Abstract
__Background__. Childhood maltreatment (CM) and exposure to community violence (ETV) are correlated with physical/mental health and psychosocial problems. Typically, CM and ETV are examined separately, by subtypes within category, or collapsed across both into one category of adversity. Consequently, research is limited in identifying subgroups of individuals with different amounts of exposure to both CM and ETV. Accordingly, we lack sufficient understanding of the extent to which problems associated with CM and ETV vary based on the amount (i.e. dose) of exposure to both of these experiences. __Methods__ We used 20 samples (28,300 individuals) to estimate person-centered profiles of CM and ETV occurrence and co-occurrence within each sample. An individual data multilevel meta-analytic framework was used to determine the average effect size across samples for different profiles and conditional probability correlations within sociodemographic, neighborhood, health, mental health, and psychosocial domains. __Results__ The profile characterized by high levels of CM and high levels of ETV correlated with stressful life events, depression and anxiety symptoms, and general indicators of externalizing behaviors. CM predominant profiles were associated with mental health diagnoses and treatment. ETV predominant profiles associated with risk-taking/violent behavior and neighborhood-level disadvantage. However, nuance based on the dose of CM or ETV was evident. __Conclusions__ It is important to identify subgroups based on the amount of exposure to CM and ETV. These subgroups have differential relationships with correlates across domains. Greater delineation and description of the lived experience will allow for more precision in addressing the burden of childhood adversity.
Childhood adversity carries substantial societal and personal burdens that persist through the lifespan, taking a toll on individuals, families, and communities. Two well-studied types of childhood adversity are childhood maltreatment (CM; physical, emotional, or sexual abuse or neglect, occurring in the home or committed by a caregiver; Finkelhor, Turner, Shattuck, & Hamby, 2015) and exposure to community violence (ETV; witnessing or being the victim of acts such as assaults, shootings, and robberies, as well as hearing gunfire outside of the home; DeCou & Lynch, 2017). Those who experience CM and ETV often lead lives of inter- and intrapersonal dysfunction across academic (Crozier & Barth, 2005; Henrich, Schwab-Stone, Fanti, Jones, & Ruchkin, 2004), physical health (Fairbrook, 2013; Wegman & Stetler, 2009), mental health (Margolin & Gordis, 2000), and psychosocial (e.g. aggression, delinquency, low self-esteem; Braga, Gonçalves, Basto-Pereira, & Maia, 2017; Cicchetti & Toth, 2005; Jonson-Reid, 1998) domains.
Generally, research documents similarities in correlates of CM and ETV. However, a closer examination of these relationships highlights meaningful differences. For example, in the mental health domain, meta-analyses find moderate to large associations between specific forms of CM and internalizing symptoms (Infurna et al., 2016) but only a small association between ETV and internalizing (Fowler, Tompsett, Braciszewski, Jacques-Tiura, & Baltes, 2009). In a study by Cecil, Viding, Barker, Guiney, and McCrory (2014) that directly compares the impact of CM and ETV on adolescent mental health, CM is associated with internalizing, externalizing, and trauma-related symptoms, but ETV is associated only with externalizing and trauma. In the psychosocial domain, ETV is associated strongly with aggression, but CM is linked only modestly with aggression (Baskin & Sommers, 2014; Fitton, Yu, & Fazel, 2020). Furthermore, Stevens and Mennen (2018) find that ETV predicts aggressive behavior, but only for youth who do not experience physical abuse by a caregiver. However, due to methodological limitations in the assessment and analysis of CM and ETV, we still do not understand, fully, which types and dose (i.e. amount) of adversity are associated with correlates across domains.
Most often, researchers take one of two approaches in assessing CM and ETV. Some explore these experiences separately (e.g. Cicchetti, Rogosch, & Thibodeau, 2012; Gaylord-Harden, Bai, & Simic, 2017). Others evaluate CM and ETV simultaneously but then collapse them into one metric of adversity (e.g. polyvictimization, adverse childhood experiences [ACEs], dimensional model of adversity and psychopathology; Felitti et al. 1998; Finkelhor, Ormrod, & Turner, 2007; McLaughlin, Sheridan, & Lambert, 2014). However, these approaches obscure the possible co-occurrence of CM and ETV. Additionally, they assume an equivalence in various forms of adversity, including CM, ETV, parental divorce, and parental incarceration. Although some research finds that adversity total score (i.e. sum across various forms) predicts greater dysfunction (Felitti et al., 1998), recent evidence using the ACE framework suggests that specific types of adversity differentially relate to correlates and that considering the type of adversity accounts for more variance in correlates than total score approaches (Henry et al., 2021; Negriff, 2020). Furthermore, most research examines CM and ETV primarily using variable-centered statistics (Morin, Bujacz, & Gagné, 2018), which generate a single set of parameters that characterizes the whole population homogeneously (Howard & Hoffman, 2018) in terms of their CM and ETV experiences. Consequently, little is known about the existence and prevalence of subgroups of individuals who experience similar doses of CM and/or ETV, the dose of exposure needed to produce adverse effects, and which correlates apply to CM and/or ETV. Thus, two open questions in the adversity literature are: (1) what are common subgroups of CM and ETV? and (2) how do these subgroups differentially associate with correlates across domains?
To answer these questions, we:
(1) perform person-centered analyses to identify the various subgroups of CM and ETV occurrence and co-occurrence (i.e. quantifying subgroups) and
(2) implement a meta-analytic framework to characterize the types and strengths of conditional relationships among CM, ETV, and relevant correlates across several domains (i.e. quantifying the relationship between CM/ETV dose and correlates).
Although it is more common for meta-analyses to rely on effect sizes gathered from published studies, meta-analyses with individual data allow researchers to create variables and establish relationships needed to address open questions (see Blettner, Sauerbrei, Schlehofer, Scheuchenpflug, & Friedenreich, 1999). This approach avoids the pitfalls of relying on any single result/ sample and synthesizes existing data in order to clarify the nature of the relationships among CM, ETV, and correlates across a variety of individuals. In 20 different samples, we implemented separate latent profile analysis to identify subgroups of CM and ETV co-variance. Then, within each sample, we examined the probability of specific profile memberships relating to sociodemographic, neighborhood, health, mental health, and psychosocial correlates that were identified by previous research as related to CM and ETV. Finally, we utilized a multilevel meta-analytic approach across the 20 samples to quantitatively estimate the average association between CM and ETV profiles and correlates.
Method
Records
Data were gathered from sources identified from August 2018 to December 2018. In total, 2988 records were identified. Documentation for each record was reviewed to determine inclusion and exclusion (see Fig. 1a for PRISMA flowchart; Supplemental Methods for search terms, a full list of records, and inclusion/exclusion criteria).
Definitions and key variables
There were several ways to conceptualize CM/ETV, whether in terms of subtypes of exposure (e.g. physical abuse only for CM or witnessing violence only for ETV), underlying dimensions (e.g. deprivation for CM-neglect or threat for CM-physical abuse and ETV), or broader definitions that included all subtypes within CM (physical, emotional, and sexual abuse and neglect in the home/by a caregiver) and within ETV (victimization/witnessing of violence in one’s community). We followed the broad definitions of CM and ETV to draw comparisons with the greatest amount of previous research and to maximize the use of various types of assessments available across datasets (Table 1). Correlates were measured when each study was conducted whereas CM and ETV were assessed retrospectively for all studies. Variables from each of the 20 samples were extracted, as available, to examine the associations among CM/ETV profiles and relevant correlates (see Supplemental Methods for details on correlates and harmonization; online Supplementary Tables 2A–2E for summary statistics, original codebook variable names, transformations, and variable type of correlates).
Creating samples
After inclusion criteria were satisfied, 14 records remained. The majority of records (eight) were cross-sectional in nature. For the six longitudinal records, only one assessment wave was used for analyses so that no participant was represented more than once across samples. These records spanned different developmental stages. Researchers have emphasized the importance of taking a developmental perspective when examining CM/ETV (Margolin & Gordis, 2000) as the timing of exposure may impact how the individual experiences it and the relationship to correlates (Gee & Casey, 2015). Therefore, for follow-up analyses, the 14 records were split by developmental stage.3 Individuals ages 0–12 were considered children (n = 7); individuals 13–17 adolescents (n = 8); and individuals 18 and older, adults (n = 5). This yielded 20 samples (see Fig. 2 for demographics summaries).
Data analyses
What are common subgroups?: Within samples latent profile analyses Latent profile analyses were used to find subgroups of individuals based on their levels of both CM and ETV (see Fig. 1b for analytic flow). Analyses were conducted in Mplus 7.11 on each of the 20 samples using a maximum likelihood robust estimator to account for missingness (Muthén & Muthén, 2013). Across the 20 samples, 18 contained more than 250 participants, indicating that, in general, samples were sufficiently large for latent profile analysis (Williams & Kibowski, 2016). Two indicators, CM and ETV scores, were z-scored and subjected to latent profile analysis.
We evaluated 2- through 6-class models (see online Supplementary Tables 1A–1T with optimal model solution bolded). Model fit was compared using the Bayesian information criterion, where smaller values indicated a better model fit (Schwarz, 1978); entropy, where higher values indicated a better ability to classify participants and discriminate among classes (Ramaswamy, DeSarbo, Reibstein, & Robinson, 1993); and the Lo–Mendell–Rubin-adjusted likelihood ratio test (Lo, Mendell, & Rubin, 2001), where a significant p value indicated that a model with k number of profiles was preferred over a model with k -1 profiles. Profile size also was considered to assess the representativeness of the profile (Nylund, Asparouhov, & Muthén, 2007). correlates using Pearson or Spearman correlations in R (R Core Team, 2013). Differences in the magnitude of pairs of associations between correlates and the probability of membership in each latent profile were evaluated using a test of dependent correlations (Lee & Preacher, 2013). Tests of dependent correlations were needed to determine if the correlation coefficients, which were derived using a shared variable, significantly differed from each other. Conditional probabilities were used to reduce the impact of unbalanced sample sizes across latent profiles, and Bonferroni correction accounted for the number of pairwise comparisons performed on each correlate. Casewise deletion addressed missingness.
How do subgroups associate with correlates?: Across samples multilevel meta-analysis
Multilevel meta-analysis was used to understand the aggregated relationships among profile membership and correlates across samples. The effect sizes (i.e. correlation coefficients), standard errors of the effect sizes, and sampling variance of the effect sizes were estimated using a Fisher z-transformation, resulting in a normalized distribution of correlation coefficients, facilitating their comparison across samples (Lipsey & Wilson, 2001). A multilevel meta-analysis approach was used to account for the hierarchical structure of data, where effect sizes were nested within profiles and samples (Van den Noortgate, López-López, Marín-Martínez, & Sánchez-Meca, 2013). Specifically, a 3-level random-effects model run with a restricted maximum likelihood estimate (Assink & Wibbelink, 2016) was used where the profile was included as the random effect (level 1: sampling variance for each effect size, level 2: variance within samples, and level 3: variance among profiles nested within samples). This model was advantageous because it allowed for an estimate of the pooled correlation for each profile and a test for differences in correlations between profiles. It also accounted for the fact that the data stemmed from different sources and that not all profiles were represented in all samples.
We used standard fit analyses to determine if there was significant heterogeneity in the distribution of effect sizes (Supplemental Methods) and followed-up accordingly with analyses that considered profile as a moderator. We examined the omnibus test of the model parameters (test of moderators) to determine whether the overall model effect was significantly moderated by profile type and subsequently examined individual profile estimates and confidence intervals (CIs). The Knapp and Hartung adjustment (Knapp & Hartung, 2003) was applied to reduce the likelihood of falsely significant events. Estimates were assessed for deviance from the reference group (Lowered CM/Lowered ETV profile). Then, we conducted pairwise post-hoc analyses of variance (ANOVAs) that compared any profile estimates which deviated from the reference group to each other. Bonferroni correction that considered the total number of correlates and the number of pairwise profile comparisons was used to account for the number of post-hoc comparisons performed per correlate. Analyses were conducted using the metafor package in R (Viechtbauer, 2010).
As follow-up to the profile analyses, we examined mean age of the sample as an additional moderator of the profile effects for each correlate that showed significant heterogeneity in the profile moderated meta-analysis (Table 2; online Supplementary Table 5). We examined the correlations between the likelihood of profile membership and age by sample in order to reduce the likelihood of overfitting due to multicollinearity (Harrer Cuijpers, Furukawa, & Ebert, 2019). The majority of samples showed small, non-significant correlations among profile membership and age. Therefore, the mean age for each sample (continuous variable) was included as a second moderator in follow-up meta-analyses. Bonferroni correction accounted for the number of correlates in the follow-up age analyses.
Results
What are common subgroups?: Latent profile analysis
Profiles were labeled as: High if CM/ETV estimates were at least 1 standard deviation (S.D.) above the sample average and significantly different from the sample average; Elevated if CM/ETV estimates were less than 1 S.D. above the sample average and significantly different from the sample average; Average if CM/ETV estimates were not significantly different from the sample average; and Lowered if CM/ETV estimates were significantly below the sample average (see Table 1 for absolute CM/ ETV amounts).
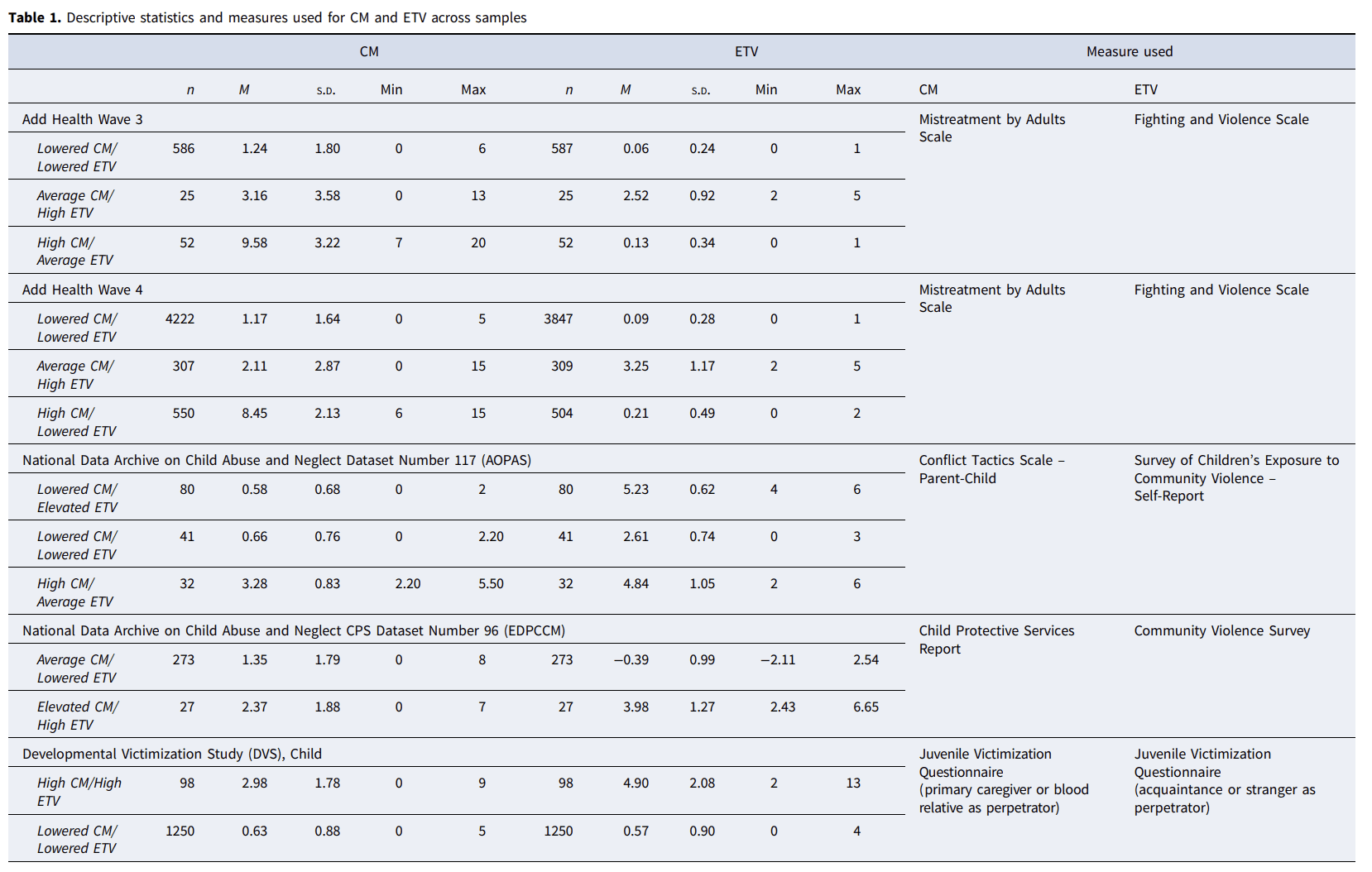
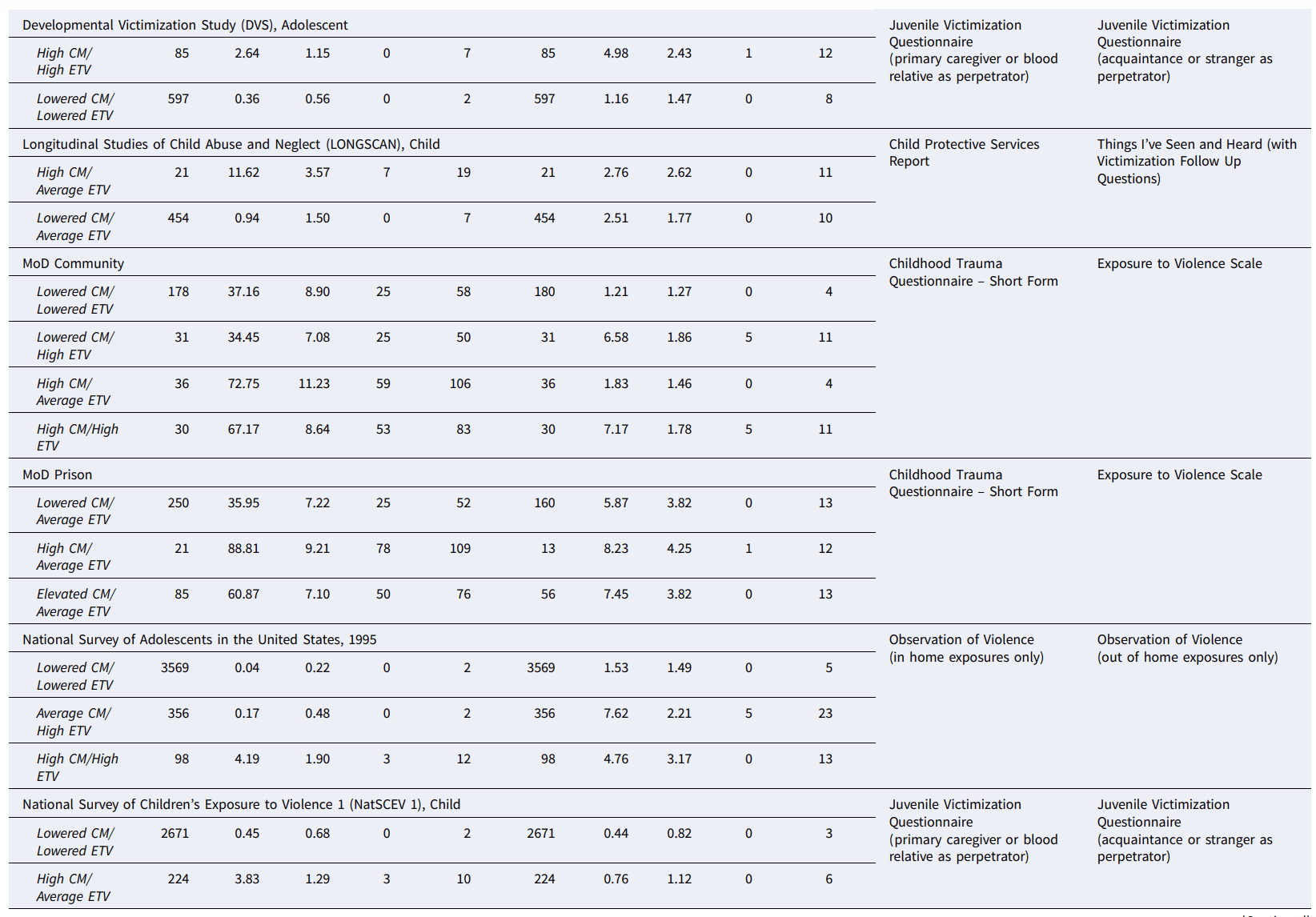
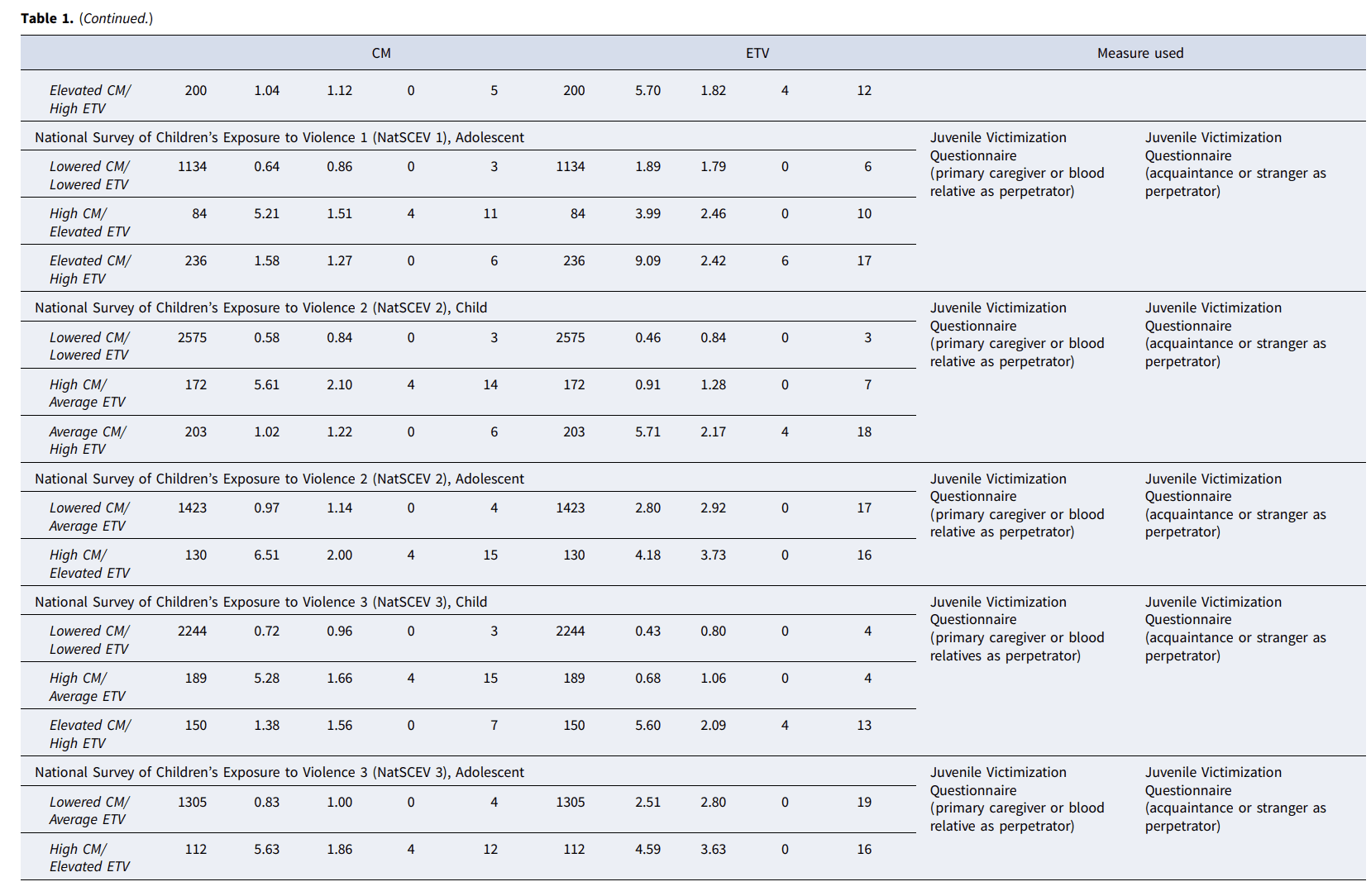
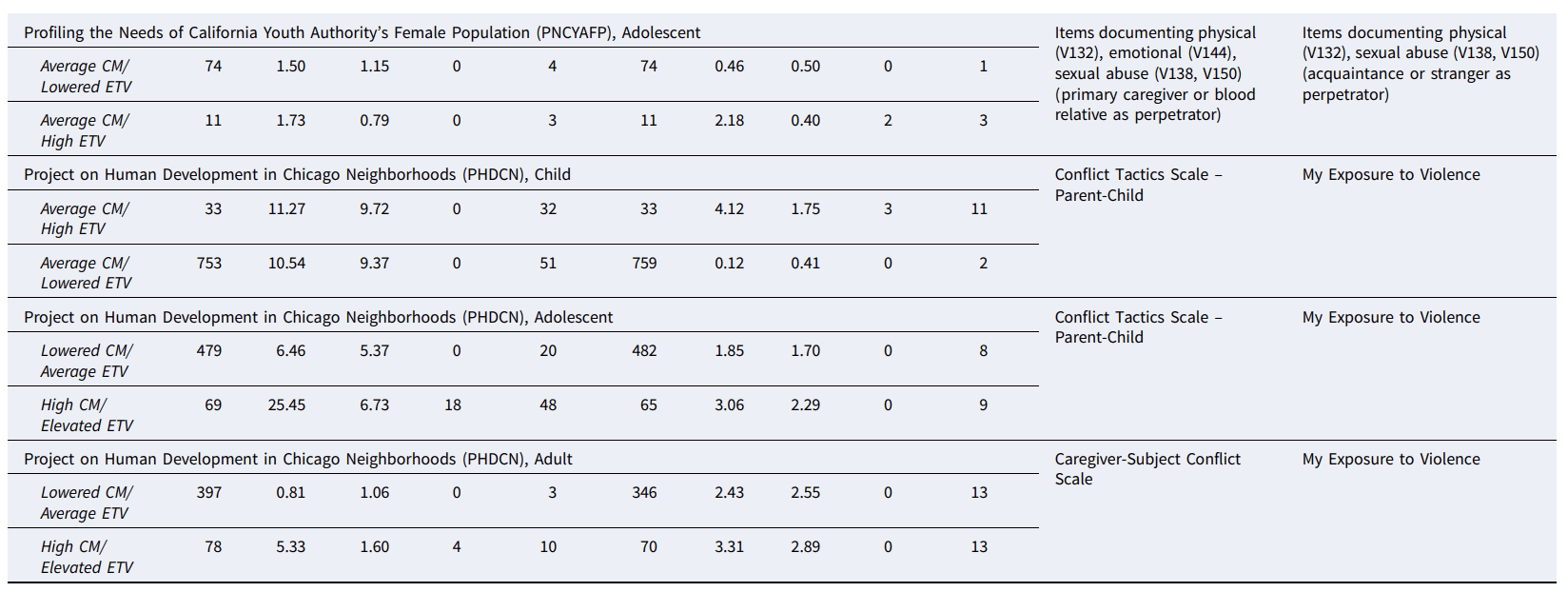
How do subgroups associate with correlates?: Correlates and meta-analysis
The relationships among profiles and correlates by sample are presented in online Supplementary Tables 3A–3T and Figures 2A–2E. These effects provided the foundation for the meta-analyses. Table 2 presents the meta-analytic results.
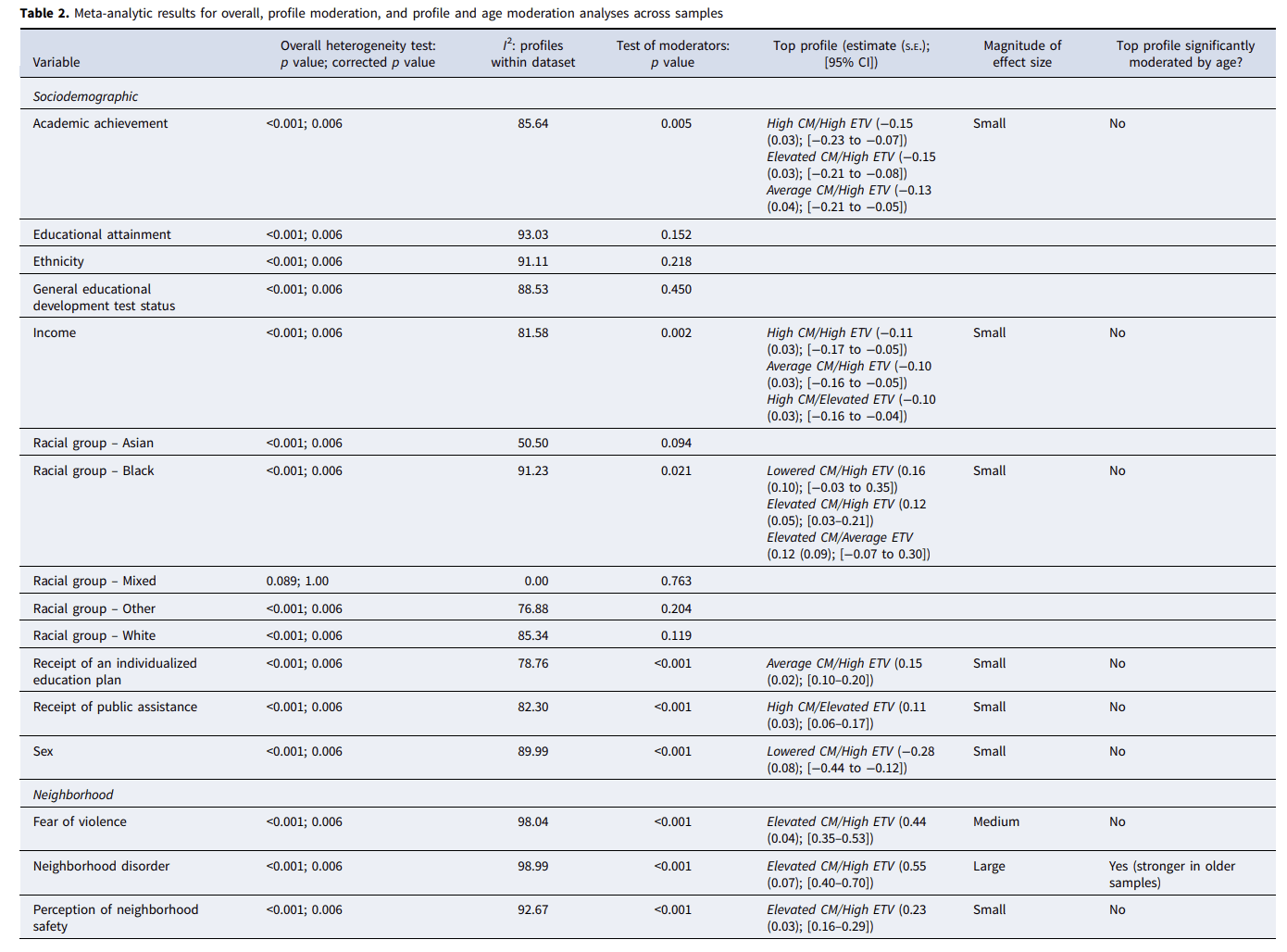
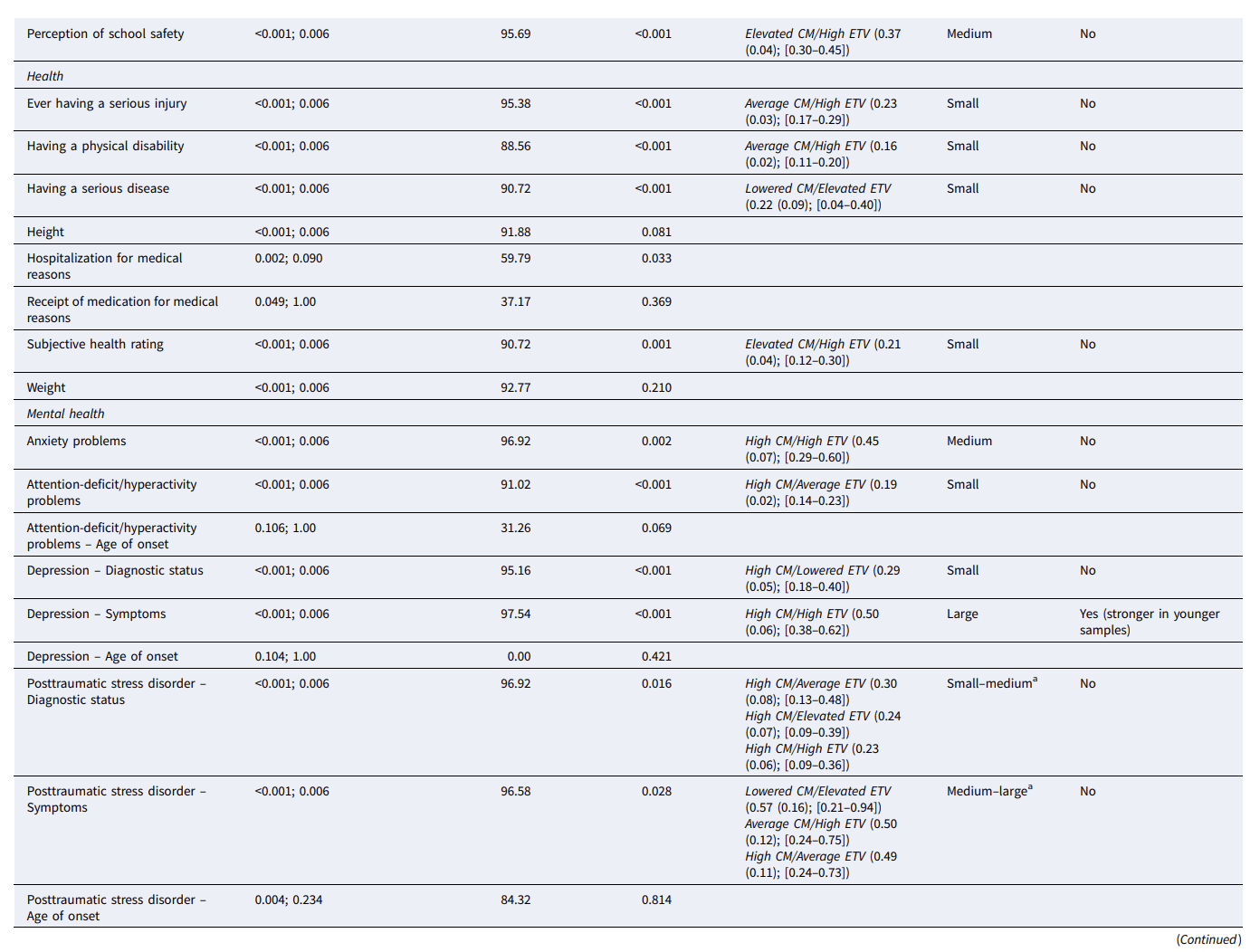
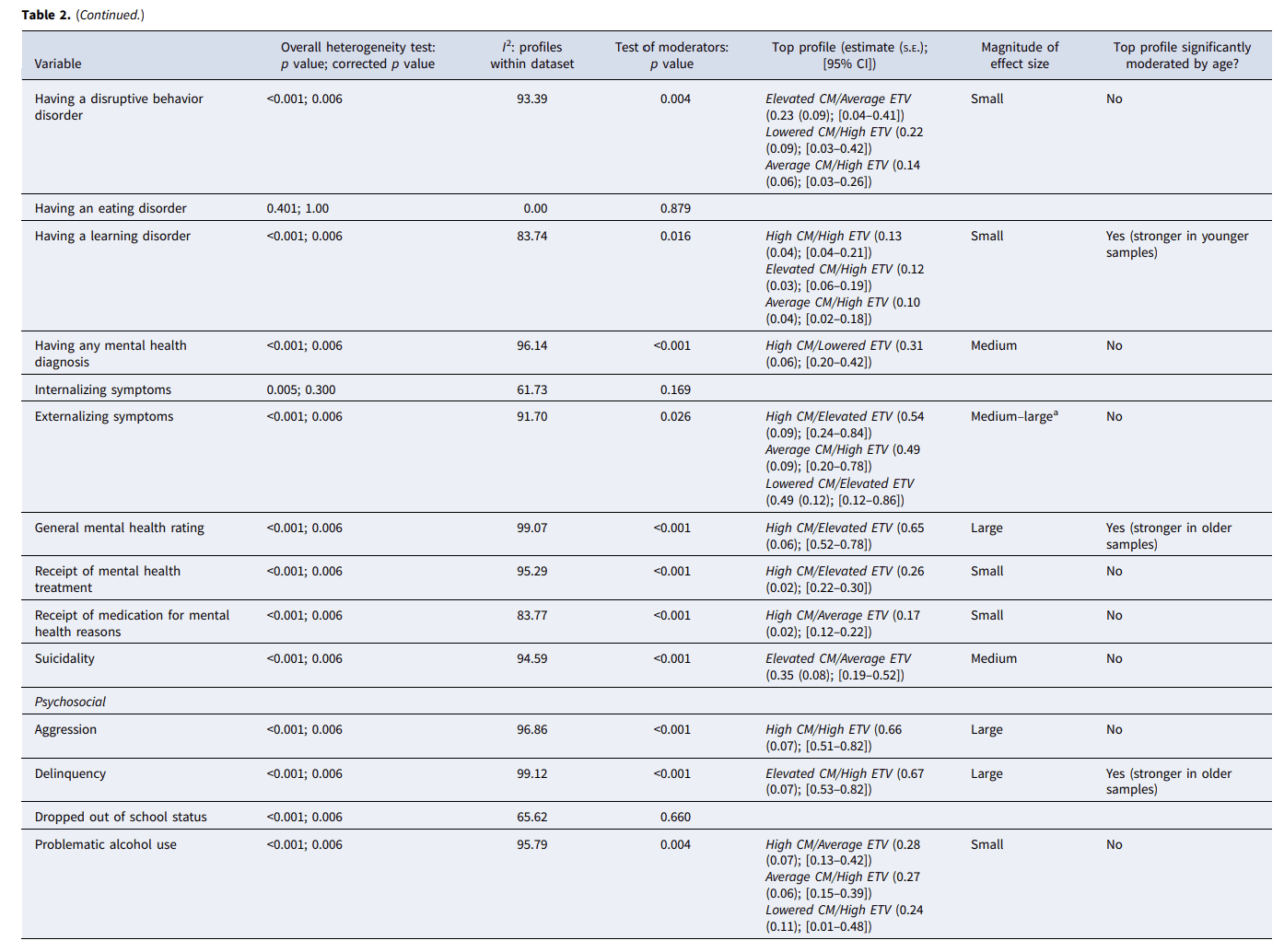
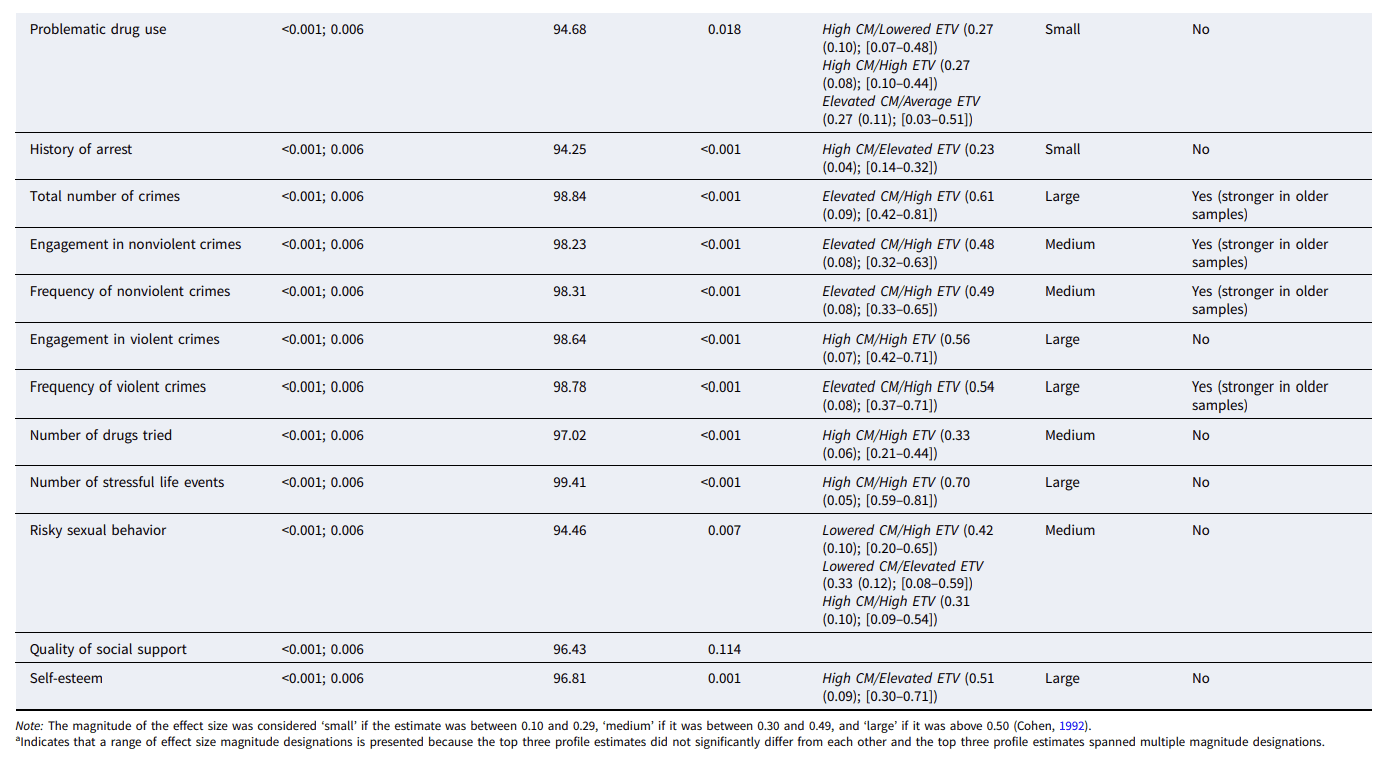
Of the 60 correlates subjected to meta-analyses, 42 demonstrated significant heterogeneity and significance on the test of moderators when profile was added (Fig. 3).
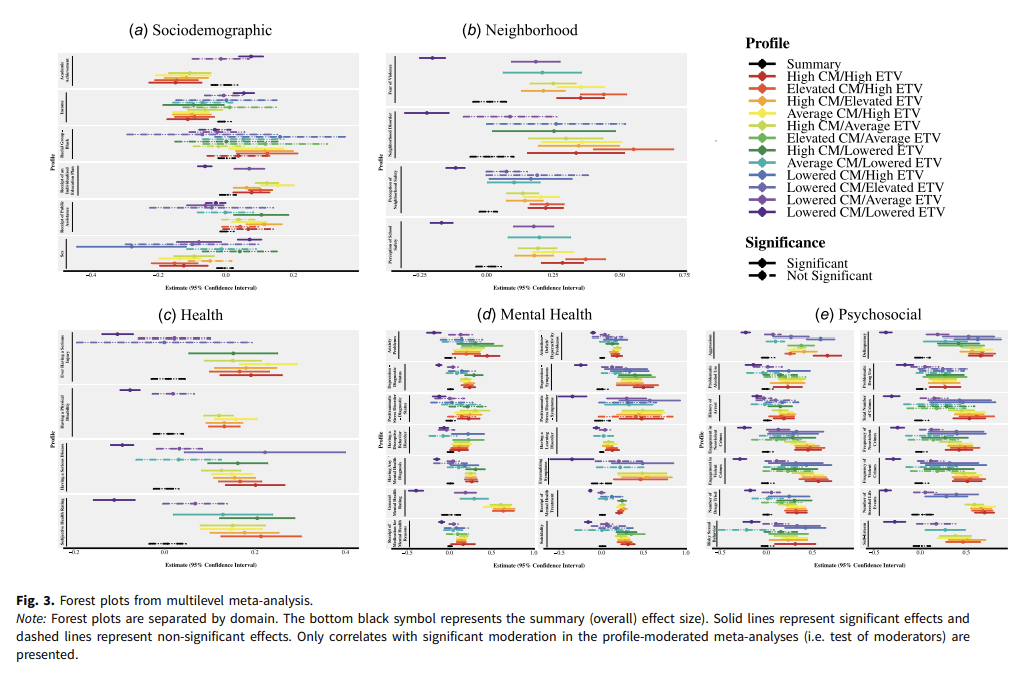
For many correlates, the estimate for a single, predominant, profile emerged as significantly different from estimates for all other profiles following the post-hoc ANOVAs. In these instances, the profile with the largest estimate for a particular correlate was interpreted. Broadly, individuals in the High CM/High ETV profile reported a wide range of problems, although most correlates did not reflect severe expressions of symptomatology or behavior. Specifically, the High CM/High ETV profile was related to increased anxiety problems (ES = 0.45) and depression symptoms (ES = 0.50), higher rates of aggression (ES = 0.66), engagement in violent crimes (ES = 0.56), more substances tried (ES = 0.33), and more stressful life events (ES = 0.70). Several effects were predominated by the presence of higher levels of CM in the profile. Generally, these profiles showed the strongest effects with mental health diagnoses, treatment, and related correlates. Specifically, individuals in the High CM/ Lowered ETV profile reported increased rates of depression diagnosis (ES = 0.29) and having any mental health diagnosis (ES = 0.31). Individuals in the High CM/Elevated ETV profile had increased rates of public assistance (ES = 0.11), receipt of mental health treatment (ES = 0.26), worse general mental health (ES = 0.65), higher likelihood of arrest (ES = 0.23), and poorer self-esteem (ES = 0.51). Individuals in the High CM/ Average ETV profile reported higher rates of attention-deficit/ hyperactivity disorder (ADHD) problems (ES = 0.19) and receipt of medication for mental health reasons (ES = 0.17). The Elevated CM/Average ETV profile was correlated with increased suicidality (ES = 0.35).
Finally, several effects were predominated by the presence of higher levels of ETV in the profile. These profiles were most robustly related to neighborhood-level correlates, health problems, and indicators of antisociality. Individuals in the Lowered CM/ High ETV profile were more likely to be male (ES = −0.28). Individuals in the Lowered CM/Elevated ETV profile had higher rates of serious disease (ES = 0.22). The Elevated CM/High ETV profile reported higher fear of violence (ES = 0.44), neighborhood disorder (ES = 0.55), lower perceptions of neighborhood (ES = 0.23) and school safety (ES = 0.37), and lower subjective health4 (ES = 0.21). Additionally, this profile had greater delinquency (ES = 0.67), total number of crimes (ES = 0.61), engagement in (ES = 0.48) and frequency of nonviolent crimes (ES = 0.49), and frequency of violent crimes (ES = 0.54). The Average CM/High ETV profile was associated with an increased receipt of an individualized education plan (IEP; ES = 0.15), higher rates of serious injury (ES = 0.23), and physical disability (ES = 0.16).
For some correlates, there was not an estimate for a single profile that was significantly different from estimates for other profiles in the post-hoc ANOVAs. Although there was a significant profile moderation effect, one profile, compared to others, did not show a statistically stronger relationship to the correlate. Here, we inspected the profiles with the largest three effects sizes to determine if a particular pattern descriptively emerged among the largest effects.
For example, lower household income and greater externalizing symptoms were related to profiles containing High CM and/ or High ETV, suggesting that CM and ETV jointly drive the relationships but neither experience consistently accounts for the relationship between particular profiles and these correlates. By contrast, greater rates of PTSD diagnosis and problematic drug use appeared determined by higher levels of CM (i.e. largest estimates for these correlates included High or Elevated CM but anywhere from Lowered to High ETV). Finally, following from the patterns above, correlates where ETV appeared to drive the relationship between profiles and correlates largely reflected sociodemographic differences and antisociality. Specifically, profiles with higher levels of ETV, and any level of CM from Lowered to High, were related to poorer academic achievement, Black racial group membership, increased PTSD symptoms, higher rates of disruptive behavior disorder, learning disorder, greater problematic alcohol use, and more risky sexual behavior.5
Meta-analysis age moderation
Age moderation results presented here are only for the correlates where a single, predominant, profile accounted for the largest effect (see online Supplementary Table 5 for full results). Younger individuals in the High CM/High ETV profile reported higher depression symptoms (ES = −0.13) and greater likelihood of having a learning disorder (ES = −0.25). Older individuals in the High CM/Elevated ETV profile had poorer general mental health ratings (ES = 1.57). Finally, older individuals in the Elevated CM/ High ETV profile had larger effects for neighborhood disorder (ES = 0.39), delinquency (ES = 0.46), total number of crimes (ES = 0.39), engagement in (ES = 0.36) and frequency of nonviolent crimes (ES = 0.42), and frequency of violent crimes (ES = 0.35).
Discussion
Research on CM and ETV highlights the substantial and long-term impact of these experiences. Nonetheless, limited research has identified subgroups of individuals based on distinctive experiences CM and/or ETV. Moreover, research is fairly piecemeal, making it difficult to reliably estimate and synthesize the impact of CM/ETV on functioning across domains. The present study addresses these limitations.
What are common subgroups?
Profile analyses within samples reveal that approximately 27% of individuals experience some (Average or higher) CM and/or ETV and 13% experience Elevated or High levels of CM and/or ETV. The variability in the types of profiles and in the number of people within these profiles emphasizes the need for research and clinical protocols to systematically assess an individual’s combination of CM and ETV and the relationship of the combination to relevant correlates.
How do subgroups associate with correlates?
Much research on adversity finds evidence of a dose–response relationship between the types of adversity and dysfunction (e.g. Chapman et al., 2004). However, our results suggest that individuals with High levels of both CM and ETV may show mild-moderate dysfunction across domains when compared to individuals with High levels of one experience but Elevated levels of the other. For example, in the mental health domain, the High CM/High ETV profile is associated with anxiety problems and depression symptoms. Notably, though, a linear dose–response is not present, as this profile is not the most strongly related to formal mental health diagnoses. In the psychosocial domain, this profile reflects some involvement in aggressive or violent behavior and experimentation with multiple substances, but again, not at the most severe forms (e.g. frequency of violent crime or problematic drug/alcohol use). Although most of the correlates appear to be less severe for this profile, the sheer exposure to High levels of CM and ETV establishes a chronic state of stress, subclinical distress, and engagement in externalizing behaviors (Cicchetti & Handley, 2019).
Examination of profiles where CM or ETV predominated and varied in dose indicates that certain subgroups show stronger relationships with more severe correlates across domains. For profiles predominated by High CM but Lowered, Average, or Elevated ETV, mental health issues (e.g. ADHD problems, depression and PTSD diagnosis, mental health diagnoses, mental health treatment and medication, as well as general mental health) are omnipresent. Furthermore, the presence of High CM may increase contact with systems of care (e.g. child protective services), opening an opportunity for diagnostic evaluation. In terms of specific diagnoses, research suggests that maltreatment perpetrated by a caregiver, in particular, may lead to a personalization of shame and/or guilt (Sachs-Ericsson, Verona, Joiner, & Preacher, 2006), spurring internalizing symptomatology that reaches a clinical threshold. Notably, in older individuals, poor general mental health strongly associates with High CM Elevated ETV, possibly reflecting the enduring negative consequences of this combination of experiences.
Although mental health issues are most prevalent for those with higher levels of CM, adding some experience of ETV also relates to receiving public assistance, being arrested, poor self esteem, and problematic drug use. Consistent with prior research, ETV contributes yet another layer of adversity that oftentimes is associated with assaults on general mental health and self-esteem (Isomaa, Väänänen, Fröjd, Kaltiala-Heino, & Marttunen, 2013) and occurs disproportionately among lower income and justice-involved individuals (Sharkey, 2018). Overall, the strength of the relationship between CM and mental health symptomology suggests that individuals who experience High CM in the presence of moderate levels of ETV would benefit from interventions targeting mental health-related dysfunction, which also may help to support social and role functioning.
For profiles predominated by higher levels of ETV, we find relationships with specific sociodemographic characteristics (e.g. male, Black racial group), neighborhood dysfunction, and poor physical health, as well as the psychosocial correlates of delinquency and criminal offending. The High ETV profiles show robust relationships with neighborhood-level dysfunction, engagement in risky (e.g. risky sex, problematic alcohol use, and delinquency), criminal behavior (e.g. number and frequency of crimes), and disruptive behavior disorder. Prior research shows that ETV is a mechanism through which neighborhood-level dysfunction is transmitted and, for that matter, appears to potentiate the effects of such dysfunction on involvement in antisocial behavior (Baskin & Sommers, 2014), related psychopathology (McCabe, Hough, Yeh, Lucchini, & Hazen, 2005), and academic difficulties (e.g. poor academic achievement, receipt of an IEP, learning disorder – which was stronger for younger, school-aged individuals; Overstreet, 2000). Importantly, certain individuals, namely those who identify as Black, are more likely to be isolated in neighborhoods rife with ETV than any other racial or ethnic group (Rothstein, 2017; Sampson, Morenoff, & Gannon-Rowley, 2002), representing the racial inequality characteristic of the United States.
Given an abundance of research suggesting ETV is particularly relevant for neighborhood-level dysfunction and antisocial behavior, then what role does CM play in this profile? Research demonstrates strong relationships among neighborhood dysfunction, ETV, CM, and antisocial behavior. But, it appears that CM follows indicators of neighborhood distress, including ETV (Coulton, Richter, Korbin, Crampton, & Spilsbury, 2018), and therefore may not be the driver of these adverse outcomes. Additionally, although CM may not drive outcomes, it still may reinforce and extend exemplars that are supportive of antisocial behavior (Patterson, Reid, & Dishion, 1992). The finding that these patterns are stronger in older samples from distressed neighborhoods suggests that High ETV/Average or Elevated CM individuals represent groups that become ensnared in an antisocial trajectory (Moffitt, 1993).
Individuals with higher levels of ETV also experience more problems with physical health (injury, disability, and subjective health [Average or Elevated CM/High ETV]; disease [Lowered CM/Elevated ETV]) and trauma symptomatology. In part, being a victim of ETV and CM increases one’s chances of being injured and suffering from a disability. But, ETV’s effect on health and trauma symptomatology also can be understood by extending the cumulative stress model (McEwen, 2013). An outgrowth of this model suggests that more ETV and some CM puts individuals at risk for negative physiological reactions, including disease and many of the symptoms associated with post-traumatic stress. This may be the result of an increase in allostatic load which negatively impacts a variety of bodily functions, possibly contributing to the large effect size found for perceptions of poor health. Thus, although neighborhood-level interventions, such as anti-violence campaigns, school safety programs, and community policing programs attempt to ameliorate the effects of ETV, they are successful only partially due to their failure to incorporate individual-level factors that also correlate with ETV.
Limitations and conclusions
Several limitations should be noted. First, CM and ETV were measured differently across samples, precluding systematic examination of subtypes of experiences within the CM/ETV constructs (e.g. threat v. deprivation; McLaughlin et al., 2014; cf., Henry et al., 2021). Second, measures of key variables were not standard across samples (e.g. self-report v. official report) and the time period of assessment varied (e.g. retrospective v. concurrent, three adult samples assessing ETV within the past 12 months v. before age 18). The impact of these methodological differences could not be examined due to a risk of creating sub-samples with too few observations and adding comparisons that could inflate error (but see Supplemental Methods on harmonization). However, profile types were replicated across samples, correlates showed face and concurrent validity from previous studies, and correlation directionality followed patterns consistent with previous literature. Third, analyses were cross-sectional, resulting in an inability to establish temporal precedence among CM, ETV, and correlates. Longitudinal studies are needed to understand temporality in the relationships among these constructs. Finally, we used the mean sample age as our measure of developmental stage, but this measure is limited in that it collapses across all participants in the sample. More sensitive consideration of developmental stage may reveal a clearer understanding of the impact of development on the relationships among CM, ETV, and correlates.
Despite these limitations, we show that the dose of CM and/or ETV matters for the presence and strength of effects related to correlates and that different correlates co-segregate with predominant-CM compared to predominant-ETV. Broadly, experiencing High levels of CM/ETV relates to wide-ranging but not necessarily severe dysfunction. High CM, by itself, is most strongly related to mental health, primarily internalizing, diagnoses. However, the variety of mental health problems increases with the addition of some level of ETV. ETV, and to some extent the additional presence of CM, most strongly relates to neighborhood dysfunction, health problems, and antisociality. The current study highlights the importance of considering the subgroups of individuals based on CM and ETV levels and comprehensive comparison of the relationships among subgroups and correlates across domains. Effect sizes generated by the individual data meta-analytic approach could lay an important foundation for the advancement of theoretical models that consider types of adversity and the development of actuarial assessments for use in research and clinical settings.