Abstract
Risk-taking often occurs in childhood as a compex outcome influenced by individual, family, and social factors. The ability to govern risky decision-making in a balanced manner is a hallmark of the integrity of cognitive and affective development from childhood to adulthood. The Triadic Neural Systems Model posits that the nuanced coordination of motivational approach, avoidance and prefrontal control systems is crucial to regulate adaptive risk-taking and related behaviors. Although widely studied in adolescence and adulthood, how these systems develop in childhood remains elusive. Here, we show heterogenous age-related differences in the triadic neural systems involved in risky decision-making in 218 school-age children relative to 80 young adults. Children were generally less reward-seeking and less risk-taking than adults, and exhibited gradual increases in risk-taking behaviors from 6 to 12 years-old, which are associated with age-related differences in brain activation patterns underlying reward and risk processing. In comparison to adults, children exhibited weaker activation in control-related prefrontal systems, but stronger activation in reward-related striatal systems. Network analyses revealed that children showed greater reward-related functional connectivity within and between the triadic systems. Our findings support an immature and unbalanced developmental view of the core neurocognitive systems involved in risky decision-making and related behaviors in middle to late childhood.
1. Introduction
Human brain undergoes rapid development with dramatic changes in cognitive and affective functions including risky decision-making and related behaviors (Teicher et al., 2016) to ensure survival and well-being (Greitemeyer et al., 2013, Kim et al., 2017). Under the influence of various individual (e.g., personal motivations, temperament) (Boles et al., 2005), family (e.g., parenting styles, sibling effects), and social-situational (e.g., observational influences, situation-driven motivations) factors (Barbara and Jennifer, 2007, Boyer, 2006), risk-taking behaviors occur early in childhood, which increase from then on, peak in adolescence and decline into adulthood according to epidemiological data (Rosenbaum and Hartley, 2019, Steinberg, 2013, Willoughby et al., 2014). Although to some extent reflective of the normative developmental pattern (Bjork and Pardini, 2015, Crone et al., 2016), risk-taking among young children, in some extreme cases (i.e., excessive externalizing behaviors), is considered as a predictor of adolescent conduct disorders (Crowley et al., 2017, Fanti et al., 2016). Yet, contrasting with ample work on the neural substrates underlying risk-taking in adolescents and adults (Guassi Moreira et al., 2021, Richards et al., 2013), it remains elusive how these systems organize to support related functions in childhood. Knowledge of such neural underpinnings in childhood is important for understanding precursors of adolescent risky behaviors and ontogenies of malfunctions in related psychiatric disorders (Casey and Jones, 2010, Dalley and Robbins, 2017).
Previous studies have demonstrated behavioral characteristics of risky decision-making from childhood to adulthood (Defoe et al., 2015, Humphreys et al., 2016), neural substrates underlying risky decision-making are nevertheless mainly examined in adolescents and adults (Braams et al., 2015, Pei et al., 2020). Recent neuroimaging studies began investigations into children, however, focused on single component (e.g., reward processing) of the complex risky decision-making processes (Blakemore and Robbins, 2012) by testing task-evoked regional activity (Crowley et al., 2017, Morelli et al., 2021, Szenczy et al., 2021). Aiming to decipher complex risk-taking and motivated behaviors, several system-based models have developed over the years with the focus on adolescence (Casey, 2015), including the dual-system models (McClure et al., 2004, Shulman et al., 2016, Steinberg, 2010), the triadic model (Ernst et al., 2006) and the imbalance models (Casey et al., 2008, Li, 2017). These models can be used to guide our understanding of neural mechanisms underlying risky decision-making in childhood. In comparison with dual-system models and imbalance models focusing on the balance between motivational limbic system and prefrontal control system, the Triadic Neural Systems Model (Ernst, 2014, Richards et al., 2013) dissects the limbic system into a reward-driven and a harm-avoidant subsystem, and posits three core neurocognitive systems including control, approach and avoidance modules (Ernst and Fudge, 2009). This model figures as a broader theory of functional mechanisms underlying motivated behaviors, and examines most of related brain areas reported in previous empirical studies. Specifically, the Control module consists of dorsal anterior cingulate cortex, dorsolateral prefrontal cortex, and ventromedial prefrontal cortex that carry distinct functions such as salience detection and inhibition (Chikazoe et al., 2007, Kouneiher et al., 2009, Rubia et al., 2010); The Approach module includes striatal regions nucleus accumbens, caudate, and putamen critical for reward function and motivation (Berridge and Kringelbach, 2015, Jensen et al., 2003); The Avoidance module includes amygdala, insula, and hippocampus which are consistently associated with emotional perception and response to aversive stimuli (Hardin et al., 2009, Rauch et al., 2003) (Table 1). Taken as a reference of maturity, the adult pattern of this model shows a nuanced and balanced coordination of these three systems to support the adaptive risk-taking behaviors. By contrast, the adolescent model is recognized as an unbalanced one and tilts towards approach behavior, characterized by enhanced responsivity of the striatal regions to appetitive stimuli (Ernst and Hardin, 2009, Galvan, 2010). This possibly results from a stronger reward-driven system, but a weaker harm-avoidant system, and poor regulatory controls during adolescence (Ernst et al., 2006). However, whether such unbalanced pattern originates from childhood or not remains to be unraveled. Moreover, there is still a lack of systematic investigations into functional coordination of the triadic neural systems in children.
Table 1. Neural substrates included in the Triadic Neural Systems Model.
Modules of the Triadic Neural Systems Model | ||
---|---|---|
Control | Approach | Avoidance |
Regions of Interest/Seed Regions | ||
Dorsal | Nucleus Accumbens | Amygdala |
Anterior Cingulate Cortex | ||
Dorsolateral | Caudate | Insula |
Prefrontal Cortex | ||
Ventromedial | Putamen | Hippocampus |
Prefrontal Cortex |
Here we aim to examine the neurocognitive development of risky decision-making during childhood using event-related functional magnetic resonance imaging (fMRI) with an adapted Balloon Analogue Risk Task (BART) (Lejuez et al., 2002) in a cross-sectional sample of 218 typically developing children (aged 6–12) and 80 healthy young adults (aged 20–26). We opted a modified BART paradigm which partitioned risky decision-making into three major conditions corresponding to triadic neurocognitive processes including approach, avoidance and control (Ernst et al., 2019), such design allows us to investigate most of the regions of interest reported in previous BART studies (Dir et al., 2019, Kohno et al., 2016, Poudel et al., 2022, Rao et al., 2008, Telzer et al., 2014). Based on the above-mentioned open questions and evidence from previous neurocognitive studies in adolescents and adults, we hypothesized that children would become more risk-taking as they grow older, and would show age-related increases of brain activity involved in reward processing and risk processing but no significant change of those regions engaging in control, partly due to the protracted development of prefrontal cortex. The overall age-related differences in behavioral performance and neural responses of risky decision-making were examined to test these hypotheses. We further hypothesized that triadic neural systems in children would show an adolescent-like pattern characterized by weaker engagement of the control system, but stronger activation of the approach system compared with adults, which may be associated with stronger connectivity of reward-related circuitry. To further reveal the childhood pattern of the Triadic Neural Systems Model and test these hypotheses, age-related differences in brain activation and functional connectivity among regions of the triadic neural systems were investigated by condition-wise Regions of Interest (ROIs) analyses using the adult pattern as a mature reference.
2. Materials and methods
2.1. Participants
A total of 331 participants were recruited in this study, which consisted of 250 typically developing children (126 girls; age range: 6–12; mean ± standard deviation [SD] = 9.21 ± 1.38) and 81 healthy young adults (44 females; age range: 20–26; mean ± SD = 22.62 ± 1.83). Neuroimaging and behavioral data were obtained from the Children School Functions and Brain Development Project (CBD, Beijing Cohort) (Wang et al., 2023, Xu et al., 2022). Children were recruited by handing out the booklets to several homogeneous elementary schools in Beijing. And adults were recruited from the corresponding local communities in Beijing. All participants had no history of vision problems and no history of neurological or psychiatric disorders, and no current use of any medication or recreational drugs. The experimental procedures were approved by local ethics in accordance with the standards of the Declaration of Helsinki. Written informed consent was obtained from each participant as well as the child’s legal guardian before their participation. Participants having excessive head motion with max displacement larger than 3 mm (32 children and 1 adult) were excluded from further behavioral and neuroimaging data analyses. Only the datasets from the remaining 298 participants including 218 children (113 girls; age range: 6–12; mean ± SD = 9.21 ± 1.39) and 80 adults (44 females; age range: 20–26; mean ± SD = 22.59 ± 1.82) were used in this study. Participant demographics are summarized in Fig. S9 & Supplementary Table S1.
2.2. Cognitive task
All participants completed a modified version of the Balloon Analogue Risk Task during fMRI scan, which can model the unpredictable rewards and risks that characterize real-world risky behaviors (Lejuez et al., 2002). On each trial of the task, participants were shown a virtual blue balloon in the center of the screen, and were given the option of pumping the balloon for a potential increase in rewards (“+ ¥ 1″ for each pump, the maximum number of pumps per balloon was 12) or cashing out to retain rewards accumulated during the current trial and transfer them to a permanent bank at the same time, by pressing one of two buttons within 3000 ms, otherwise the balloon would explode automatically for no response (Fig. 1A). Balloon explosion may also appear every time after participants pressed the “pump” button: once the balloon exploded, participants would forfeit unrealized earnings accumulated during the trial. The explosion point of each balloon was drawn from a uniform probability distribution from 1 to 12 pumps (Rao et al., 2008), and to encourage participants to make multiple inflation attempts for single balloon, the actual amount of monetary reward they could earn also increased accordingly with the number of inflations from 1 to 12 Chinese yuan (Supplementary Table S2). Thus, pumping could increase the size of the balloon, the accrued rewards in a temporary bank, as well as the likelihood of explosion. The larger the balloon was pumped, the greater the monetary reward but the higher the probability of loss. Participants were instructed that their goal during this task was to maximize the reward by balancing potential gain against potential risk of losing the accrued reward, and they would receive the equivalent of their total earnings as a bonus at the end of the study.
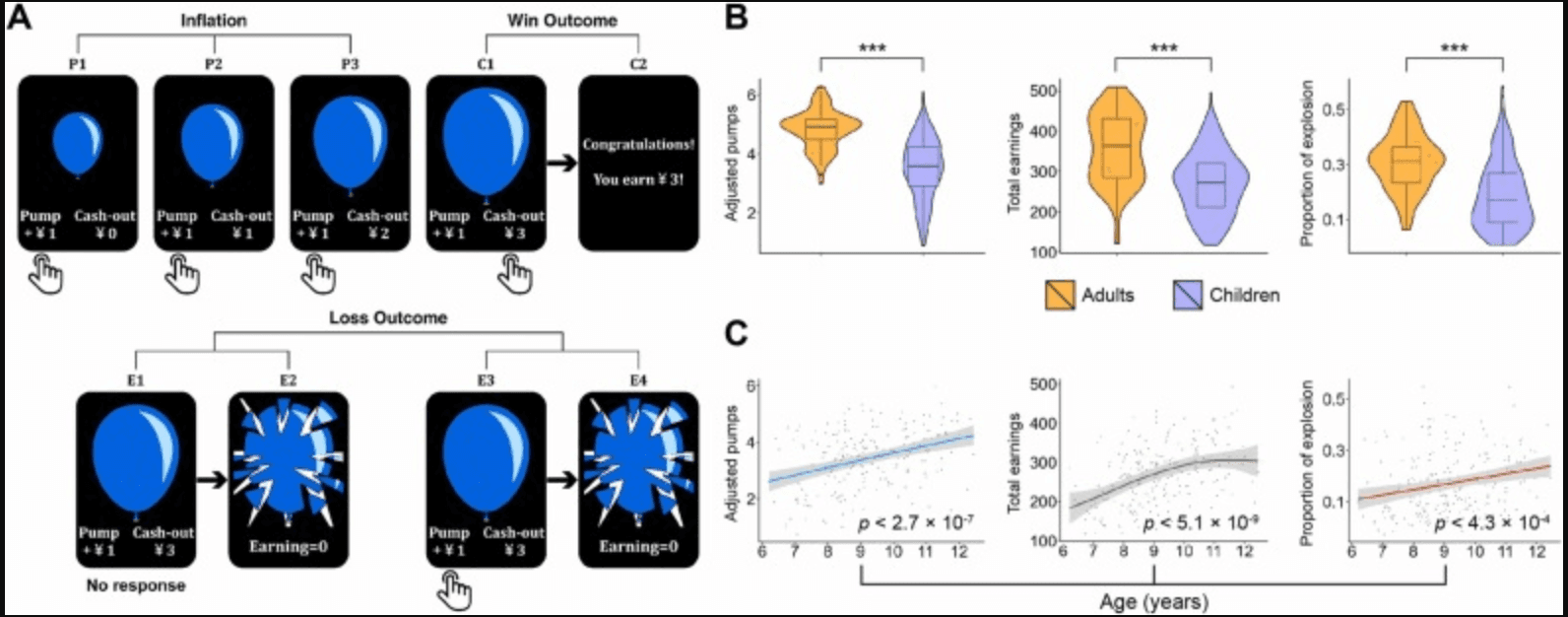
Fig. 1. Experimental design and behavioral performance of three core processes during risky decision-making. (A) An example trial of the BART: participants were shown a computerized balloon and selected between two button responses: “pump” or “cash-out”. By pressing the “pump” button, participants could sequentially inflate the virtual balloon as well as earn a monetary reward (1 yuan for every decision to pump the balloon) in a temporary bank (e.g., P1-P2-P3: “Inflation” condition). They could also retain rewards accrued during the current trial and transfer them to the permanent bank by pressing the “cash-out” button at any point (e.g., C1-C2: “Win Outcome” condition). The larger the balloon was inflated, the greater the rewards, but the higher the probability of balloon explosion happening after a “pump” choice, which led to the loss of corresponding rewards (e.g., E3-E4: “Loss Outcome” condition). Besides, making no response all through the 3000-ms display of a balloon would also result in an explosion (e.g., E1-E2: “Loss Outcome” condition). Arrows marked the end of a trial. (B) Violin plots showing BART behavioral performance in adults (n = 80) and children (n = 218) based on three indexes (i.e., average adjusted pumps, total earnings and proportion of explosion). (C) Average adjusted pumps, total earnings and proportion of explosion increase with age in children. Colored line/curve represents the best fit using the generalized additive model with shaded area indicating 95% confidence interval. Notes: * **p < 0.001.
In summary, every trial started with the presentation of the original balloon at the smallest size (P1 in Fig. 1A), included all pumps on the unexploded balloon (e.g., P1-P2-P3 in Fig. 1A; this stage was considered as “Inflation” condition, during which participants accumulated rewards by inflating the balloon, while they had to undertake the risk that balloon explosions may happen after a “pump” choice), and ended with the decision to cash out, which led to a display of the single-trial earned for 1500 ms (e.g., C1-C2 in Fig. 1A; this stage was considered as “Win Outcome” condition), or ended with a balloon explosion, which resulted in a 1500-ms feedback of an exploded balloon together with the message, “Earning = 0″ (e.g., E1-E2 and E3-E4 in Fig. 1A; this stage was considered as “Loss Outcome” condition). As the task was self-paced during one 6-minutes run, and each trial actually came to an end with a “cash-out” choice or an unexpected balloon explosion, the total number of completed trials was not predetermined, but depended on the response speed varying between participants. All stimuli were presented via E-Prime 2.0 (http://www.pstnet.com ; Psychology Software Tools, Inc).
2.3. Data analytic plan
To address the open questions and test the corresponding hypotheses (mentioned in the Introduction), age-related differences in behavioral performance of risky decision-making were first examined by implementing the generalized additive model to detect linear or non-linear relationships between age and behavioral measures. Next, to examine age-related differences in brain systems involved in risky decision-making, univariate and multivariate analyses were employed to quantify how neural responses of risky decision-making differ between age groups from 6 to 12 years old. Based on the Triadic Model framework, to further investigate the brain activation patterns underlying risky decision-making in children compared with adults, condition-wise (i.e., “Inflation”, “Win Outcome”, and “Loss Outcome”) ROI analyses and group comparisons were conducted to capture multi-dimensional differences in task-related activation at both module-level and single-region-level. Moreover, to explore the functional coordination among regions of the triadic neural systems in children compared with adults, task-dependent functional connectivity analyses, together with corresponding condition-wise ROI analyses and group comparisons, were conducted at both module-level and single-region-level. Details about these analyses are provided in the following sections.
2.4. Behavioral data analysis
As the number of pumps is constrained on balloons that explode, we indexed adaptive decision-making by calculating average adjusted pumps, which represents the average number of pumps on balloons that did not explode (Rao et al., 2018, Telzer et al., 2014), as well as total earnings (Kohno et al., 2016). Then we conducted corresponding independent t-tests to examine group differences (adults vs. children) in risky decision-making (Fig. 1B left & middle). Similarly, separate independent t-tests (adults vs. children) were performed for proportion of explosion (the ratio of exploded balloons to total balloons) and reaction time (Fig. 1B right & Fig. S1A). Subsequently, the generalized additive model (GAM) based on the R package “mgcv” (https://cran.r-project.org/web/packages/mgcv/index.html ) was used to investigate age-related differences of behavioral performance in the BART from 6 to 12 years old (Fig. 1C & Fig. S1B). Such a method allows us to detect the linear or non-linear relationships between age and behavioral measures without defining a set of priori functions (i.e., polynomials) (Baum et al., 2017). Notably, the GAM estimates nonlinearities using restricted maximum likelihood (REML), and determines a penalty with increasing nonlinearity in order to avoid over-fitting the data (Wood, 2006). We used the penalized splines to estimate developmental patterns of risky decision-making, and included gender as one of covariates in these models. Accordingly, the final model for estimating age effects on each BART behavioral score can be expressed as:
Y = Spline (Age) + Gender, where Y represents average adjusted pumps, total earnings, proportion of explosion or reaction time.
2.5. fMRI data acquisition
Whole-brain functional images were acquired from a 3 T Siemens MRI scanner (Magnetom Prisma syngo MR D13D, Erlangen, Germany) using a 64 head coil with a T2 * -sensitive echo-planar imaging (EPI) sequence based on blood oxygenation level-dependent (BOLD) contrast. Thirty-three axial slices (3.5 mm thickness, 0.7 mm skip) parallel to the anterior and posterior commissure (AC-PC) line and covering the whole brain were imaged with the following parameters: volume repetition time (TR) = 2000 ms, echo time (TE) = 30 ms, flip angle (FA) = 90°, voxel size = 3.5 × 3.5 × 3.5 mm3, field of view (FOV) = 224 × 224 mm2. A set of 184 volumes were collected during the BART scan in a single run. And each participant’s high-resolution anatomical images were acquired through 3 Dimensional sagittal T1-weighted magnetization-prepared rapid gradient echo (MPRGE) with 192 slices: TR = 2530 ms, TE = 2.98 ms, FA = 7°, inversion time (TI) = 1100 ms, voxel size = 0.5 × 0.5 × 1.0 mm3, acquisition matrix size = 256 × 224, FOV = 256 × 224 mm2, BW = 240 Hz/Px, slice thickness = 1 mm.
2.6. fMRI data preprocessing
Image preprocessing was performed using Statistical Parametric Mapping (SPM12, http://www.fil.ion.ucl.ac.uk/spm ). The first 4 volumes were removed for stabilization of magnetic resonance signal and participants’ adaptation to scanning noise. Remaining images were corrected for slice acquisition timing and realigned for head motion correction. Subsequently, functional images were co-registered to each participant’s gray matter image segmented from corresponding high-resolution T1-weighted image, then spatially normalized into a common stereotactic Montreal Neurological Institute (MNI) space and resampled into 2-mm isotropic voxels. Finally, images were spatially smoothed by convolving an isotropic 3D-Gaussian kernel with 6-mm full width at half maximum (FWHM).
2.7. Univariate general linear model (GLM) analysis
To assess task-related brain responses in the BART, three conditions including “Inflation”, “Win Outcome”, and “Loss Outcome”, were modeled as three separate event-related regressors and convolved with the canonical hemodynamic response function (HRF) implemented in SPM12. Additionally, each participant’s motion parameters derived from the realignment procedure were included to regress out effects of head movement on brain response. We performed high-pass filtering using a cutoff of 1/128 Hz, and conducted global intensity normalization and corrections for serial correlations in fMRI using a first-order autoregressive model (AR (1)) in the GLM framework. Subsequently, both individual- and group- level statistical analyses were conducted using SPM12.
Contrast parameter estimated images for “Inflation”, “Win Outcome”, and “Loss Outcome” conditions, initially generated at the individual-level, were submitted to group-level analyses treating participants as a random factor. Separate independent t-tests were performed to identify group differences (adults vs. children) in whole-brain activation under these three conditions of BART (Fig. S2). Analysis of variance with six different age groups including children (some groups had been merged into one because of their small sample size; e.g., Age 6 & 7) and adults was conducted to investigate how brain activation patterns underlying risky decision-making changed by age group (Fig. 2A). For visualization purposes, significant clusters were determined by using a height threshold of p < 0.005 and an extent threshold of p < 0.05 corrected for multiple comparisons using family-wise error corrections based on nonstationary suprathreshold cluster-size distributions computed by Monte Carlo simulations (Nichols and Hayasaka, 2003). Since the BART paradigm in current study was self-paced, the trial number of each condition will be differentiated across and within subjects, which may influence the group-level analyses of contrast activations. To clarify such issue, another group-level regression model taking the trial numbers of each condition as covariates was additionally conducted, and the results were corrected at the same levelas the above analyses for multiple comparisons. The corresponding results can be seen in Fig. S4.
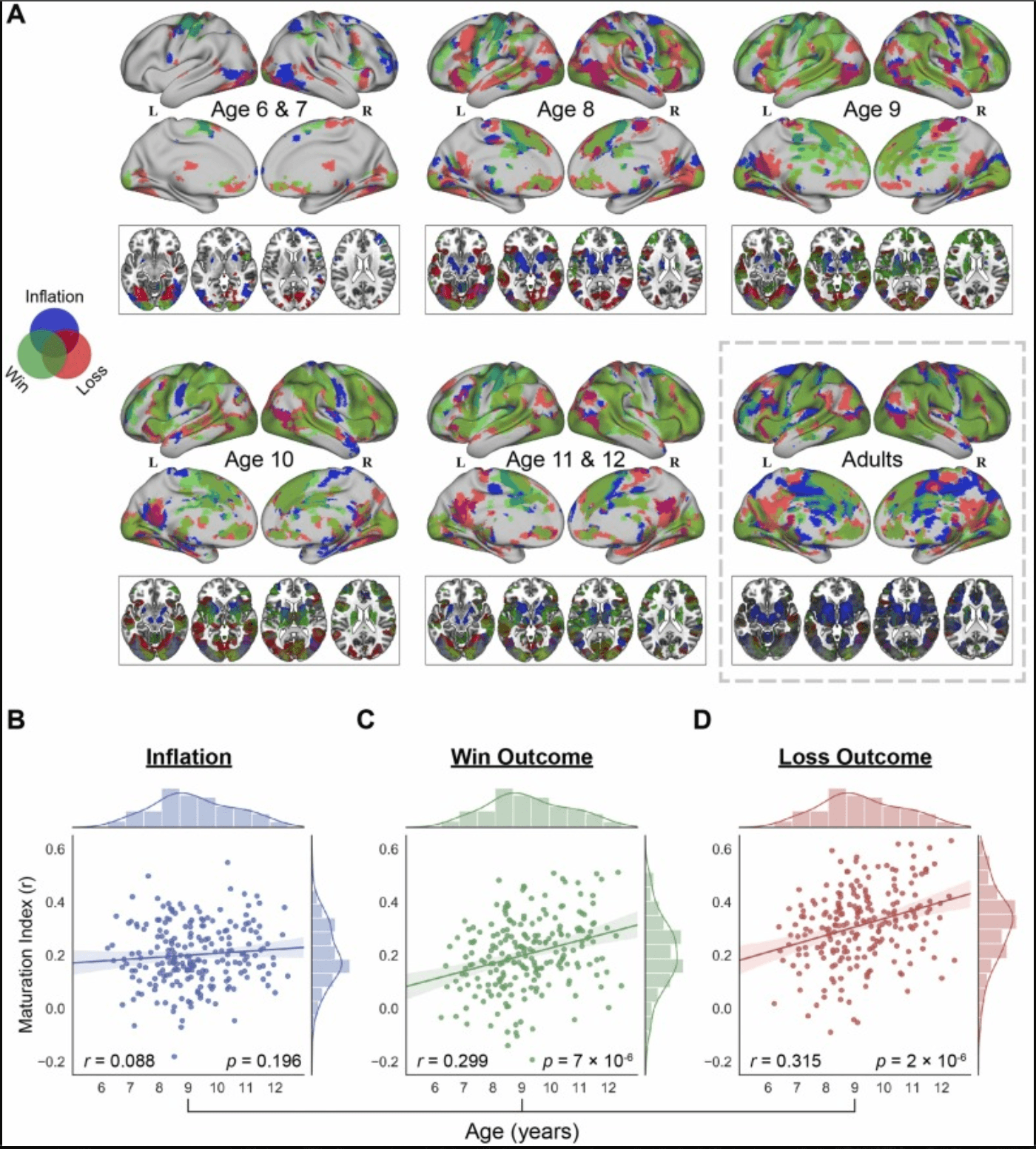
Fig. 2. Age-related differences in brain systems engaged in three core processes during risky decision-making. (A) An overview of activation patterns under the “Inflation” (shown in blue), “Win Outcome” (shown in green) and “Loss Outcome” (shown in red) conditions in different age groups. Axial slices (z = −10, 0, 10, 20) are displayed for reference. Overlaps between these conditions are shown in corresponding colors. (B-D) Age-related differences in brain activation under the “Inflation”, “Win Outcome”, and “Loss Outcome” conditions in child groups from 6 to 12 years old. Colored line indicates the best linear fit, and shaded area indicates 95% confidence interval. Marginal histograms indicate the distribution of age and maturation index. Notes: L, left; R, right.
2.8. Multivariate maturation index
To examine age-related differences in task-related neural representation patterns between child groups from 6 to 12 years old, we computed an overall multivariate maturation index for each of the three conditions (Kriegeskorte et al., 2008, Zhuang et al., 2022), which could assess the degree of neural activity pattern similarity in each child relative to the mature template of corresponding neural activity pattern averaged across adults. Condition-related independent brain masks were generated from activated brain regions of adults under the three conditions respectively, in which significant clusters were determined by using a stringent threshold of q < 0.05 (cluster size > 30) false discovery rate correction for multiple comparisons. Next, condition-specific multivoxel pattern vectors were extracted from corresponding brain masks in each child, and averaged neural activity patterns for each condition were created by averaging corresponding pattern vectors across adults. Then we calculated the maturation index represented by Pearson correlation between condition-specific pattern vector in each child and corresponding averaged pattern vector across adults. Subsequently, for each condition, the maturation index was entered as a dependent variable, and the age was entered as an independent variable into a linear regression (Fig. 2B-D). As demonstrated in Fig. S9, the unbalanced number of participants included in each age group would introduce the problem of narrower range of variables of interests. To clarify the possible impact of such issue on the above analyses, a Stratified Bootstrapping Analysis was subsequently conducted using the self-edited script based on Python (https://github.com/psychRay/BART_supplement_analyses/tree/master ): the same number of participants extracted from each age group were combined into a new sample and correlation coefficient between maturation index and age was calculated in it, which was replicated with 10000 times and returned a distribution of correlations. And the significance of correlation was judged based on whether 95% confidence intervals of correlation distributions include zero value (i.e., equals to two-tailed p < 0.05). Furthermore, to offer a better view of brain-behavior associations, the simple Pearson correlations among maturation indexes and behavioral variables were calculated (Supplementary Table S3 & S4).
2.9. Regions of Interest analysis
To characterize age-group differences in brain engagement in risky decision-making, at the group-level, we ran complementary ROIs analyses focusing on the Triadic Neural Systems Model, which provide a theoretical framework for the neuroscience researches on motivated behaviors (Ernst, 2014, Ernst and Fudge, 2009, Richards et al., 2013). And the neural substrates of the triadic modules are summarized in Table 1 (i.e., Control: dorsal anterior cingulate cortex (dACC; we extracted parameter estimates from different subregions of anterior cingulate cortex, and found age-related differences in the dorsal part), dorsolateral prefrontal cortex (dlPFC), ventromedial prefrontal cortex (vmPFC); Approach: nucleus accumbens (NAc), caudate, putamen; Avoidance: amygdala, insula, hippocampus). These ROI masks were defined based on the anatomical templates of the corresponding nine regions by using multiple atlases integrated in the WFU PickAtlas toolbox (Maldjian et al., 2003) (https://www.nitrc.org/projects/wfu_pickatlas ) and the NeuroSynth meta-analysis database (Yarkoni et al., 2011) (https://www.neurosynth.org ), which also helped to avoid the possible overlaps and inconsistencies caused by using single atlas. Specially, for dACC, dlPFC and vmPFC, the corresponding meta-analysis maps associated with them were first generated using “dacc”, “dlpfc” and “vmpfc” as terms in the NeuroSynth database, and then three ROI masks were defined as the overlap between these maps and anatomical templates of anterior cingulate, middle frontal gyrus and medial frontal gyrus, respectively. NAc, caudate and putamen were anatomically defined using the Individual Brain Atlas Statistical Parametric Mapping (IBASPM) templates (Alemán-Gómez et al., 2006). Amygdala, insula and hippocampus were anatomically defined using the Automated Anatomical Labeling (AAL) atlas (Rolls et al., 2015, Tzourio-Mazoyer et al., 2002) (Fig. 3A). In addition to ROIs separately defined at a single-region-level, separate regions constituting each functional module were merged into one unified mask respectively to form the module-level ROIs. Specifically, the dACC, dlPFC and vmPFC masks were merged into the Control module ROI; the NAc, caudate and putamen masks were merged into the Approach module ROI; the amygdala, insula and hippocampus masks were merged into the Avoidance module ROI (Table 1), by using the Image Calculator function in SPM12.
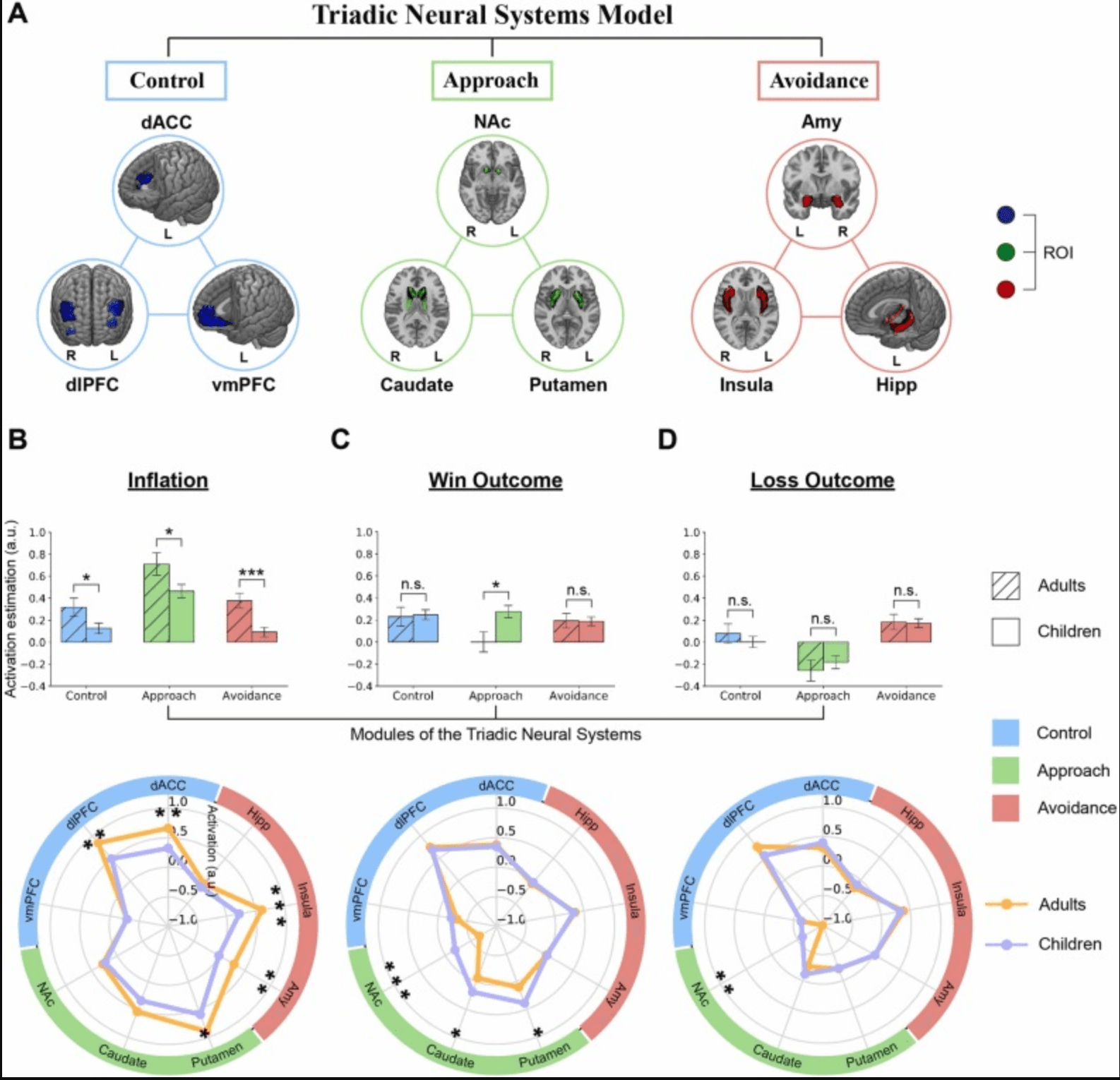
Parameter estimates (or β weights) associated with the three conditions of interest were extracted from these ROIs and averaged across voxels within each region (module-level and single-region-level), and were subsequently submitted for statistical testing based on the MATLAB platform. Separate contrasts on group differences (adults vs. children) were performed at both module-level and single-region-level to investigate age-related differences in brain activation patterns underlying different risky decision-making processes, the results of which were visualized using polar graphs as well as bar graphs (Fig. 3B-D & Fig. S5).
2.10. Task-dependent functional connectivity analysis
Task-dependent functional connectivity was investigated using the psychophysiological interaction (PPI) analysis based on SPM12, which examined condition-specific modulation of functional connectivity of a specific ROI with the rest of the brain, after removing potentially confounding influences of overall task activation and common driving inputs. Consistent with ROIs analyses, seed regions of the Triadic Neural Systems Model were defined as the anatomical templates of the corresponding brain regions (Table 1; i.e., Control: dACC, dlPFC, vmPFC; Approach: NAc, caudate, putamen; Avoidance: amygdala, insula, hippocampus). And under each of the three task conditions (Inflation vs. Win Outcome vs. Loss Outcome), separate whole-brain PPI analyses were conducted with each of the nine ROIs as a seed. The mean time series from these seed ROIs were deconvolved to uncover neuronal activity (i.e., physiological variable) and multiplied with the task design vector (i.e., a binary psychological variable) to form a psychophysiological interaction vector. And this interaction vector was then convolved with a canonical HRF to form the PPI regressor of interest. To remove overall task-related activation and the effects of common driving inputs including head motion parameters on brain connectivity, we also included the psychological variable representing the task conditions as well as the mean-corrected time series of the seed ROIs in the GLM. Brain regions showing significant PPI effects were determined by testing for a positive regression slope of the PPI regressor, which suggested significant variations in the brain response can be explained by the contribution of the psychophysiological interaction (Cole et al., 2013, Friston et al., 1997) and further formed the connectivity matrices.
Connectivity matrices corresponding to PPI effects under the “Inflation”, “Win Outcome” and “Loss Outcome” conditions at the individual-level were then entered into functional network analyses (Yu et al., 2019). Network connectivity was calculated within the three functional modules defined by the Triadic Neural Systems framework, as well as between all pairs of the three modules under the three conditions of BART (Fig. 4A), as follows:
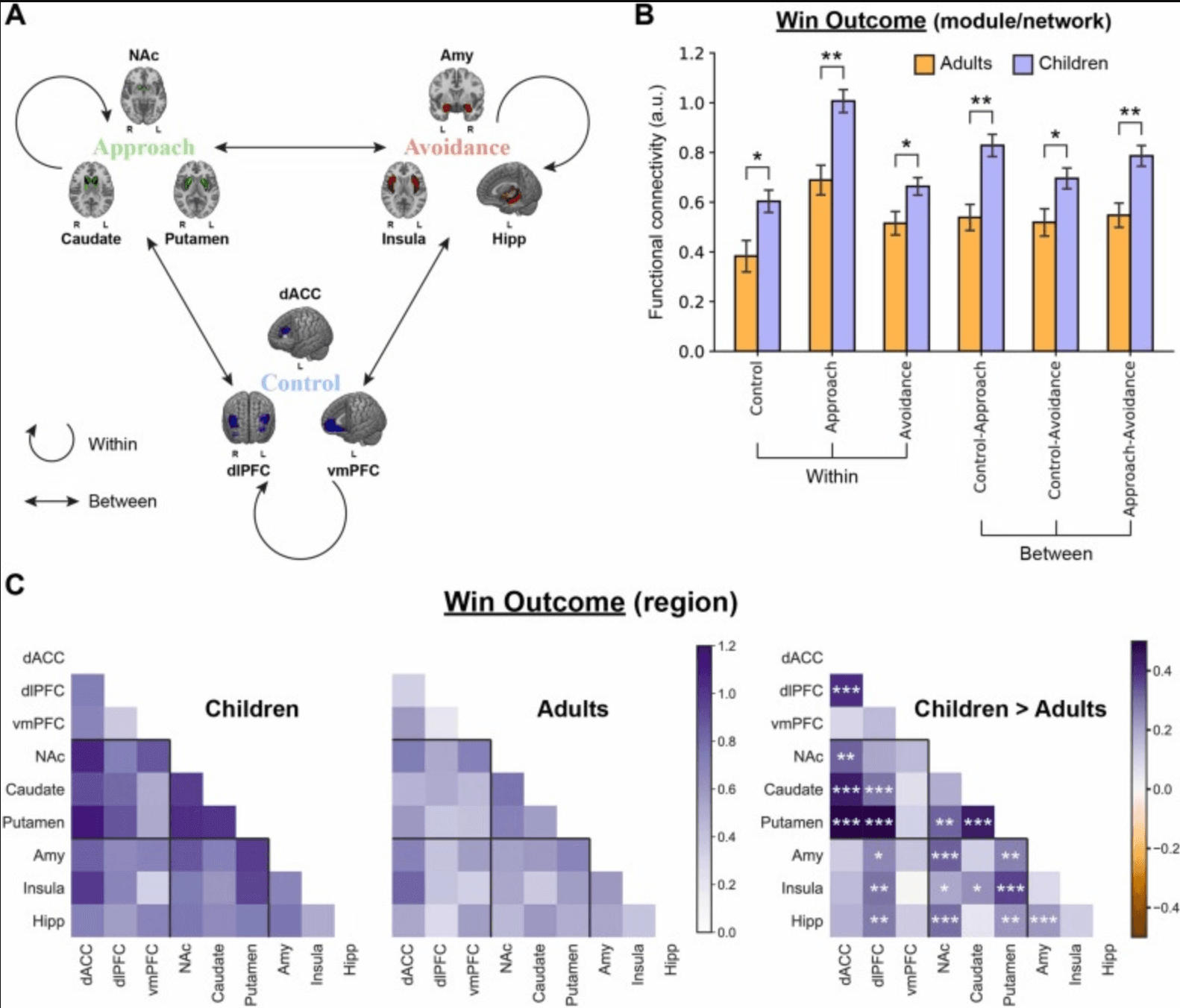
Fig. 4. Age-related differences in brain network connectivity between children and adults under the “Win Outcome” condition. (A) Within-network connectivity was computed within each module of the Triadic Model, and between-network connectivity was calculated between all pairs of these modules. (B) Differences in within-network connectivity and pairwise between-network connectivity between children and adults. (C) Reward-related functional connectivity patterns of children (left) and adults (middle), as well as their differences (right). Notes: L, left; R, right; a.u., arbitrary units; *p < 0.05; * *p < 0.01; * **p < 0.001; Error bars, s.e.m.
Within-network connectivity (Wa) for each module {a∈1,2,3} was computed as the average connectivity across all the links within the functional module (values along the diagonal of the matrix were not included), as follows:
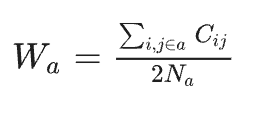
where Cij is the value in the 9 × 9 connectivity matrix; Na is the number of nodes within module a; i and j denotes different ROIs (i ≠j ).
Pairwise between-network connectivity (Pa−b) was computed as the average connectivity across all the links between two modules, a and b {a,b∈1,2,3; a≠b}, as follows:
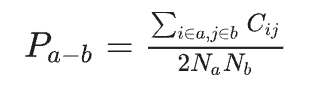
Subsequently, within-network connectivity and pairwise between-network connectivity were compared across groups (adults vs. children) using two-sample t-tests based on the MATLAB platform (Fig. 4B & Fig. S6). To offer more details and as a supplementary support for above analyses, we further conducted tests between children and adults at ROI-ROI level to investigate the specific connectivity which may contribute to the observed age-related differences (Fig. 4C, Fig. S7 & S8). And the significant results were identified using false discovery rate correction at q< 0.05.
3. Results
3.1. Age-related differences in behavioral performance of risky decision-making
We first examined age-related differences in behavioral performance during the Balloon Analogue Risk Task between adults and children. Independent t tests for average adjusted pumps, total earnings and proportion of explosion revealed that children had less average adjusted pumps (t′ < −13.22, P < 0.001; Z = −9.745, P < 0.001) (for comparisons failing the Levene's Test for Equality of Variances, results from both t′ test and Mann-Whitney U test are provided), less total earnings (t′ < −7.99, P < 0.001; Z = −7.152, P < 0.001), and lower proportion of explosion (t′ < −8.46, P < 0.001; Z = −7.339, P < 0.001) than adults (Fig. 1B).
To further characterize age-related differences of risky decision-making behaviors from 6 to 12 years old, we implemented a GAM to search for linear or non-linear relationships between age and behavioral measures. This analysis revealed significant increases in average adjusted pumps (P < 2.7 ×10−7), total earnings (P < 5.1 ×10−9) and proportion of explosion (P < 4.3 ×10−4) as a function of age (Fig. 1C). Additionally, children showed slower response (i.e., longer reaction time) in risky decision-making relative to adults (t296 > 6.47, P < 0.001) (Fig. S1A), and tend to become faster with the increase of age (P < 1.8 ×10−5) (Fig. S1B). To offer an overall view of associations between behaviors of risky decision-making and ages, the correlations among variables of interests (including age) were calculated separately in child and adult samples (Supplementary Table S3 & S4). The additional comparisons of age-related differences revealed no significant age-behavior correlations in adults but supported the above age-related increases in children, which to some extent provides evidence for gradual improvements in risky decision-making during childhood. These results indicate that children are generally less reward-seeking, less risk-taking and satisfied with relatively lower rewards compared to adults. With the increase of age, children tend to pursue higher rewards and become more risk-taking.
3.2. Age-related differences in brain systems involved in risky decision-making
Next, we investigated age-related differences in brain systems engaged in risky decision-making under three conditions of the BART in children compared with adults. As shown in Fig. 2A, there were noticeable changes in task-evoked univariate activations related to the conditions of “Inflation”, “Win Outcome” and “Loss Outcome” in different age groups. Taking trial number of each condition as covariates did not alter the main results, in which only group of lower age (6 & 7 years-old) was to some extent influenced (Fig. S4).
To quantify these age-related differences in brain systems underlying risky decision-making, we calculated an overall multivariate maturation index for each of the three conditions in children. This index is represented by the similarity between each child’s specific activation pattern vector and the averaged activation pattern vector for the corresponding condition in the adult brains. Subsequent linear regression analyses revealed no significant age-related difference in the maturation index (r = 0.088, P = 0.196) for the “Inflation” condition (Fig. 2B), but significant age-related increases in the maturation indexes for the “Win Outcome” (r = 0.299, P = 7 ×10−6) and “Loss Outcome” (r = 0.315, P = 2 ×10−6) conditions (Fig. 2C, D). To clarify the possible bias introduced by unbalanced number of participants included in each age group, the additional Stratified Bootstrapping Analysis was performed. And the results demonstrated the consistency with the above analyses (Fig. 2B-D), in which significant correlations between maturation indexes and age under the “Win Outcome” (95% CI of r: [0.083, 0.487]) and “Loss Outcome” (95% CI of r: [0.132, 0.519]) conditions were identified and no significance under the "Inflation" condition were found (95% CI of r: [−0.143, 0.283]) (Fig. S3). Furthermore, the results of correlation analyses among maturation indexes and behavioral variables (Supplementary Table S3 & S4) revealed significant positive associations between maturation index under the "Loss Outcome" condition and total earnings, and negative associations between maturation index under the "Inflation" condition and reaction time. The former shows that greater similarity of multivariate activity under the "Loss Outcome" condition with adults may contribute to more earnings during risky decision-making, which further indicates the age-related improvements in learning from failures. And the latter negative correlation provides additional evidence for the age-related increases in adaptive risk-taking behaviors.
These results indicate that distributed and largely overlapping brain regions, which demonstrate age-related differences, support the complex process of risky decision-making in children. Taking the adult pattern as the reference of maturity, the neurodevelopment related to risky decision-making from 6 to 12 years old is characterized by increasingly mature activation patterns associated with “Win Outcome” or “Loss Outcome”, but no significant age-related difference of activation patterns involved in the “Inflation” processes requiring the engagement of cognitive control, which may partly result from the protracted development of control-related brain regions during this period.
3.3. Weaker engagement in control-related brain systems, but stronger engagement in reward-related systems during risky decision-making in children than in adults
Based on the Triadic Model framework, we further examined how children and adults differed in neural activities involved in risky decision-making under the three conditions of the BART. Nine regions of interest, core nodes of the three functional modules constituting the Triadic Neural Systems Model, were defined by using the anatomical atlases (Fig. 3A). Three regions from each module were merged into one respectively to form module-level ROIs. Activation estimates extracted from these ROIs were then entered into group level statistical analyses for each condition.
Compared with adults, children showed weaker activation in all of the three functional modules under the “Inflation” condition (Control: t296 < −2.01, P < 0.045; Approach: t296 < −2.06, P < 0.040; Avoidance: t296 < −3.43, P < 0.001; Fig. 3B upper). Significant regions included the dACC (t296 < −2.80, P < 0.006) and dlPFC (t296 < −2.70, P < 0.008) in the control module, putamen (t296 < −2.25, P < 0.025) in the approach module, amygdala (t296 < −3.00, P < 0.003) and insula (t296 < −3.80, P < 0.001) in the avoidance module (Fig. 3B lower). Under the “Win Outcome” condition, however, children exhibited significantly higher activation only in the approach module (t296 > 2.57, P < 0.011) than adults, which consists of the NAc (t296 > 4.04, P < 0.001), caudate (t296 > 2.30, P < 0.023), putamen (t296 > 2.36, P < 0.019) (Fig. 3C upper & lower). Under the “Loss Outcome” condition, there was no reliable difference in the activation of three functional modules between children and adults (Fig. 3D upper), while subsequent analyses revealed stronger activation of NAc in children (t296 > 3.28, P < 0.002; Fig. 3D lower). These results indicate that, compared to adults, the Triadic Neural Systems Model in children is characterized by weaker regulatory controls of the prefrontal cortex, but stronger reward-related striatal systems.
3.4. Stronger functional connectivity of reward-related brain networks in children than adults
Focusing on the three modules of the Triadic Neural Systems Model (Control: dACC, dlPFC, vmPFC; Approach: NAc, caudate, putamen; Avoidance: amygdala, insula, hippocampus), we investigated age-related differences in functional connectivity patterns during risky decision-making by using whole-brain PPI analyses. First, separate contrasts were run to examine differences in the means of within- and between-network connectivity between children and adults under the three conditions of the BART (Fig. S6). We found that these brain systems only exhibited significantly different connectivity patterns under the “Win Outcome” condition, characterized by stronger network connectivity in children (Fig. 4B & Fig. S6B). There was no reliable difference between children and adults in within- and between-network connectivity under the “Inflation” (Fig. S6A) or “Loss Outcome” (Fig. S6C) condition. We further sought to explore which seed region’s connectivity with other brain areas in the Triadic Model accounted for the detected group differences.
Within-network connectivity for each functional module was computed as the average connectivity across all the links within the module (Fig. 4A). Compared with adults, children showed significantly higher within-network connectivity in all of the three functional modules under the “Win Outcome” condition (Within Control: t296 > 2.652, q < 0.013; Within Approach: t296 > 3.762, q < 0.002; Within Avoidance: t296 > 2.285, q < 0.024) (Fig. 4B), and the greatest difference was observed in striatal regions, characterized by stronger NAc-putamen (t296 > 3.237, q <0.005) and caudate-putamen (t296 > 5.065, q <0.001) connectivity (Fig. 4C right). There was no significant difference in within-network connectivity between children and adults under the “Inflation” (Fig. S6A upper) or “Loss Outcome” (Fig. S6C upper) condition. Pairwise between-network connectivity was defined as the average connectivity across all pairwise links among nodes of the three modules (Fig. 4A). Compared with adults, children exhibited stronger connectivity between all of the three pairs under the “Win Outcome” condition (Control-Approach: t296 > 3.596, q < 0.002; Control-Avoidance: t296 > 2.311, q < 0.024; Approach-Avoidance: t296 > 3.167, q < 0.004) (Fig. 4B) (more details regarding ROI-ROI connectivity are provided in supplementary Fig. S7 & S8). And there was no reliable group difference under the “Inflation” (Fig. S6A lower) or “Loss Outcome” (Fig. S6C lower) condition. These results indicate that children exhibited stronger reward-related brain network connectivity than adults, especially for functional connectivity within the Approach module and cross-network connectivity between Control and Approach modules (i.e., PFC-striatum).
4. Discussion
In this fMRI study, we investigated age-related differences in three neurocognitive systems underlying risk decision-making during middle to late childhood. Behaviorally, children exhibited age-related increases in risk-taking behaviors measured by adjusted pumps, total earnings and proportion of explosions during the task from 6 to 12 years old. At neuroimaging level, brain regions engaged in reward and risk processing significantly developed with age during childhood and exhibited a progressive approximation towards adults as revealed by multivariate maturation index, which partly accounts for children’s increasing risk-taking propensities. Critically, in comparison with adults, children exhibited weaker activation in prefrontal control system, but stronger activation in approach-related striatal systems. Moreover, greater reward-related functional connectivity within and between these brain systems was observed in children than in adults. These findings highlight heterogenous age-related differences in three neurocognitive systems involved in risky decision-making during childhood, and suggest that immature and unbalanced triadic neural systems may underlie risk-taking behaviors in children.
4.1. Age-related characteristics of risk-taking behaviors during childhood
Behaviorally, children tended to pursue higher rewards and exhibit more risky behaviors with the increase of age, which to some degree supports the view that increased risk-taking is a consequence of normative development (Crone et al., 2016), at least from 6 to 12 years old. Given that the risky behaviors dramatically rise and peak in adolescence (Burnett et al., 2010, Casey and Jones, 2010, Figner et al., 2009, Galvan, 2010, Paus et al., 2008) and are subsequently improved in adulthood through learning (Humphreys et al., 2016), we speculate that risky behaviors may increase early from childhood to adolescence, which is in line with the epidemiological findings (Rosenbaum and Hartley, 2019, Steinberg, 2013). In addition, children exhibited less risk-taking with less pumps relative to adults, which partly contradicts previous studies reporting children’s stronger risk preference (Paulsen et al., 2011). Such contradiction may be derived from the modulations of risk-taking by factors like task demands, age range and cultural differences (Defoe et al., 2015). This is also consistent with the findings showing that children until age 12 do not exploit advantageous options when processing risky reward (Van Duijvenvoorde et al., 2012).
4.2. Unbalanced functional organization of the triadic neural systems in children
At the brain activation level, multivariate maturation indexes based on whole-brain activity patterns provide new insights into how child’s individual pattern of risky decision-making develops relative to the matured template defined by brain activity patterns in healthy adults. Specially, we divided the BART into three conditions (i.e., “Inflation”, “Win Outcome”, and “Loss Outcome”) in view of the complexity of risky decision-making processes (Blakemore and Robbins, 2012, Guassi Moreira et al., 2021). As revealed by the maturation index, brain activity concerning reward- and risk-feedback demonstrated continuous development, but no significant age-related difference was found in brain activity patterns under the “Inflation” condition during which the engagement of control-related brain regions was needed. This finding may suggest a relatively protracted development of brain systems involved in cognitive control for risky decision-making. Taken together, such unbalanced development may contribute to a gradual increase in risky behaviors during childhood. Notably, this finding coincides with the theoretical models which postulate that risk-taking in the following adolescence is driven by the relatively slower development of self-control capacities (Casey and Jones, 2010, Ernst and Fudge, 2009). The Triadic Neural Systems Model (Ernst et al., 2006) and the dual-system imbalance models (Casey et al., 2008, Li, 2017), derived from empirical studies focusing on human neurodevelopment and translations across species, have also suggested that unbalanced development of prefrontal control and limbic regions may lead to a processing imbalance in motivated behaviors (Casey, 2015), from which the further speculation could be made that the behavioral propensity for risk-taking in children might be at least partly due to unbalanced functional organization of brain systems involved in risky decision-making.
Extending on prior findings that put forward the Triadic Neural Systems Model of motivated behavior in adolescence, we provide empirical evidence supporting the neurodevelopment of risk-taking onset early from middle and late childhood. Interestingly, under the “Inflation” condition when the prefrontal control module was urgently needed to balance reward against loss risk, we found yet children’s significantly weaker activation than adults in dlPFC and dACC, both of which play pivotal roles in saliency, regulatory control, attention and conflict (Amodio and Frith, 2006, Bush et al., 2000, Carter and van Veen, 2007). Under the “Win Outcome” condition during which the reward information was displayed, the significantly higher activations in the NAc, caudate and putamen within approach system were observed in children compared with adults, which coincides with the previous observation of enhanced responsivity of the striatal system to appetitive stimuli in early adolescence (Ernst and Hardin, 2008), together indicating that children demonstrated adolescent-like higher sensitivity to reward feedback and weaker control for reward-risk balance. As for the avoidance system, it manifests obvious differences between children and adults under the “Inflation” condition, but not under the “Win Outcome” or “Loss Outcome” condition. This might be partly due to adults’ better ability to learn from losses, in which the loss memory of previous trials and uncertainty evaluation will contribute to aversive learning during inflation. And such ability, evidenced by previous studies (Kuhnen and Knutson, 2005, Peter et al., 2010, van Duijvenvoorde et al., 2022, Wu et al., 2021), is largely dependent on brain activity in the amygdala (Maren, 2016, Sanford et al., 2017), insula (Hardin et al., 2009, Rauch et al., 2003) that constitute the avoidance system in the Triadic Neural Systems Model.
Together, these findings point toward unbalanced functional organization of the triadic neural systems underlying risky decision-making during childhood, which manifests a stronger and faster development of reward-related striatal systems and a weaker and slower development of control-related prefrontal systems. Such an adolescent-like pattern also partly strengthens the idea of using childhood indexes as the precursors and predictors of adolescence risk-taking (Crowley et al., 2017, Ernst and Fudge, 2009, Ernst et al., 2006).
4.3. Heightened reward-related functional coordination in children
At the brain network level, we also observed stronger within- and between-module functional connectivity of the triadic neural systems in children than adults, which may suggest immature functional coordination of brain regions underlying risky decision-making in childhood. The stronger functional coordination among regions within the approach system, revealed here by higher within-module connectivity in children, was demonstrated under the context of win outcome and is somehow in line with regional activation results, which offer both activation- and connectivity-level evidence supporting children’s higher sensitivity to reward. Additionally, by virtue of the BART paradigm, reward can only be acquired through stopping pumping the balloon and is directly linked to the inhibition of risk-taking, based on which our results revealed that stronger between-module (Control-Approach) connectivity in children under the “Win Outcome” condition may result from and even contribute to the successful inhibition of risky behaviors. Such pattern may be derived from the relatively slower functional specialization (within-module connectivity) of executive control network (Finn et al., 2010), maturation of which in adults bolsters the enduring monitoring of inflation process and controlling of pumping decision to achieve better risk-reward balance. From the cross-sectional view, that PFC-striatum connectivity at rest decreased with age from childhood to adulthood (Fareri et al., 2015) conforms to the above age-related differences in between-module connections and further strengthens our view. Notably, all of these age-related differences only existed under the “Win Outcome” condition, no significant group difference in within- and between-module functional connectivity was observed under the “Inflation” and “Loss Outcome” conditions in our present study. Viewed in connection with children’s higher striatal activation under the “Win Outcome” condition displaying reward-related information, the approach system consisting of striatal regions may have a relatively dominant position in the functional coordination of these systems in children, which further contributes to these condition-specific connectivity patterns.
Taken together, our above findings suggest that children, who are more sensitive to reward feedback and poorer at reward-risk balance than adults, have immature functional coordination of the triadic neural systems underlying risky decision-making.
4.4 Limitations
Our findings should be interpreted in the context of limitations and tradeoffs in our experimental design. First, our present study with cross-sectional design focused on children of age-range from 6 to 12 years old instead of covering the entire period from childhood to adulthood, and mainly investigated age-related differences based on “adults vs. children” contrasts, which limited its ability to discuss mechanisms of development compared with longitudinal designs. Second, although children-friendly, our current task paradigm adapted from the original BART paradigm (Lejuez et al., 2002) did have some limitations including the inaccurate estimation of risk-taking (Pleskac et al., 2008, Young and McCoy, 2019) and the difficulty in dissociating different states of decision-making (Rao et al., 2008). Third, children and adults participating in the experiment were recruited from several homogeneous schools and communities, but some of personal traits (e.g., impulsivity, reward/risk preferences, sensation seeking) and socioeconomic factors (e.g., the average income) that may be associated with neural sensitivity to risky rewards (Dalley and Robbins, 2017, van Duijvenvoorde et al., 2022) were not strictly manipulated. These potential factors may limit the generalizability of our findings to a broader population. Future large-scale, longitudinal studies that can better simulate real-world risk situations (De Groot, 2020) and take more interferential factors into consideration will help provide a more comprehensive understanding of the life-span neurocognitive mechanisms underlying risky decision-making.
5. Conclusion
Our study sheds light on the neurobehavioral development of children’s risk-taking behaviors, and demonstrates immature and unbalanced functional organization of the triadic neurocognitive systems involved in risky decision-making during childhood, characterized by weaker prefrontal engagement but stronger reward-related striatal activation as well as brain network connectivity. Our findings establish a critical link between the unbalanced triadic neural systems and children’s risk-taking behaviors and provide implications into understanding the neurodevelopment of these systems.