Abstract
Background Individual differences in reward-processing are central to heightened risk-taking behaviors during adolescence, but there is inconsistent evidence for the relationship between risk-taking phenotypes and the neural substrates of these behaviors. Methods Here, we identify latent features of reward in an attempt to provide a unifying framework linking together aspects of the brain and behavior during early aneadolescence using a multivariate pattern learning approach. Data (N=8,295; nMale=4,190; nFemale=4,105) were acquired as part of the Adolescent Brain and Cognitive Development (ABCD) and included neuroimaging (regional neural activity responses during reward anticipation) and behavioral (e.g., impulsivity measures, delay discounting) variables. Results We revealed a single latent dimension of reward driven by shared covariation between striatal, thalamic and anterior cingulate responses during reward anticipation, negative urgency and delay discounting behaviors. Expression of these latent features differed among adolescents with attention deficit hyperactivity disorder (ADHD) and Disruptive Behavior Disorder (DBD), relative to those without, and higher expression of these latent features was negatively associated with multiple dimensions of executive function and cognition. Conclusions These results suggest that cross-domain patterns of anticipatory reward processing linked to negative features of impulsivity exist in both the brain and in behavior during early adolescence, and that these are representative of two commonly diagnosed reward-related psychiatric disorders, ADHD and DBD. They further provide an explicit baseline from which multivariate developmental trajectories of reward processes may be tracked in later waves of ABCD and other developmental cohorts.
Introduction
Alterations in reward-processing are core to the presentation of a wide range of psychiatric disorders and are theorized to play a central role in risk-seeking behaviors during development (1-4). Reward-processing itself is a complex, multi-faceted construct that may be assessed in humans across behavioral, clinical and neurobiological domains (5). Such cross-domain assessment is central to many predominant research initiatives which seeks to link dimensional constructs (e.g., reward sensitivity) across multiple levels of analyses and diagnoses (5-8). Despite this, the relationship between many commonly used measures of impulsivity and reward is not well established and integrated frameworks are seldomly used (9-11). In addition, developmental findings attempting to relate risk-seeking behaviors to brain function have been inconsistent and at times divergent. Prior work suggests that these inconsistencies may arise from methodological differences and heterogeneity in risk profile definitions across studies (12-14), but it is not yet known whether they may also be due to the use of traditional univariate analyses as opposed to multivariate alternatives (14), which are often only possible in large datasets.
Anticipatory reward processing occurs immediately before response selection and is an important dimension of risk decision-making that influences future outcome selection (15). Deviations from normative anticipatory processing (e.g., hyper- and hypo-responsiveness) in brain areas implicated in reward are commonly observed in psychopathologies with impulsive and risk-seeking behavioral phenotypes including attention deficit hyperactivity disorder (ADHD) (3, 4, 16) and substance use (17, 18). However, findings have at times been discrepant. For example, while some studies have reported hyperactivation across areas of the brain, including the ventral striatum, during anticipatory reward in ADHD (4), other work has shown hypoactivation (3, 19, 20), or no differences (21), in the ventral striatum. These conflicting results may be due in part to varying levels of trait impulsivity across study populations (3) and differences between ADHD subtype. Furthermore, hypoactivation in the ventral striatum during anticipatory reward has been observed in adolescent smokers with limited smoking histories (18) whereas other work has found hyperactivation in areas of the striatum may serve as a risk factor for substance use initiation (17).
Given that findings of reward responsiveness within the context of impulsive and risk-seeking psychopathologies are varied and may depend on distinct subtypes within a population, integrative approaches using well-powered large, diverse samples are warranted. Identifying latent dimensions of reward and impulsivity across the brain and behavior, particularly during early adolescence, may shed light on complex systems subserving risk for common psychiatric disorders and/or identify common sources of individual variation within reward systems across development. Here, we therefore take a multivariate pattern learning approach to identify latent features of reward in a large sample of youth, providing a unified framework linking together multiple aspects of behavior and the brain. In doing so, we aim to shed light on interactions across these core domains and inform current understanding of how the expression of specific reward-related phenotypes relate to clinical features.
To accomplish the above study aims, we selected relevant measures collected by the Adolescent Brain and Cognitive Development (ABCD) study during middle childhood, or ‘pre-adolescence’, corresponding to data collected at ~age 10, consistent with landmark longitudinal work tracking (non-fMRI) facets or risk and impulsivity (22). For our analysis approach, we then selected a statistical approach capable of identifying associations between two sets of variables, canonical correlation analysis (CCA). CCA is a data-driven, pattern-learning approach for identifying associations between sets of variables (i.e., a ‘many-to-many’ approach) (23). It is therefore well suited for the identification of latent dimensions shared across different data sources (e.g., fMRI and non-fMRI datasets), and thus optimal for the proposed work. In the CCA framework, this co-variance between sets of data is commonly referred to as a ‘mode’ and represents pairs of latent features of each variable set (23) (this may be considered as roughly analogous to a component when using Principal Component Analysis). These latent features for each mode serve as unique brain-behavior profiles that can be used to identify distinct characteristics of clinical psychopathology across multiple dimensions simultaneously.
Based on previous multivariate findings in ABCD (24-26), we anticipated that CCA would identify one or more primary modes linking brain and behavioral assessments of impulsivity and reward, and that individual variation on these modes would be linked to individual differences in related but separately measured (i.e., not included in the CCA analysis) reward-related phenotypes; e.g., psychiatric diagnoses of ADHD, Disruptive Behavior Disorders (DBD), and Obsessive Compulsive Disorder (OCD) based on clinical interviews, NIH Toolbox measures of executive functioning (24). Given previous findings from work outside of ABCD (27, 28), we further hypothesized that individual-level variation in behavioral measures (e.g., delay discounting, in-scanner task performance) would be most closely linked to individual differences in patterns of anticipatory reward-processing brain responses, relative to self-report and caregiver assessments. Specifically, as has been previously reported in ABCD and elsewhere, we expected that individual differences in both ventral and dorsal (25) striatal engagement, as well as anterior cingulate cortex (ACC) engagement (25), during anticipatory reward processing would be linked to externalizing disorders (e.g., ADHD, DBD) (25) and behavioral (29) measures of impulsivity, such that increased levels of behavioral impulsivity (e.g., more delay discounting) would be associated with relatively decreased (i.e., blunted) neural response to reward anticipation (18). Finally, we further hypothesized that scores from identified modes of shared reward co-variation would have more robust associations with clinical measures than traditional, univariate measures (e.g., BOLD response within a single ROI correlated with a single clinical measure of reward).
Methods
ABCD dataset
The ABCD study is a longitudinal assessment of adolescent development across 21 sites in the United States with an enrollment of 11,875 youth (30, 31). ABCD offers an unprecedented opportunity to explore these relationships before the onset of significant psychopathology (30, 31) within a large, diverse recruitment sample. This immense dataset also affords researchers the opportunity to validate previous findings that may have been obtained in smaller sample studies, and to test whether prior effects scale to larger, more heterogenous samples such as ABCD. Participants are assessed annually, beginning at age 9/10 on behavioral, clinical, and psychosocial measures in order to facilitate understanding of adolescent neurodevelopment (32). In addition, multimodal magnetic resonance imaging (MRI) data is collected every other year (33). To support our aim of identifying latent brain-behavior dimensions of impulsivity and reward among youth prior to adolescence, data from the baseline collection point and year one follow-up were used. All data were downloaded from the Data Exploration and Analysis Portal for ABCD (https://deap.nimhda.org) under NIMH Data Use Agreement #7342 (Release 3.0).
Construction of datasets to identify latent features
As described above, CCA is an approach for identifying dimensional associations between two multivariate datasets. The first variable set, X—hereafter referred to as the Neuroimaging dataset—contained 32 beta-weight estimates corresponding to neural activation during MIDT reward anticipation within brain regions previously implicated in reward encoding (details below and in SI). The second variable set, Y—hereafter referred to as the Behavioral dataset—contained 42 well-validated self-report, parent-report and behavioral measures relevant to reward processing and risk-taking behaviors.
Neuroimaging dataset
Neuroimaging reward measures were obtained from ABCD’s curated data release (i.e., all pre-processing and first-level modeling was conducted by the ABCD Consortium; details in SI). We selected regions-of-interest (ROIs) that were defined using FreeSurfer’s ASEG (subcortical) and Desikan-Killiany (cortical) atlases. ROIs related to reward anticipation were selected a priori based on previous literature (25) from ABCD. ROIs included both cortical (anterior and posterior cingulate, orbitofrontal cortex, medial and lateral prefrontal cortex, occipital cortex, precentral and superior frontal gyrus, insula) and subcortical (amygdala, nucleus accumbens, caudate, putamen, thalamus, ventral diencephalon, hippocampus) regions (additional details in Supplemental Table 2). Poor quality neuroimaging data was excluded using the ABCD Recommended Image Inclusion data file (abcd_imgincl01). ABCD’s recommended inclusion criteria is specific to each neuroimaging modality and takes into account in-scan behavioral performance, repetition times, image quality, and neurological screenings (33, 34). Data from 8,295 individuals (nMale=4,190; nFemale=4,105) were available after excluding individuals based on ABCD’s MID-specific recommendation (imgincl_mid_include). See Yang and Jernigan (n.d.) (35) for specific considerations, variable names, and thresholds.
Behavioral dataset
Self-report behavioral measures included the modified Behavioral Inhibition/Behavioral Activation System (BISm/BASm) (36, 37) and the Urgency-Premeditation-Perseverance-Sensation Seeking-Positive Urgency (UPPS-P) (38). Caregiver-reported behavioral measures included all nineteen subscales from the Childhood Behavior Checklist (CBCL) (39, 40). Task-based behavioral variables included indifferences scores for all delays assessed during the Delay Discounting task (41-43) (see supplemental material for details and limitations) as well as reaction times and total earnings for each run of MIDT (44) (additional details in SI). A list of all 42 behavioral measures and their corresponding ABCD variable name can be found in Supplemental Table 1.
Multivariate, pattern learning analyses of neuroimaging and behavioral data
As described above, we chose a multivariate, pattern learning approach to identify associations between sets of variables from fMRI and behavioral domains, i.e., CCA. CCA identifies common patterns of co-variation between two multivariate datasets without assumptions of directionality (see Wang et al. (2020) for review) (23). These common sources of variation are represented as new latent variables, defined as canonical variates, which are then used to obtain a canonical correlation. Pairs of canonical variates are often referred to as “modes”, with the first mode explaining the largest amount of variance between the two sets of data. Subsequent canonical variate pairs, or modes, are uncorrelated with, and provide less explained variance than all previous modes. Canonical loadings are obtained for each variable in both sets and represent their contribution to a given mode’s explained variance. Our analysis pipeline followed closely to that of Dinga et al. 2019 (45) and is briefly described below. All statistical analyses were performed in R (4.0.3) using custom functions (45) and prepackaged libraries including ‘candisc, ‘lme4’, ‘emmeans’, and ‘caret’.
Consistent with prior work in ABCD (25), we controlled for potential confounding effects of participant sex at birth, interview age, parent income, and highest education obtained by a parent. This was done by residualizing from each single column separately, for both datasets before performing the CCA. Missing data was median imputed (caret, R) before carrying out the CCA (cancor, R), consistent with current recommendations (23) (Neuroimaging=7.3% or 19,986 of 273,735; Behavioral=7.6% or 26,498 of 348,390). Permutation testing (n=1,000) was performed to confirm the validity of the test statistics (Canonical Correlation; Wilk’s Lambda) obtained from statistically significant modes (45, 46) by permutating rows of data to obtain a null distribution of test statistics under the assumption of non-systematic associations between both variable sets. The p-value obtained from this distribution is derived from the proportion of permutations with values less than those obtained in the original, unshuffled variable sets (47). A non-significant permutation test would indicate that the true CCA model test statistics, using the original, unshuffled data, may have been obtained due to chance alone.
Finally, we used 10-fold cross-validation (CV) as an additional form of model validation to obtain out-of-sample estimations, ensuring study sites were equally distributed across each subset, to assess the generalizability of statistically significant between-canonical-variate correlations (45, 47). CV controls for overfitting by testing model parameters on an independent set of data not used in the model building (47). For our 10-fold CV, the dataset was randomly split into a training (90%) and test (10%) set across 10 iterations and canonical correlations obtained from each iteration were averaged for a final correlation.
Follow-up characterization of latent features in relation to other variables
Linear mixed-effect models (lme4 package, R) were used to evaluate associations between individual differences in subject-level canonical variate expression (technically, ‘projections’) mapped to diagnostic categories and dimensions of cognitive and executive functioning (48). Each variate set (i.e., brain and behavior) was tested separately for robust associations with the diagnostic and executive function measures. Given the nested structure of ABCD, all mixed-effect models included random nested effects for family and site as recommended in the ABCD Data Exploration and Analysis Portal (DEAP). Thus, each model contained two independent variables accounting for the nested structure and one which served as the primary variable of interest. Significant effects were followed up with Tukey post-hoc analyses (emmeans package, R).
Relevance of canonical variates to clinical diagnoses
Individual participant expressions for the identified CCA modes were entered as dependent variables in mixed-effect models focusing on psychiatric diagnoses with potential relevance to reward-processing, as assessed via semi-structured interview using the Kiddie-Schedule for Affect Disorders and Schizophrenia (K-SADS) (49). We used the K-SADS to identify youth with lifetime (i.e., past or present) diagnoses of disruptive behavioral disorders (DBD), which was a combined total from youth diagnosed with either conduct disorder or oppositional defiant disorder (DBD; n=1,111) (25), attention-deficit hyperactivity disorder (ADHD; n=1,604) (50) and obsessive-compulsive disorder (OCD, n=816) (51). Complete data was available for 8,190 of the 8,295 adolescents with good quality imaging data. To control for multiple comparisons within each set across the three diagnoses (i.e., DBD, ADHD, OCD), results were considered significant at p<.017 (.05/3). While we considered comparing CCA modes between youth with and without other reward-relevant diagnoses (e.g., bipolar disorder), the incidence rates of these other diagnoses were determined to be too low in the entire ABCD sample (n<1,000 in full sample; e.g., N=505 in current sample across all bipolar subtypes). Nonetheless, the inclusion of DBD, ADHD, and OCD allowed us to evaluate the relevance of our identified modes to both externalizing (DBD, ADHD) and internalizing (OCD) disorders (52, 53). See Supplemental (Section 1.6) for details regarding the relevance of the canonical variates to executive functioning.
Results
Permutation testing and 10-fold cross-validation
As detailed above, we used two forms of model validation in our analysis to ensure robustness and reproducibility: permutation testing and 10-fold CV (results prior to these steps are presented in the SI). This approach identified a single primary brain-behavior mode of shared covariation with an effect size greater than r=0.02 (Figure 1, rMode 1=0.11; Supplemental Figure 1, rMode 2<=0.00; Supplemental Figure 2 rMode 3=0.02; Supplemental Figure 3, rMode 4<=0.00).
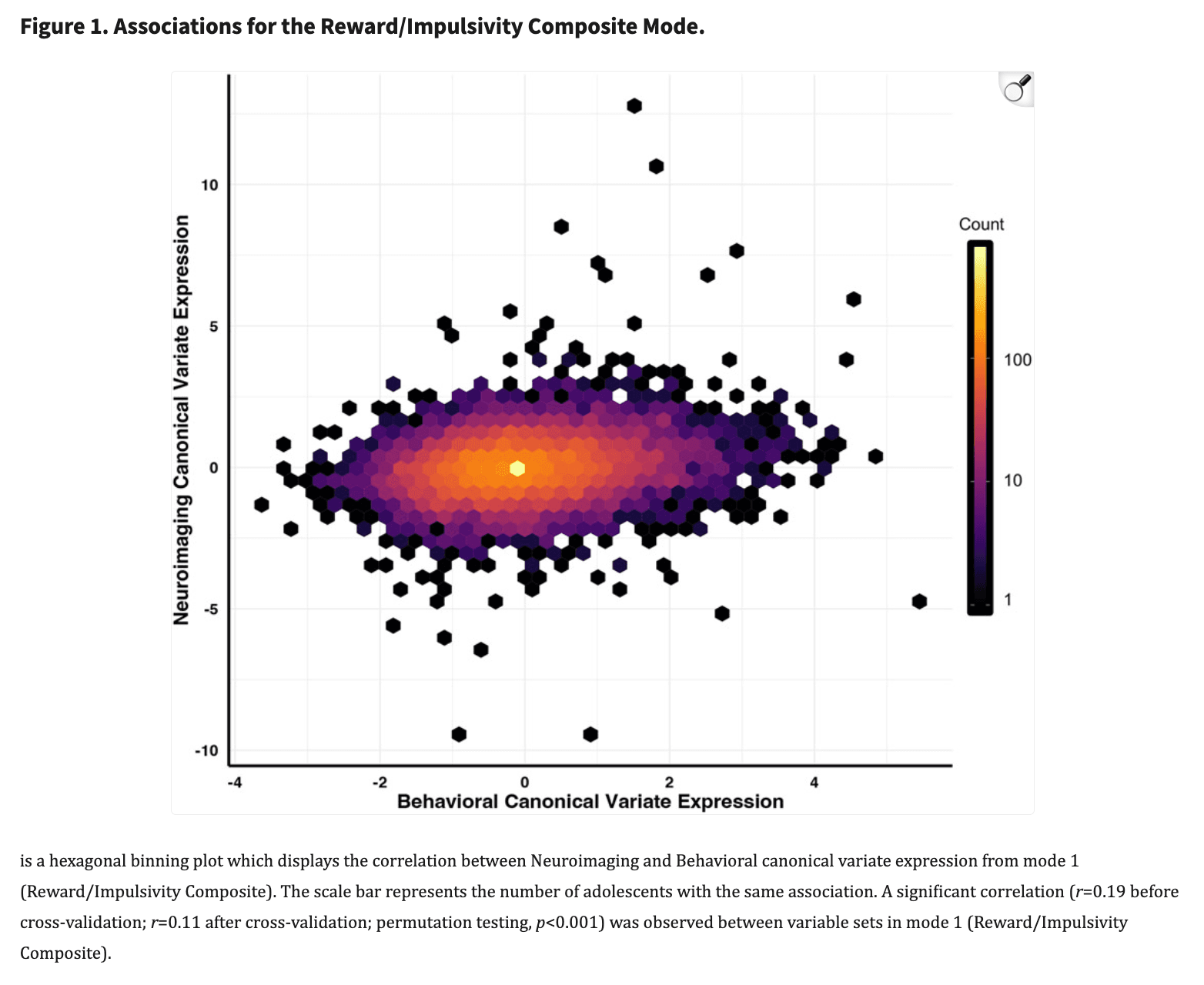
Multivariate relationships between clinical, behavioral, and brain measures of reward
Having identified a significant mode confirmed with cross-validation, hereafter referred to as the Reward/Impulsivity Composite (RIC) mode, we next characterized the precise relationships between top contributors in each set of variables. Figure 2 displays the top 10 canonical loadings corresponding to the Behavioral dataset (Figure 2A) and the Neuroimaging dataset (Figure 2B) for the RIC. These loadings represent the linear correlation between the RIC and the original variables and exist on a negative-positive spectrum. Thus, larger absolute values reflect a greater contribution to the RIC and a positive (negative) loading indicates that higher (lower) values of the variable were associated with a higher RIC score. For example, if brain region A had a loading of −0.05, and brain region B had a loading of 0.05, both regions would have the same magnitude of effect, but in opposite directions, with A associated with a lower RIC score and B associated with a higher RIC score, on average across subjects.
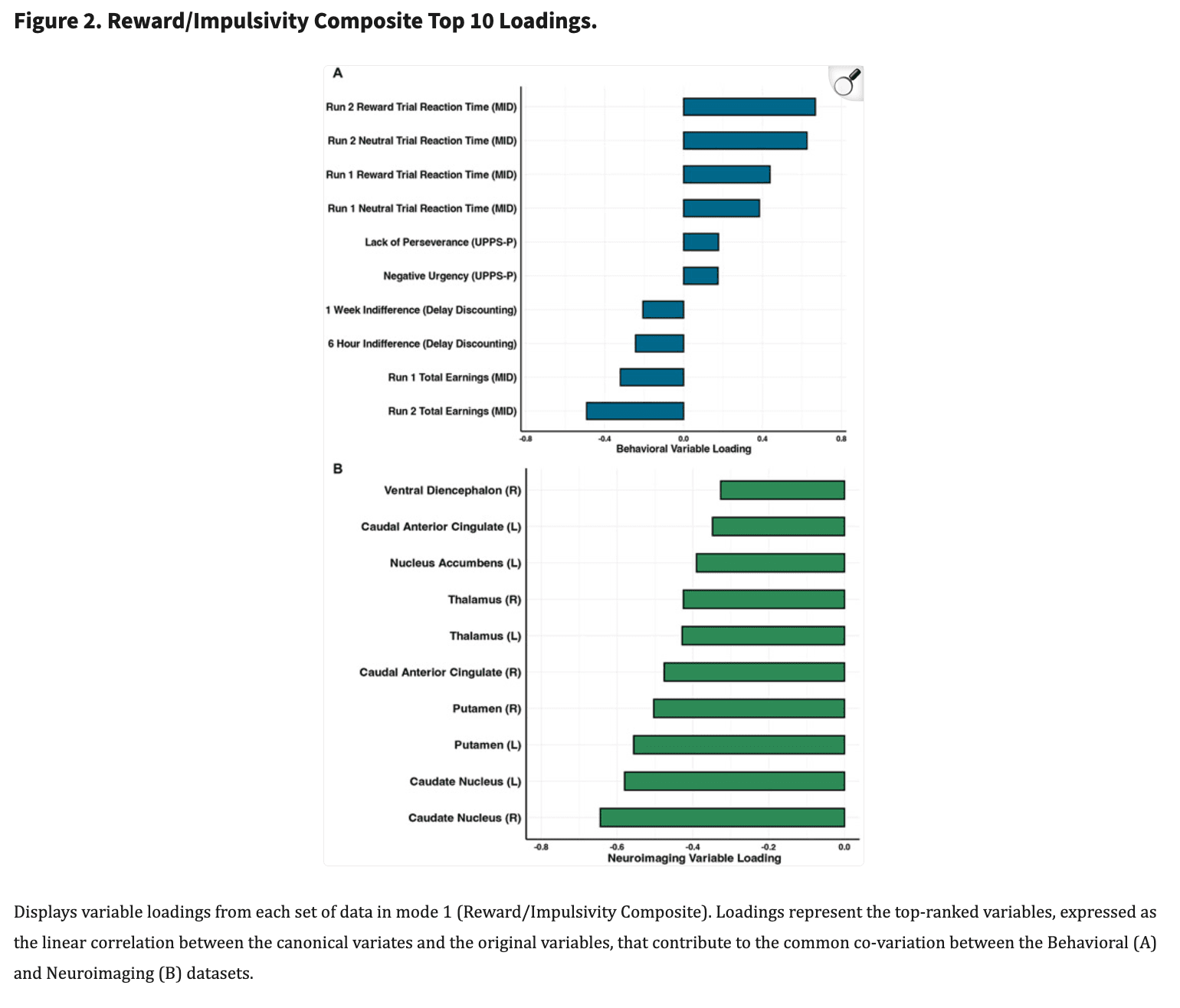
At the behavioral level, multiple performance indices from the MIDT (i.e., total earnings and reaction times for reward and neutral trials) and delay discounting scores for short delay trials (i.e., 6-hour, 1-week) emerged as the primary contributors to mode 1 in explaining variation in the RIC. Specifically, decreased MIDT total earnings (i.e., worse overall performance), increased reaction times (i.e., slower responding which also indicates worse performance) for neutral and positive trials on the MIDT, and lower indifference scores (i.e., higher delay discounting) were associated with higher RIC scores. At the self-report level, the negative urgency (i.e., impulsive actions resulting from negative affect) and lack of perseverance (inability to complete difficult or time-consuming tasks) subscales of the UPPS-P emerged as the primary contributors, such that higher UPPS-P scores were associated with higher RIC scores.
At the neurobiological level, lower task-evoked patterns of regional brain activation consistently emerged as primary contributors to RIC scores. Specifically, during reward anticipation, decreased activations within bilateral caudate, bilateral putamen, bilateral thalamus and bilateral anterior cingulate were all associated with higher RIC scores (see Supplemental Figure 4 for visualizations). Taken together with findings from behavioral and self-report domains, these data indicate that individuals with higher (vs. lower) RIC scores are characterized by increased self-reported impulsivity, impaired reward task performance, steeper short-term delay discounting slopes (i.e., preferring shorter, smaller rewards) and blunted cortico-striatal-thalamic activation during reward anticipation.
Univariate Comparisons
As described above, our multivariate pattern learning approach identified a primary mode, the RIC, that accounted for a subtle yet statistically significant amount of variance (rMode 1=0.11). For comparison, we also computed univariate effect sizes for the highest loaded neural measure of reward (right caudate nucleus) and the highest loaded clinical measure (UPPS-P) using the original variables. Results of this univariate test (Supplemental Figure 5) indicated much smaller effect sizes for univariate associations of the original variables, with maximum r-values of ~0.03. Having established the increased exploratory power of our multivariate approach, we next went on to explore the relationship between individual differences in RIC scores, potentially relevant psychiatric diagnoses and executive functioning indices.
Relationships to clinical diagnoses and executive functioning measures
Adolescents diagnosed with DBD (n=1,111, μ=0.010, σ=0.067) had significantly higher Behavioral dataset canonical variate expressions compared to adolescents without a DBD diagnosis (n=7,079, μ=−0.006, σ=0.062, p<0.001, d=0.122) (Figure 3A, see Supplemental Figure 6 for violin plots). In addition, adolescents with a history of an ADHD diagnosis (n=1,604, μ=0.225, σ=0.065) had significantly higher Behavioral dataset variate scores when compared to those without (n=6,586, μ=−0.047, σ=0.062, p<0.001, d=0.317) (Figure 3B). (See supplemental material for unchanged results after addition of stimulant medication status to the ADHD model). No difference was observed between adolescents with (n=816) and without an OCD diagnosis (n=7,374, p=0.873) (Figure 3C). For the Neuroimaging dataset, adolescents diagnosed with ADHD (n=1,1604, μ=0.057, σ=0.029) had significantly higher canonical variate expressions than those without (n=6,586, μ=−0.013, σ=0.019, p=0.012, d=0.071) (Figure 3E). No differences were observed between the Neuroimaging dataset variate scores and diagnoses of DBD (n=1,604, p=0.462) or OCD (n=816, p=0.99). These significant effects remained after including the covariates that were used to residualize the data prior to running the CCA (i.e., sex at birth, interview age, parent income, and highest education) and suggests these relationships may be robust to individual differences in core demographics.
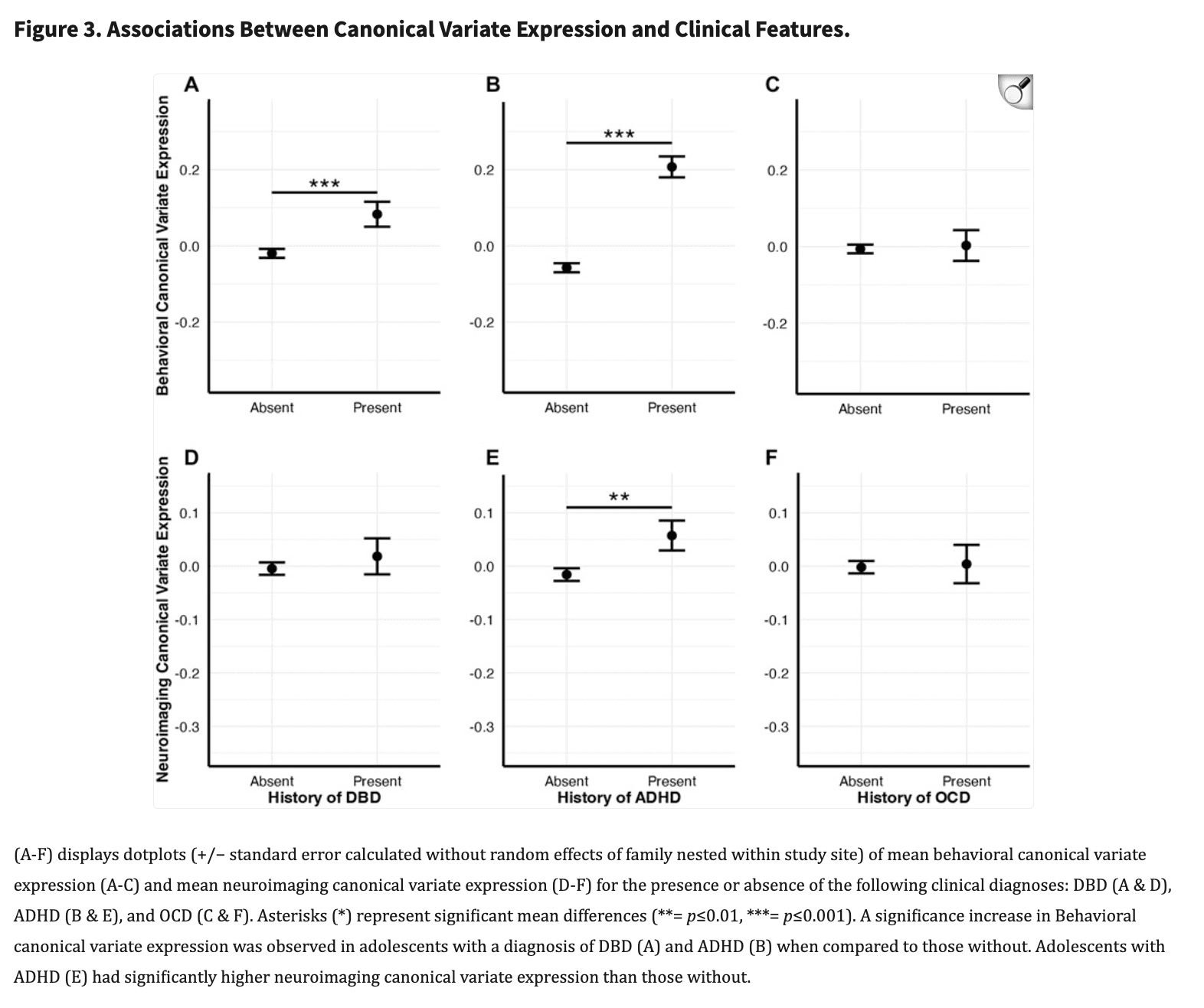
Significant correlations were observed between the Behavioral and Neuroimaging canonical variate expressions and NIH Toolbox cognitive performance (Supplemental Figure 7), such that higher variate expressions (i.e., higher behavioral impulsivity and lower anticipatory brain response) were associated with worse cognitive task performance.
Discussion
Using a conservative cross-validation approach and permutation testing, our data-driven analysis indicated that individual differences in specific behavioral domains—performance on the MIDT, delay discounting, lack of perseverance and negative urgency—covary with individual differences in anticipatory reward processing within specific, primarily subcortical, neural systems including the thalamus, caudate, putamen and anterior cingulate. Notably, this constellation of measures suggests that low neural response to reward anticipation is most closely linked to behavioral indices of reward sensitivity (e.g., slower reaction times to obtain rewards or avoid losses; delay discounting) and to self-report indices of negative aspects of impulsivity (i.e., negative urgency, lack of perseverance), in comparison to more classic, positive aspects of impulsivity (e.g., sensation seeking, positive urgency, BIS/BAS; see SI for additional Discussion). These results further indicate that such behavioral facets of impulsivity are more closely linked to individual differences in primarily ‘bottom-up’ subcortical brain regions relative to ‘top-down’ cortical regions. Together with the work by Hawes et al. (2021), this suggests that anticipatory reward processing during early adolescence might relate more to externalizing phenotypes than clinical assessments like the BIS/BAS, CBCL, and in part the UPPS-P. Longitudinal follow-ups will help determine whether the construct we identified is consistent across development or if there are others that emerge.
At the neural level, primarily subcortical brain regions—including the caudate and putamen—emerged as the primary drivers of the RIC, consistent with these regions’ critical role in reward-processing (54-56) and with developmental models emphasizing earlier maturation of subcortical vs. cortical structures (57). Among the cortical ROIs considered, only the anterior cingulate cortex emerged as a primary contributor to the RIC. The negative loadings for all brain-based loadings suggest decreased activation is associated with increased RIC scores. This relationship was further evidenced by our validation analysis findings for adolescents with ADHD and supports previous findings, particularly within the ventral striatum (19). Further discussion of these brain regions and their relevance to the RIC is provided in the SI.
Follow-up analyses comparing individuals with and without common reward-related psychiatric disorders indicated significantly increased scores for both brain- and behavior-based components of the RIC among youth with a history of ADHD. Given the negative loadings of variables in the brain-based components, this indicates adolescents with ADHD have blunted activation in areas of reward, consistent with prior work in smaller samples and using univariate approaches. In addition, the direction of the loadings for the behavior-based set suggests a higher severity, or worse performance, on the tasks and assessments in this set, together, constitute a phenotype of reward and impulsivity that is associated with ADHD. This interpretation is supported by previous work in both adolescents (19) and adults (58) with ADHD that found striatal hypoactivation to be associated with greater behavioral symptoms of impulsivity. Importantly, our replication using such a large sample increases the validity of these earlier findings that were observed in significantly smaller samples. This replication using a multivariate approach also provides a foundation for studies using multivariate analysis by demonstrating its utility in identifying latent features of psychopathology which simultaneously link aspects of the brain and behavior.
In contrast, while youth with a DBD diagnosis had increased expressions of behavioral measures, no differences in brain-based components of the RIC were found for youth with and without DBD. Despite differences in analytic strategy, the absence of brain-based differences in reward activation among youth with and without DBD is generally consistent with results from multivariate latent reward network models recently reported by Hawes et al. (2021) (25). For example, these authors found significant decreases in anticipatory reward network activation only in youth diagnosed with DBD when compared to healthy controls and those with both DBD and callous-unemotional (CU) traits. Youth with DBD+CU did not display reduced mean-level reward network factor activation during anticipatory contrasts when compared to healthy controls. Our sample presented here was not restricted to only DBD individuals and more closely reflects a heterogenous population that includes youth with comorbid disorders (e.g., callous traits). Nonetheless, Hawes et al. observed reduced activation in the dorsal anterior cingulate cortex in both DBD only and DBD+CU adolescents when compared to healthy controls in their univariate region of interest analysis. While our brain-based validation analysis was not significant for adolescents with DBD, the anterior cingulate was the highest loaded cortical region in our CCA and further suggests this area may be important in the development of adolescent reward-related psychopathology.
Our study has several limitations. First, effect size estimates were modest. However, recent studies have begun to challenge the definition of what constitutes a small or modest effect size, particularly within the context of big data initiatives such as ABCD (59-61). This modest effect size may also be due in part to heterogeneity within our clinical sample that is related to over-reporting of clinical diagnoses on self-report assessments relative to clinician interviews. Nonetheless, small effects are also useful for developing novel hypotheses and can be more important at the population-level (62). Second, although our multivariate pattern learning strategy was entirely data-driven, it was constrained by our a priori selection of assessments and brain regions with documented relevance to reward. Furthermore, we chose to only include neural activation data from reward vs. neutral anticipatory contrasts of the MID, and as such, our findings should only be considered in the context of anticipatory reward processing. While we only considered ADHD, DBD, and OCD in our follow-up analyses, the rates of other clinical diagnoses are low. Thus, it will be imperative to assess RIC expression across other disorders (i.e., depression) as the prevalence rates increase in future ABCD releases. We also did not control for other development factors such as differences in puberal status in our analysis. However, recent work suggests that at baseline most adolescents have not yet entered puberty (63) and thus may be important to consider at later timepoints. While we considered an exhaustive approach (i.e., inclusion of all possible variables, as in our prior work) (64), we ultimately elected to combine our data-driven analysis with theory-based variable selection. This was because we were interested in determining whether commonly used indices of reward taken from multiple levels of analyses would in fact naturally covary together, as anticipated by common translational approaches such as RDoC (5-8). While future work should consider a truly exhaustive approach, it is important to note that this will involve inclusion of 1,000’s of variables and significant computational resources. To our knowledge, the present analysis nonetheless represents the largest, data-driven, multivariate assessment of reward-processing metrics across behavioral, clinical and neuroimaging domains. Finally, it is important to acknowledge the limitations of ABCD’s imaging data. Although we removed participants based on ABCD’s neuroimaging exclusion criteria, recent work has called into question the reliability and stability of the task-based fMRI data, particularly when considering longitudinal analyses (65). In addition, the ABCD consortium uses multiband acquisition which may produce less reliable mesolimbic activity measurements (66). As future waves of imaging data become available, it will be important to evaluate the reliability of mesolimbic activity in relation to specific psychopathologies.
In summary, the present study used a multivariate statistical approach to identify relationships between behavioral and neuroimaging assessments related to reward. Our results suggest that significant patterns emerge in both the brain and behavior during early adolescence that are representative of reward-related psychiatric disorders such as ADHD. These findings may have significant implications for the treatment of reward-related disorders as they highlight important phenotypes (e.g., discounting, negative urgency, perseverance) that may act as intervention targets and are robust to core demographics. Importantly, our multivariate analysis allowed for simultaneous brain-based findings that take into account the relationship between all included regions (vs. single regions in isolation), thereby revealing the neural correlates of these phenotypes. Future waves of ABCD data will help determine whether these specific phenotypes are stable over time and how these patterns of reward relate to other disorders including the development of other common reward-related disorders and behaviors (e.g., substance use initiation, bipolar disorder, depression). More generally, these data have important implications for translational work in humans as they highlight the importance of multivariate approaches for understanding unified frameworks of brain-behavior relationships and suggest that reliance on univariate analyses to explain complex, interconnected phenomena may limit our potential to identify latent features of human psychopathology. Thus, it will be important for future work to consider building upon earlier studies that have relied on univariate frameworks. We believe our comparisons here provide a foundation for future extensions and generalizations of earlier work. They further provide an implicit baseline from which the development of the newly identified RIC may be tracked in future waves of ABCD.
Supplementary Material
Supplemental Material
NIHMS1997223-supplement-Supplemental_Material.docx (1.3MB, docx)