of Velcro covering the palms. On the table in front of the infant were small, lightweight objects with edges covered in the corresponding side of the Velcro. With a quick swipe of the hand, the infant could easily “pick up” an object as it stuck to the mitten. After the enrichment phase for the infants in the experimental condition, these infants as well as the infants in the control condition (who did not play with the “sticky mittens”) were taken to the lab for an assessment of object exploration skills. Infants who had had the enrichment experience showed accelerated reaching behavior toward the new objects compared with control infants, even when not wearing the sticky mittens. These data suggest that experience may be a critical factor in manipulating processes considered to be under developmental constraint.
Rovee-Collier's conjugate reinforcement paradigm [see Rovee-Collier and Hayne, 2000] also produced observable actions on objects by infants before they typically do so on their own. In this paradigm, a ribbon is tied to an infant's ankle and the other end is tied to a mobile stand; once an infant makes the association between their ankle and the rewarding mobile movement, the infant's rate of leg kicks increases sharply. This is an additional evidence that producing actions on objects with observable effects is highly reinforcing for young infants. It remains an open question whether this experience with leg-kicking and mobile-moving would generalize to other abilities. These experiments suggest that behaviors that seem developmentally constrained can, in fact, be manipulated in developmental time. That is, processes that presumably rely on experience-expectant mechanisms (e.g. motor skill) show experience-related plasticity in their developmental onset. An ERP experiment in conjunction with these types of enrichment manipulations would help the field examine neural mechanisms that support these accelerated learning experiences. For instance, one could imagine that these accelerated behavioral experiences are subserved by precocial activity in motor cortices. As neural development is hierarchical and highly linked across the brain, these early enriched experiences likely have subsequent effects on downstream neural and behavioral development. Conducting such studies in infants is particularly useful as it would help avoid some of the potential confounds of domain-general experience that older children are already equipped with.
Do Development and Learning Processes Share the Same Neural Mechanisms?
Without delving too deeply into the different types of learning processes that occur, both in development and beyond, there are undoubtedly some types of learning that are highly similar in both. For instance, learning by trial and error is one common and lifelong way that organisms, from rodents to primates, master their environment. Given their limited speaking and language comprehension abilities, infants constantly learn through trial and error; as such, they are problem-solvers who are constantly faced with a problem and the challenge of solving it. A common dilemma a young infant encounters is how to balance and sit upright. After repeated collapses and attempts at a solution, the infant eventually learns to use an arm in a so-called “tripod stance” to support him/herself. In adults, the neural activation that accompanies learning by trial and error, particularly through unexpected outcomes, is referred to as the “prediction error signal” [e.g. Hollerman and Schultz, 1998; Schultz et al., 1997], thought to be mediated primarily through the neurotransmitter dopamine. Briefly, there is an increased dopamine firing rate in nonhuman primates and increased activation in dopamine-rich region in humans when the organism receives an unexpected event [Fiorillo et al., 2003]. Eventually, the dopamine signal decreases as the organism learns to expect the event [Fiorillo et al., 2003; 2008]. These findings have implicated dopamine as a learning signal. While methodological constraints have precluded examination of the neural basis of prediction error learning in the young infant, I would argue that the dopaminergic neural mechanisms are the same. That is, dopamine neurons respond to expected and unexpected events similarly in the infant as they do in the adult. However, I would also assert that, because the system in which dopamine is acting is very different across development, that prediction error cannot be exactly the same in the infant as it is in the adult. While prediction error learning is a form of environmental adaptation across development, neural plasticity that arises from it differs. In the young infant, this plasticity will influence the basic architecture of the neural system (i.e. how the brain is going to be organized) while in the adult, this plasticity is modifying the existing architecture of the brain (i.e. reorganizing and modifying but not laying the groundwork). This dichotomy is analogous to building a house, where building a brand new house represents developmental plasticity and a house remodel represents plasticity in the mature system. The tools and mechanisms are identical, but the environment in which the change is occurring is vastly different.
The utility of “noise”
One of the greatest confounds in developmental work is the significant difference in ability or performance between children and adults. As compared with adults, who can and do implicitly draw on domain-general neural resources, the child's ability to perform any given cognitive operation is inefficient at best, as they require additional effortful, explicit, and implicit requirements to perform complex cognitive demands (e.g. response inhibition) as well as adults. For example, numerous studies have shown that even when children perform a given task as well as, or without any differences in observable behavior, as adults, they recruit distinct neural strategies. Tamm et al. [ 2002] compared the changing performance of children, adolescents, and young adults on the Go/No-Go task, a measure of inhibitory control. Despite an overall reduction in reaction time with age, younger subjects showed the same level of accuracy as adults. However, the fMRI data collected alongside the performance measures revealed that younger children demonstrated a greater level of activity within left superior and middle frontal gyri than did older children and that, conversely, older participants demonstrated an increased focal activation in the left inferior frontal gyrus relative to their younger counterparts. In a separate study of cognitive control, effective interference suppression in children was associated with prefrontal activation in the opposite hemisphere as adults while effective response inhibition was associated with activation in posterior, but not prefrontal, regions activated by adults [Bunge et al., 2002]. The authors also reported that children failed to activate a region in right ventrolateral prefrontal cortex that was recruited for overall cognitive control by adults. Similarly, a more recent study showed that children recruit distinct activation profiles from adults also differ temporally (i.e. show different time-courses of activation) across relational reasoning tasks [Crone et al., 2009]. Together, these studies provide evidence for the alternative neural strategies that immature systems often engage to support more mature behavioral demands. Despite the apparent nuisance that such extreme behavioral and neural variability introduces into the study of development, dynamic systems theory celebrates this variability [Smith and Thelan, 2003]. This noise allows investigators to examine developmental trajectories of change over the short timescales of problem-solving (i.e. because of intersubject individual differences) and/or over a longer developmental span (i.e. as when comparing children with adults).
Schlaggar et al. have elegantly demonstrated how variability can be used to gather insight into developmental versus performance-related neural activity. Using a single-word processing task, they compared neural activity in a performance-matched subgroup of children and adults taken from a larger sample [Schlaggar et al., 2002]. That is, the children and adults in the matched group did not differ in behavioral performance, making it possible to determine whether any functional activation differences were due to developmental stage or performance. They found distinct patterns of activation that were age-related, performance-related, or independent from either. As such, their data shed new light on age-related regions (regions that were more/less active in children regardless of performance) that most likely reflect effects of brain development. In a follow-up study [Brown et al., 2005], the same group reported a more thorough examination of progressive and regressive neural changes across development. Using lexical association tasks, the authors identified increases and decreases in different brain regions that varied by age, performance ability, or neither. Seventy-five percent of the regions identified as showing age-related changes (i.e. independent of performance) showed decreases in activity over age. These regions were most prominently located in medial frontal and anterior cingulate cortex, right frontal cortex medial parietal, and posterior cingulate cortex. The remaining 25% of regions that showed increases in brain activity with age, were primarily later-stage processing regions, including lateral and medial dorsal frontal cortex and left parietal cortex. This strategy, of taking advantage of developmental and behavioral variability post hoc, is precisely the approach that needs to be adopted to disentangle neural mechanisms of development- and learning-related plasticity.
The approach by Schlaggar et al. described above can easily be modified to examine training-related plasticity. By substituting a training component for the “performance” group (i.e. the group who had naturally occurring variability in performance ability), one could separate neural activation changes related to age, training, or neither. For instance, a group of individuals ranging in age from childhood to adulthood could be trained on a motor task, such as juggling, that all were naive to. Participants would be scanned before and after training. Post hoc, participants would be divided into groups based on their level of juggling skill. In this manner, neural regions would be divided in those that are age-related and training-related, thereby allowing insight into neural regions more susceptible to experience and those with greater developmental constraints.
Longitudinal training studies across development
Despite the inherent challenges, the only way to identify the root of neural plasticity as either developmental or experiential is to conduct a longitudinal training study. There is no question that the incredibly challenging, logistically difficult, and expensive nature of this type of work is what has precluded the field from embarking more vigorously on this type of research. However, a few recent studies have proven its feasibility. For instance, Durston et al. [ 2006] used a combined longitudinal and cross-sectional study to examine shifts in cortical activity during a response inhibition task. The longitudinal findings, relative to the cross-sectional data, showed attenuated activity in dorsolateral prefrontal cortical areas with age. In parallel, there was increased focal activation in ventral prefrontal cortex that was related to improvements in task performance [Durston et al., 2006]. A more recent study by Hyde et al. [ 2009] implemented a training component. Their study builds on previous studies in adult musicians and matched nonmuscians that have revealed structural and functional differences in brain regions relevant to music production [Bermudez and Zatorre, 2005; Elbert et al., 1995; Gaser and Schlaug, 2003; Pantev et al., 1998; Zatorre et al., 2007]. The authors were motivated by the question begged of this type of research: Do musicians (or others who show skill-related neural plasticity) do so because of biological predispositions to music or because of intensive music training? Hyde et al. compared structural changes in relation to behavioral changes in young children who received 15 months of instrumental musical training relative to a group of children who did not. The children who received private keyboard lessons showed greater behavioral improvements on music discrimination and related tasks than the nontrained children; neither group showed differences between baseline and testing on nonmusical tasks. In addition, the musically trained children showed greater structural changes in right precentral gyrus, corpus callosum, and the primary auditory region [Hyde et al., 2009], consistent with findings in adults [Zatorre et al., 2007 for review]. Their data provide new evidence for training-induced structural brain plasticity in early childhood. Using structural MRI, Schlaug et al. [ 2005, 2009] also identified structural differences in the corpus callosum in young musicians. Based on total weekly practice time, they divided a sample of 5- to 7-year-old children into three groups: high-practicing, low-practicing, and controls. There were no differences in corpus callosum size at baseline, but differences emerged after approximately 29 months, with the greatest increased change in the high-practicing group of children [Schlaug et al., 2009]. Further, total weekly music exposure predicted degree of change in the corpus callosum as well as improvement on a nonmusic related motor-sequencing task.
In addition, training interventions have been implemented in clinical populations and have similarly shown robust plasticity. In a group of children with ADHD, training significantly improved performance on a nontrained visuospatial working memory tasks. In addition, motor activity, as measured by the number of head movements during a computerized task, was significantly reduced in the treatment group [Klingberg et al., 2002]. In a separate study, strong improvement in attention was found after only 5 days of attention training in a group of 4- and 6-year-old children. This change was paralleled by changes in EEG patterns that resembled a more mature pattern of activation, such that training had specific effects on the scalp distribution of the ERPs that was similar to the influence of development [Rueda et al., 2005].
Several studies have provided strong support for the claim that children with reading disabilities can benefit significantly from intervention techniques; the impact of such interventions on neural plasticity has been assessed using fMRI [McCandliss and Noble, 2003]. In one study, dyslexic children received an intervention after an initial baseline scan showing the typical reduced activation of the left posterior superior temporal gyrus (STG) during a phonologically challenging task [Simos et al., 2002]. Following the 80-hour intervention, all dyslexic children showed significant increases in reading skill, as well as increased activation in the left posterior STG [Simos et al., 2002]. Similar changes in neural activation were reported in separate intervention training in children with other dyslexia [McCandliss et al., 2001; Temple, 2002]. In a recent report, Keller and Just showed that reading remediation induces changes in white matter of poor readers [Keller and Just, 2009], such that fractional anisotrophy (FA) was significantly increased following remedial instruction. This FA increase was correlated with improvement in phonological decoding ability, which demonstrates how behavioral intervention can influence neural plasticity.
A recent study was able to examine the effects of reading on structural neural change without the influence of development [Carreiras et al., 2009]. Structrual MRI scans were obtained from adult participants who had recently completed a literacy program in adulthood (before the program, they were illiterate) and matched illiterates who had not yet started the literacy program. Their findings suggest that learning to read strengthens the coupling between left and right angular gyri and between the left dorsal occipital gyrus and left supramarginal gyrus [Carreiras et al., 2009].
Collectively, these studies have suggested that plasticity within the immature brain shows similarities to plasticity in the adult system. First behavioral improvements related to intensive training or experience are associated with neural plasticity specific to the task at hand (e.g. increased activation in the STG following reading intervention). What this suggests is that experience-dependent mechanisms do not differ greatly across the lifespan. Second, neural regions previously associated with experience-expectant mechanisms, such as motor abilities and language, show a high degree of plasticity across development, suggesting that perhaps there is plasticity in processes that are initially precipitated by expectant interactions with the environment.
These studies have also led to more questions that will undoubtedly be addressed in the next generation of research on this topic. First, which neural systems show greater or less training-related plasticity earlier in development? For example, there is significantly greater plasticity in receiving and learning from language input during infancy than in any other point in life. As infants receive increasing exposure to their native language, neural systems sensitive to language lose plasticity, which is translated into more difficulty discriminating speech sounds of foreign languages and learning new languages [Doupe and Kuhl, 2008]. Are there other examples of such extreme behavioral and neural loss of plasticity across the lifespan, whereby learning itself imposes constraints on plasticity? Second, how do the timescales of neural plasticity change across development? That is, do observed behavioral and neural changes occur more or less quickly in the developing brain? Again, to borrow from the language literature, young children learn to discriminate foreign languages more quickly and more proficiently than adults [Snow, 1987]; does this accelerated timescale hold for all cognition? Last, which behaviors cannot be “sped up” by exposure earlier in development because of time-locked experience-expectant mechanisms? Certainly, pubertal constraints (as described in more detail in Blakemore et al. [2010], this issue) will impose at least some limits on plasticity associated with this maturational change.
Methodological Considerations and Potential Confounds
The dearth of studies that examine learning versus development is a consequence of the potential confounds and fairly prohibitive methodological factors inherent in this type of work. In this closing section I outline the most common issues.
Resources and attrition
Cost and attrition are two significant reasons that training and development studies are not more prevalent in the literature, despite calls for the need of this kind of work [Casey et al., 2005]. In addition to steep scanner costs at many institutions and scanning facilities, a healthy sample size of both children and adults are necessary for sufficient statistical power to detect learning versus developmental differences. Also, additional staff is needed to conduct the training portion of the study, as participants will need to visit and the lab and receive training at least weekly and in some cases daily, depending on the study. If the training aspect involves a particular still, such as music training, the staff will need to be proficient in this as well. Together, these requirements lead to a very expensive experiment.
Subject attrition also poses a potential hurdle in this type of work. Subject burden for a training and/or longitudinal study is relatively high, as participants visit the lab for multiple sessions and are required to maintain cognitive and training engagement. However, several groups have successfully conducted longitudinal studies [e.g. Durston et al., 2006, Giedd et al., 2009, and an ongoing study at UCLA] by increased attention and sensitivity to families' needs. Some useful strategies for successful subject retention are listed in Table I.
Table I. Strategies to increase subject retention
1. During the first study visit, ask parents to give the names and phone numbers of three people who know how to contact them. |
2. Contact parents by phone every 2 months for a longitudinal study that spans 1 year or longer. |
3. Send children birthday and holiday cards as well as a small gifts or gift cards for these occasions. |
4. Provide snacks and breaks at each visit. |
5. Reinforce children at each visit and evaluation with small age-appropriate toys, shirts, hats, and school supplies. |
6. Compensate parent for their time and effort at each visit. |
7. Send manuscripts and copies of posters/presentations that describe relevant findings to demonstrate the importance of the work they are participating in. |
Scanner-related anxiety
Subjects, particularly children, may exhibit scanner-related anxiety (e.g. Eatough et al., 2009]. Initial anxiety might lead to increased head motion, increased vigilance, or attention, all of which can skew the observed results and/or interpretations. As such, investigators should implement techniques to reduce the possibility of this potential confound. In addition to acclimation to the scanning environment with a mock scanner, as many groups do, it would be prudent to conduct two baseline scans in training studies. The first baseline scan, which could be conducted using an unrelated task, would capture the novelty-related anxiety while the second baseline scan would serve as the “true” baseline of the training session.
Performance differences
As elegantly reviewed in Schlaggar (this issue) and stated above, performance differences between developmental and adult populations can introduce significant confounds and make difficult the accurate interpretation of neuroimaging data. Without addressing performance differences, the observed differences in brain activity between children and adults could be misinterpreted as maturational differences in functional neuroanatomy. Successful ways to address this issue include the use passive tasks in the scanner, the use of tasks that are equated for difficulty, and performance-matching, as reviewed earlier. In addition, the dependent measure should test for accuracy (as equated task difficulty), rather than reaction time, as the latter is well known to lag in developmental populations.
A problem in the interpretation of training studies is the possibility that brain effects might arise from stress, sensory stimulation, motor activity, or other nonspecific consequences of the training procedure, rather than information acquired through training [Greenough et al., 1987]. As such, it is critical that appropriate control groups are studied in tandem.
Last, choosing the optimal time between scans is a potential dilemma, as the field simply does not have enough knowledge of the precise nature of the underlying neural events, how quickly they are affected by experience, and their impact on the imaging signal [Draganski and May, 2008]. MRI voxel-based morphometry has revealed changes within 1 week after training [Driemeyer et al., 2008]. Other groups [Draganski et al., 2006] have waited 3 months between scans, as this is the length of time needed for newly generated stem cells to differentiate into neurons [Cummings et al., 2005].
CONCLUSIONS
Neural plasticity is the brain's solution to the challenge of integrating new information into its repertoire of neural networks. Three main points have been outlined in this review. First, the reader is asked to consider how and if development differs from learning and, subsequently, how this distinction translates to differences or similarities in neural mechanisms. I have proposed that rather than struggling to parse out distinctions between two constructs that are nearly inextricable, it is important to recognize how their effects bleed into one another, and how experience-expectant and experience-dependent mechanisms simultaneously and independently influence each one.
Second, that the local neural environment in which plasticity occurs is a critical component of change. While the actual neural mechanisms (e.g. synaptic mechanisms) that underlie plasticity probably do not differ significantly in the immature and mature system, the local environment in which that plasticity is occurring is undoubtedly different. This difference exists not only in terms of the cellular, anatomical, and metabolic environment, but in the much larger contextual environment of the individual. For instance, modifying synapses that are already committed (e.g. learning a motor skill such as juggling) is very different than committing the synapse for the first time (e.g. learning the motor coordination necessary for the first time a baby holds himself up).
Last, the challenges of this type of work have precluded significant advances in addressing plasticity-related questions. Despite these hurdles, it is critical that the field more vigorously embrace longitudinal training studies in developmental groups if we are truly committed to uncovering the mechanisms underlying plasticity across development. The greatest utility of cross-sectional studies of development is the insight into developmental differences. However, to understand developmental changes in the brain, a longitudinal approach is critical. Eventually, answers to these questions will be useful in identifying strategies and developmental timing for optimal learning, remediation, and rescue from brain injury.