INTRODUCTION
Mental states matter. Consequently, we and colleagues designed and executed a brain-imaging experiment attempting to detect—for the first time—differences between mental states relevant to criminal law.
Imagine you’ve just killed someone in Colorado. It was not your purpose or desire to kill him. Nevertheless, another human being is dead. Arrested and on trial, you do not dispute that your action unjustifiably caused his death. But whereas the prosecutor argues that you knew someone would die as an inevitable by-product of your actions, you assert in your defense that you knew no such thing. Instead (you claim) you were merely reckless. That is, you acted as you did with awareness of a substantial risk that someone would be fatally injured, but without knowing you would kill anyone.
In Colorado, as in many states, there is a huge difference in the sentencing ranges for those convicted of knowing and reckless homicides. In Colorado it means the difference between being sentenced to sixteen to forty-eight years in prison and none.1 So your fate rests in the hands of lay jurors who will decide what your mental state was at the time of the fatal act. Specifically: Did you know you would kill someone, or were you merely aware of a risk that you would?
Now, any plausible theory of the point or purpose of meting out punishment to offenders—whether utilitarian, retributivist or expressivist—will recognize good reasons to condition punishment, or its amount, on the offender’s mental state. Mental states matter to the nature and severity of incentives to which human behavior is sensitive, to moral desert, and to society’s collective outrage. But, whatever its rationale, the practice of predicating differences in punishment on differences in mental state means that you now face two large problems ignored by our current criminal justice system.
First, the criminal justice system simply assumes that most jurors can reliably distinguish between the two mental states at issue. It is well known to all first-year law students that the supermajority of states follows the Model Penal Code’s long-standing approach to categorizing culpable mental states into four types: purposeful, knowing, reckless, and negligent. Large numbers of offenses, including homicides, are then subdivided into corresponding categories. And the extent of legal intervention—length of prison stay, for instance—scales accordingly. But less well known is that a body of experimental evidence suggests that jurors are not particularly good at understanding which category is which.2 Subjects frequently get it wrong, even when directly instructed on the relevant legal definitions and standards. They find it particularly difficult to sort defendants between the Model Penal Code’s categories of “knowing” and “reckless.” They confuse the two about 50% of the time, under some conditions, and do little better under others.3 In such a case, that’s nearly coin-flipping odds of false conviction.
The other big problem is that no one knows if the legally assumed and statutorily instantiated distinction between knowing and reckless mental states reflects an actual and inherent psychological difference. Sure, we might all believe such a difference exists, on the basis of introspection alone. But introspection seems an insufficiently sound basis on which to lay the very foundations for policy distinctions of such large consequence. The supposed distinction between knowing and reckless could be nothing more than a convenient fiction, upon which countless trials—and far more plea bargains—have been built.
To see the bite of this second problem, first consider the way in which Legal Realists have approached the mens rea categories. The Legal Realist tradition is well-known for the claim that many legal terms and concepts falsely purport to classify defendants and their circumstances on the basis of their intrinsic features.4 Instead, assert the Realists, defendants are classified on the basis only of the judge’s desire to hold some liable and to decline to hold others liable, even when those two groups of people do not differ in any way other than in the eye of the judge.5 But then note that this critique has extended to juries as well. That is, the Legal Realists claim that the question of whether you were knowing or reckless when you performed the act that killed someone only purports to be a question about your psychology.
The important, long-lingering question—as relevant to retributivists as to utilitarians—is therefore this. Does the distinction between Model Penal Code mens rea categories, such as knowing and reckless, reflect an intrinsic psychological difference, actually found in human beings? If so, we believe that one should expect in principle that there would also be a difference between the brains of reckless and knowing individuals, at the times of their actions. Because, after all (and setting aside some philosophical subtleties6) anytime there is a psychological difference there must also be a brain difference.
So is there a neural difference or not? Regardless of where legislators choose to draw the lines between different mental states, and regardless of how many categories they create, those categories should reflect something real, and not merely assumed or hoped-for. Because the lives and liberties for thousands, each year, depend on the mental state category assigned to them.
So if we have used the supposed distinction between knowing and reckless (and other Model Penal Code categories) to justify a different treatment under the law, when there is in fact no detectable or meaningful psychological distinction, then widespread injustice will have followed in the wake of the Model Penal Code, and will continue indefinitely, if unchecked.
With a grant of nearly $600,000 from the MacArthur Foundation Research Network on Law and Neuroscience7 (Research Network), we—as part of a larger interdisciplinary team8—set out to investigate the knowing-reckless distinction in the brain, and the boundary that may separate them. Specifically, we aimed to see if we could use brain activity alone to detect the difference between those who the law would classify as “knowing” and as “reckless.” By combining the relatively new technical achievements of functional magnetic resonance imaging (fMRI) with new advances in the analytic abilities of machine-learning algorithms (a form of artificial intelligence) our team conducted the first assault on this thorny legal problem.
This Essay reports and describes, for a legal audience, the results and implications of our experiment. We found evidence strongly supporting the existence of a brain-based distinction between knowing and reckless mental states. Our detailed neuroscience paper was first published in a dedicated peer-reviewed science journal, the Proceedings of the National Academy of Sciences.9 It received some sensationalist press coverage, including headlines such as this one from the British Daily Mail: Something On Your Mind? AI Can Read Your Thoughts and Tell Whether You are Guilty of Committing a Crime.10 Half-truths like these are dangerous. So our goal here is to explain for a legal, non-scientific audience what we did and—more importantly—how it does and does not matter for the law.
Our team’s discovery is relevant to law in two ways.11 First, it provides new information relevant to the substantive debates over the accuracy and legitimacy of the distinction the Model Penal Code draws between knowing and reckless mental states. In this one important domain, that is, there is reason to think that what the law purports to do—draw a distinction in liability on the basis of a distinction in psychological state—is what it actually does. Second and collaterally, our results serve as a concrete and salient example of how new neuroscientific techniques, sometimes partnered with artificial intelligence tools, can be used to probe matters of legal relevance.
We proceed in three primary parts. Part I provides an overview of the experiment and the results. Along the way, it offers a necessary but brief and accessible introduction to how fMRI brain imaging works. Part II discusses the important implications of this new finding. Part III provides necessary caveats and cautions, to ensure that our results won’t be over- or misinterpreted.
I. DETECTING MENS REA IN THE BRAIN
A. Background: Initial Obstacles
The central challenge was to develop an experimental paradigm that could elicit states of mind that legal scholars and the legal system in general would consistently classify as knowing and reckless. So suppose two different scenarios in which a person takes something that does not belong to him without permission. In both scenarios, our defendant emails his neighbor to ask permission to borrow that neighbor’s car. Assume further that, in the past, the neighbor has said yes to this request about half the time, and about half the time has said no.
In the first scenario, our defendant checks his email and sees that the neighbor has said no. Then the defendant borrows the neighbor’s car anyway, knowing full well that he does not have permission to do so. He figures (incorrectly as it turns out) that the neighbor won’t even notice. In the second scenario, our defendant never checks his email for a reply, and therefore never sees that the neighbor has said no. Then the defendant goes ahead and borrows the car anyway. He figures there’s about a 50% chance he has permission, and he also figures (incorrectly) that either way the neighbor will probably never notice. The Model Penal Code would classify the first defendant as liable for a knowing theft and the second as liable for a reckless theft.
As the example makes plain, a central component of the distinction between knowing and reckless mental states, under the Model Penal Code regime, is that a person in a knowing state of mind is essentially 100% certain about the presence of an element of a crime. In contrast, a person in a reckless state of mind can have a belief about the probability located within a range— not so low as to promise a de minimis expected harm, but not so high as to be functionally equivalent to certainty. So what we needed was an experiment in which subjects would sometimes choose to perform an act while knowing a certain condition was in place, and sometimes choose to perform the same act while aware, instead, of a risk high enough to potentially qualify as “substantial and unjustifiable” while still far enough below 100% as to fall short of knowledge.
B. The Paradigm: Eliciting Knowing and Reckless Mental States
We asked our subjects to imagine that they were given an opportunity to carry a briefcase across the border.12 The briefcase might or might not contain “valuable content” (such as documents or microchip processors), which we refer to as “contraband.”13 And a carried briefcase might or might not be searched at the border.
There was a significant financial incentive to choose to carry the briefcase. Specifically, subjects who could get a briefcase across the border without being searched could leave the lab with quite a lot of money (sixty dollars, on top of the twenty dollars they earned for participating). But getting caught at the border carrying the contraband resulted in a financial penalty (the subject could leave with nothing more than the fee for participating). The other two options—getting “caught” with an empty briefcase or crossing successfully with an empty briefcase—had payoffs in between.
What we were primarily interested in was the differences between our subjects’ brain activity when they were certain that the briefcase contained contraband and their brain activity when there was some “substantial and unjustifiable” risk that it did. While subjects made decisions about whether to carry briefcases across the border, we scanned their brains.
We instructed subjects before the game began on the details of the payoff structure, as illustrated in Figure 1. Subjects began each of the 125 trials they completed with a hypothetical $6,000 in the bank. The payoff structure then governed how much a subject could earn or lose from the intersection of her choice (carry or don’t carry) with two variables (1) the probability that a carried case contained contraband (the Contraband Risk); and (2) the probability that a carried case would be searched (the Search Risk).
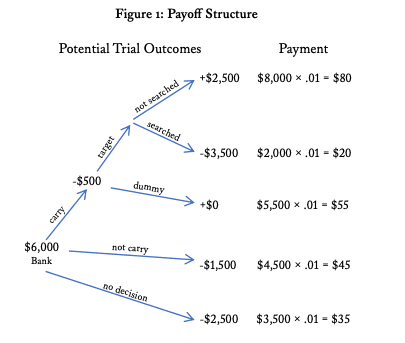
Specifically, a subject gained $2,000 (in her virtual bank account) each time she carried a case containing contraband through a checkpoint unapprehended. But she lost $4,000 from that account if she carried a case with contraband and got caught. If she carried a case that ultimately contained no contraband, she lost $500, regardless of whether her case was searched. To incentivize the subjects to make a choice, especially the choice to carry, we added two more fees: choosing not to carry cost $1,500 and refusing to choose whether or not to carry cost $2,500. We also informed subjects that if they completed the experiment they would receive 1% of the payout from one trial, chosen at random. Subjects could take this 1% home in cash. Given the details of the payoff structure, each subject therefore received between twenty and eighty dollars for participating. These payoff details are illustrated in Figure 1.
At the beginning of a trial, we provided each subject with two pieces of information. We informed them of the Contraband Risk and the Search Risk. To communicate Contraband Risk, we showed the subject a picture of one, two, three, four, or five cases (shown collectively in Figure 2)—only one of which ever contained contraband. We informed subjects that (1) this was the pool of cases from which the subject’s own case would be chosen; and (2) one, and only one, briefcase in that pool contained contraband.
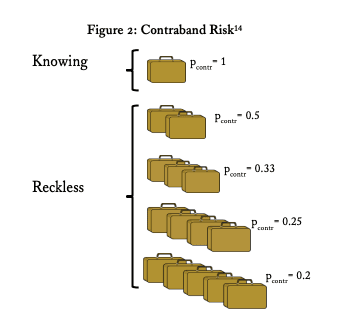
Thus, if the subject saw a picture of five cases then that subject could deduce that choosing to carry a randomly-assigned case in that trial yielded a one-chance-in-five (or a 20% likelihood) of getting a case with contraband. Conversely, if the subject saw only one case, and understood that only one case would contain contraband, that subject could deduce that choosing to carry that case yielded a one-chance-in-one (or a 100% likelihood) of getting a case with contraband. And so on for two, three, and four cases.
To signal the probability that a carried case would be searched, we showed each subject, on each trial, a picture of ten tunnel exits (shown collectively in Figure 3), some number of which—either two, four, six, or eight—showed a guard standing prominently in the exit. As with cases, subjects could readily calculate that the probability of being searched was much higher if there were eight guards (eight-chances-in-ten, or 80%) than if there were only two guards (two-chances-in-ten, or 20%).
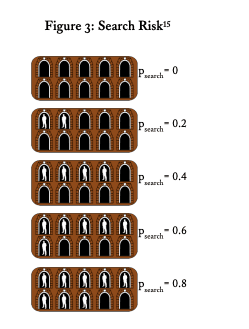
As a standard precaution, and to counterbalance any e)ect on our subjects of the order in which we showed the two images that signal these two pieces of information—Contraband Risk and Search Risk, respectively—half of the subjects always learned of the Contraband Risk before they learned the Search Risk. And the other half of the subjects always learned the Search Risk before they learned the Contraband Risk. As we learned, this proved important.
After learning these two pieces of information, and mindful of the payoff) structure, subjects were tasked with indicating whether they would be willing to carry a case through a tunnel. The subjects understood that, if they chose to carry, both the case and the tunnel would be selected at random from those presented. After subjects registered their choices to carry or not carry, there was a pause of a few seconds before the subjects started over in a new trial, with a new allotment of hypothetical money, new information, and a new choice whether to carry or not carry a case.16
C. Virtues of the Paradigm
This design has several virtues.
First, the paradigm clearly distinguishes between subjects who are in a “knowing,” as distinct from “reckless,” mental state. For the “knowing” condition, recall that we informed subjects that one and only one case would ever contain contraband. For this reason, whenever a subject chooses to carry the single case offered (i.e., a case that must contain contraband), we can reasonably believe that, absent inconsistent behavior to the contrary, she knows that the case she will carry contains contraband. In comparison, consider the mental state of the subject when she is presented with two, three, four, or %ve presented cases and chooses to carry. In those situations, we can reasonably believe that the subject is aware of the respectively varying degrees of probability that she is carrying the contraband, and is therefore in a “reckless” state of mind.
Second, because we did not explicitly inform our subjects of the risks— i.e. by describing those probabilities as “50%” or “1-in-2”—we mimicked an important feature of many real cases. Specifically, people in real-world situations ordinarily infer probabilities from evidence, rather than being presented with numeric information about probabilities. For instance, as someone decides whether to run the red light, there is no sign hanging in the air that says, “the probability of killing someone by running this red light is 19%.” Rather, one reaches a judgment about the probability by looking at the number of oncoming cars, their speed, and other similar factors. In the experiment, our subjects had to infer the two relevant probabilities from a picture of that round’s pool of cases, and a picture of tunnels (some fraction of which had guards in them). This is not, of course, the form that most evidence of probability takes in real life. But it is far closer than would be directly presented numerical information.
Third, varying the chance of being caught from 20% to 80% allowed us to mimic another feature of real cases: people who commit crimes often decide to do so in part by calculating the risks of being apprehended. Juries assessing mens rea, however, are never asked to determine what probability the defendant assigned to his being caught; it is not relevant to the mens rea inquiry. What matters is what probability (in lay, rather than statistical terms) the defendant assigned to legally-relevant elements of the crime, such as killing someone else, or not having permission to borrow, or there being drugs in the briefcase he was carrying. Controlling the information about the chance of detection boosted our ability to do what fact finders are asked to do: meaningfully distinguish between the awareness of the risk that is relevant to a recklessness assessment (namely the awareness of the risk that the case contained contraband) from the awareness of the risk of apprehension, which is not relevant to the question of recklessness.
Fourth, varying the pool of cases from one to five created the possibility that we might learn something about how brain states vary within the reckless mental state itself. That is, the brain states might vary as a function of the changing probabilities that one would be carrying contraband—from 20% (when five cases were presented) to 50% (when only two cases were presented).
We discuss important limitations to this experiment in Part III. But the core idea here is that collecting data on brain activity during each trial, and analyzing that data in conjunction with the varying behavioral outputs (i.e., choosing, each trial, whether or not to carry), should afford us some window on whether, and if so how, neural activity varies between knowing and reckless conditions.
D. Tools for Detecting Mens Rea
How, exactly, did we collect and analyze the data? This subsection provides a brief overview of how fMRI brain imaging works and how machine-learning algorithms assist in finding useful and predictive patterns in the data.
1. fMRI Brain Imaging
Prior to the invention of functional magnetic resonance imaging (fMRI) in the early 1990s, researchers had sophisticated tools (such as CT scans, using computed tomography) for measuring the physical structure of the brain, but somewhat limited tools (such as EEG and PET scans, using electroencephalography and positron emission tomography) for measuring brain function. fMRI significantly changed all that. First, fMRI enables strong inferences about neural activity within and across the entire brain. Second, fMRI is sufficiently noninvasive that it can be used on healthy people without surgery or injections.
Over the last twenty-five years, fMRI has become one of the world’s most dominant research tools for learning about brain function. Its details are both technical and elegant.17
At the big picture level, the fMRI process is like a bat’s echolocation. Similar to how a bat sends a wide high-frequency sound at small potential targets, and then makes strong inferences about their locations from the directions of sound reflected back, fMRI beams radio waves to the brain, and enables inferences from the differential patterns in energy that returns from within brain tissues. More specifically, fMRI allows researchers to discover and monitor both the locations of changes in blood flow, and the amounts of those changes, correlated with the different moments in each subject’s information-gathering, information-processing, and decision making tasks, as well as with the final decision itself.
Researchers place a subject on her back within a large tube that is surrounded by massive, super-cooled, super-conducting wire coils arranged to move electrical energy in a particular pattern. The coils are arranged to create a very strong magnetic field within the scanner that can be exquisitely manipulated (and even graduated in strength) along the axes of length, width, and height.
To understand how this works, you must also understand that: First, all atoms (including those in the body) contain some spinning particles, each bearing an electrical charge. Second, spinning objects with an electrical charge are, in themselves, tiny magnets. Third, placing a person within a strong magnetic field of an MRI tends to align the axes of spin of their subatomic particles, just as metal filings on paper will align with a field of a magnet held underneath.
Let’s now connect this to neurons in the brain. Neurons are the cells that carry electrical impulses from one end to another and that, by virtue of their interactions within the brain, enable everything from perception to decision to action. Like all cells, they need nutrients supplied by the blood, like oxygen, to live and function. The more active neurons are, the more oxygenated blood they need.
Which brings us to the happy fortuity that enables fMRI to discover things about brain function: oxygenated blood cells (which bring oxygen to the neurons) and de-oxygenated blood cells (which have already o)-loaded their oxygen to neurons) have different magnetic properties. The significance is this: when an MRI “pings” (so to speak) a brain in the scanner, certain subatomic particles that are all spinning in the same axis are temporarily bumped out of alignment. When the signal stops, and those subatomic particles snap back into alignment with the magnetic field, they release a certain amount of energy, which can be spatially located, in the brain, by an array of receivers in the MRI machine.
Because fMRI technology can detect changing ratios in oxygenated and deoxygenated blood, over both time and space, researchers can make inferences about where different brain regions are most and least active during each trial of the experiment. Researchers then compare that information either to a baseline of brain activity (the so-called “resting state,” when an awake brain is simply talking quietly to itself, without any specific task to perform, other than normal bodily functions) or to a contrasting set of decisions that task the brain in different ways. This enables researchers to learn about how the brain operated during the particular decisions they are studying.
Put another way, just as a bat can place a mosquito in airspace, on the basis of reflected sound waves, fMRI can detect increases and decreases, within brain space, in the ratio of oxygenated to deoxygenated blood. And this in turn enables strong inferences about where and when, in the brain, neurons are working harder.
2. fMRI in Our Experiment
Our experiment used fMRI technology, as just described, to scan a subject continuously as that subject (1) sees each scenario stimulus on a screen; (2) processes what it means; (3) makes decisions about whether or not to carry a case; and (4) registers that decision behaviorally by pressing one of two buttons with her fingers.
Neurons work harder when a person is seeing, processing information, deciding, and pressing a button than when the person is not engaged in these activities. That calls up more blood to deliver more resources. In the same way that transitioning from a jog to a sprint has our muscles calling up more oxygen and energy from the blood, neurons that are working harder call up more oxygen and energy as well.
Throughout the entire process through which our subjects assessed the probabilities and decided whether to carry the case across the border, the scanner recorded data from the entire brain about where, when, and how oxygenated and deoxygenated blood ratios were changing. Because we knew exactly what each subject was seeing when, and also knew exactly when and what the decision output was (i.e. to carry or not to carry), we could correlate different patterns of brain activity with the different probability combinations and with the different decisions each subject reported.
Each subject was in the scanner for about forty minutes. Since we measured changing oxygenated blood levels in tens of thousands of brain locations during that period, there were literally millions of pieces of data collected about each subject. To analyze those data, we deployed a form of artificial intelligence known as a machine learning algorithm.
3. The Machine Learning Algorithm
“Machine learning” describes a process by which a software program can “learn” the associations between various inputs, conditions, and outputs.18 In our case, the inputs are the brain data. The conditions are the separate risks, within each given trial of carrying contraband and being searched. And the outputs are the subjects’ choices whether or not to carry, given these conditions.
The core idea is that if you train the algorithm by showing it actual data from actual subjects, the software attempts to find the most common patterns within that data set. It can then use those patterns to predict how to classify the subject’s mental state, during a given trial, into a knowing or reckless state of mind, using brain data alone. The machine “learns” what patterns of brain activity are associated with being in a knowing mental state by comparing the fMRI data gathered when subjects were contemplating carrying a single briefcase. And the machine “learns” how those patterns differ from the brain activity associated with being in a reckless mental state by comparing them with the brain activity when the subjects were contemplating a pool of two, three, four, or five briefcases.
We used a particularly sophisticated algorithm, known as “the elastic net,”19 that learned not just from the data, but also from the failures and successes of other e)orts to learn from the data. We can clarify what that means with an analogy.
Imagine a teacher who, in her first year in the classroom, tries to teach her students to identify birds by showing them pictures. She puts a slide up on the screen and says, “Robin!” and then another and says, “Cardinal!” and then moves on to other slides of other species. Before her second year of teaching, she reviews the students’ performance from the first year and finds that some of the pictures she showed were more useful than others for teaching the students. The students were confused by some pictures and found others more helpful. Perhaps they did exceptionally well at identifying cardinals shown from the front, on their final exam, and there is only one picture in the stack of a cardinal from that angle. From that result, she concludes that one picture in the stack was particularly pedagogically useful. She repeats the process for other species and makes extra copies of the useful pictures, adding them to her stack.
Our hypothetical teacher then tries again the following year with a new group of students. They see all the original pictures, shown to the prior set of students, but the pictures that were useful last year they see more than once. The teacher reviews again. She finds that, even among the pictures she made extra copies of, some were exceptionally helpful to the students. She makes further extra copies of those and adds them to the stack, creating a new, even better stack to use for next year’s students. And so on. In her tenth year of teaching, she has a great stack of photos, far better than her first-year stack. The tenth-year students, as a result, are fantastic at identifying birds from pictures, much better than the first-year cohort.
Our algorithm learned in a way analogous to this and so became better and better at classifying knowing and reckless mental states across several generations. Algorithms that work this way are sometimes called “pattern classifiers.” And using such classifiers with respect to brain data is sometimes called “multi-voxel pattern analysis” or MVPA for short (where a “voxel” is like a three-dimensional pixel volume in the brain, such as a two-by-two-by-two-millimeter cube).
Another example makes clearer how this can work. Suppose we wanted to see if a machine learning algorithm could reliably determine whether a person whose brain was scanned with fMRI was looking, at the time the brain data in question were acquired, at a photo of a face, or a photo of a place.
We could feed the algorithm brain data from when a bunch of different subjects are seeing faces, and “tell” the algorithm, essentially: “These data are all from condition one, which we will call ‘faces.’” We could then feed the algorithm brain data from a bunch of subjects who were at the time seeing places and “tell” the algorithm “These data are all from condition two, which we will call ‘places.’” Then we could show the algorithm new unlabeled brain data from a single subject and ask it to determine, on the basis of di)erences it observes between the two conditions, whether this person was in fact looking at a face or a place at the time the brain data were acquired.
The greater the differences between the aggregate sets of condition one and condition two brain data, the better will be the algorithm’s ability to predict what the unknown subject was looking at. In laboratory conditions, when researchers actually know what this mystery subject was looking at, but are testing the effectiveness of the algorithm, the accuracy of that prediction can be quantified (such as, say, 89% accurate). The more accurate the algorithm, the more confidence researchers can have about the predictions the algorithm can make with respect to subjects whose stimuli are not known to researchers. Consequently, if researchers are using a training method like the elastic net, they can then use their degree of con%dence to alter their training method, emphasizing the particularly useful, and representative parts of the first round training data to retrain in the second round, in order to improve predictive power. And so on.
In like fashion, we first set our algorithm the task of predicting whether one of our research subjects was in a knowing or reckless mental state, during any particular trial in the scanner. Second, we set our algorithm the task of predicting whether a subject in a reckless mental state was seeing two, three, four, or five cases. Third, we set our algorithm the task of predicting how many guarded tunnels (representing search risk) the subject was seeing at a given moment. Finally, we set the algorithm to predict whether or not, given the brain data observed, a subject was about to choose to carry, or to decline to carry, the case.
4. Testing the Machine Learning Algorithm
Many statistical techniques can test the accuracy and reliability of a machine learning algorithm. We used a common technique rather descriptively called “leave-one-out cross-validation.”20
There are more subtleties and complexities to this technique than we expect readers will want to know.21 But the key idea is that you can train the algorithm repeatedly, and independently, on one subset of data already collected, and ask it to make predictions about the other subset. By continuously and precisely changing the subsets, you can get a very clear sense of the algorithm’s accuracy.
For instance, if you have collected brain data on forty subjects, you can have the algorithm learn from subjects one through thirty-nine, and then make a prediction about subject forty. Then you can start over, having the algorithm learn from subjects two through forty, and then make a prediction about subject one. And so on, always leaving one subject out, systematically varying which subject that is. This method of repeated testing gives clear indications of the algorithm’s accuracy. If the algorithm does well in classifying the subject who was left out of the training set, no matter which subject that is, then that gives you greater confidence that the algorithm is tracking what it should be tracking.22
E. Primary Findings
To recap, we asked our subjects to play a game while we scanned their brains. They each made 125 decisions as to whether to carry a briefcase across the border when given varying information about the probability that the briefcase contained contraband (the Contraband Risk) and the probability that the case would be searched at the border (the Search Risk).
We then built an algorithm—a digital machine, essentially—that takes brain data as an input and returns one of two outputs: reckless or knowing (with respect to the contents of the briefcase). The output is the machine’s best guess about the mental state of the person whose brain data it takes as an input. We then used a variety of tools for measuring how well the machine worked. We measured how well it did with the very job that we ask fact finders to do whenever we ask them to determine whether a criminal defendant was reckless or knowing. However, unlike the fact finder, we used only information about a person’s brain instead of evidence admitted in court. Here are the two most important results.
1. Knowing and Reckless Brain States Differ
Our paramount finding is this: our algorithm correctly classified people as knowing or reckless 71% of the time, in some conditions. Recall that prior empirical work has demonstrated that ordinary people, asked to classify people as knowing or reckless, are only slightly more likely to get a correct answer than we would get if we were to flip a coin. (That is, just above 50%.)
Our algorithm, by contrast, outperformed ordinary people, not to mention coin-flips, by a significant margin; we outperformed chance by 21% and outperformed ordinary people by almost that same amount. And unlike ordinary people, who draw on a wide range of evidence about human behavior in making their decisions about another’s mental state, the algorithm used only information about brain activity supplied by an fMRI.
It is this result that makes this experiment worth reporting to a legally minded audience. In a sense, our algorithm was able (again, in some conditions) to read minds by looking at brains. And it did not read a trivial aspect of mind; it read an aspect of mind crucial to mens rea, and therefore to criminal punishment.
Put simply: by combining fMRI brain-imaging techniques with a machine learning algorithm, we were able to distinguish among guilty minds.
2. Order of Information Matters
Last section we twice indicated that we could make distinctions, on brain data alone, “in some conditions.” That is an important caveat, and one we wish to clarify. The caveat concerns the order in which subjects received risk-relevant information.
Recall that half our subjects were first presented with information about the size of the pool of briefcases and then shown information about the likelihood that they would be searched at the border (we called this “the Contraband-First Condition”). The other half of our subjects saw these two pieces of information in reverse order (we called this “the Search-First Condition”).
Interestingly, our algorithm was excellent at classifying the mental states of those in the Search-First Condition and abysmal at classifying the mental states of those in the Contraband-First Condition. Where, as just mentioned, the algorithm correctly classi%ed subjects 71% of the time if they first saw the information about the likelihood of being searched, the algorithm succeeded in correctly classifying only 32.1% of the time when examining information about the brains of those who first saw information about the likelihood that their briefcase contained contraband.
The difference between the two sequences in which subjects received information was also reflected in the behavior of our subjects. They were far less likely to choose to carry the briefcase across the border if they were presented first with the likelihood of being searched, and second with the likelihood that the case contained contraband, than if they saw the pieces of information in reverse order. For instance, when our subjects were faced with a 40% chance of being searched, and were presented with only one case to carry, our subjects chose to carry under 40% of the time in the ContrabandFirst condition, but over 60% of the time in the Search-First condition. This same behavioral discrepancy was found, importantly, when the probabilities of the various possible payoffs from carrying were held constant. Put another way: tell someone that they have a high chance of being searched, but almost no chance their briefcase contains contraband, and they are much less likely to choose to carry it than if you tell them that there is almost no chance the case contains contraband, but there is a high probability of being searched.
II. IMPLICATIONS OF DETECTING MENS REA IN THE BRAIN
A. Immediate Legal Implications
The primary finding of our study has several important implications. First, our team’s experiment provides a clear answer to the question: Does the distinction between knowing and reckless mens rea reflect a detectable distinction between brain states? The answer is: yes.
Based on current evidence, the distinction is not simply projected onto people who are intrinsically no different from one another. Put another way, the supposed distinction is no more in the eye of the beholder than detectable differences in the brain are in the eye of the beholder.
The alternative hypothesis, recall, is that the legal definitions of knowing and reckless do not apply differentially thanks to different psychological features of defendants. They instead reflect, on that view, independently formulated judgments about which defendants should be punished more severely. But that hypothesis is not consistent with the data we collected.
Using a combination of fMRI brain imaging and an algorithmic artificial intelligence, we were able to quite reliably predict—on the basis of brain activity alone—whether or not a subject was in a knowing or reckless mental state. This suggests that differential liability can legitimately rest, if we retain our collective decision for it to do so, on there being a distinction between knowing and reckless mental states of the kind that is reflected in distinct neural activity. Our main finding is inconsistent with any argument that these distinctions between knowing and reckless are arbitrary, invented, or merely provide cover for juries or judges to punish some defendants more than others.
Although our main finding was not true in all conditions (recall that when subjects receive Contraband Risk information before receiving Search Risk information the algorithm could not accurately distinguish the reckless from the knowing) the fact that it was true in any conditions strongly suggests (subject, of course, to future studies that may replicate and extend our findings) that there is a brain difference between those the law classifies as knowing or as reckless.
Of course, it is possible that there are some real-world circumstances under which there is no meaningful difference between the knowing and the reckless mental states. But, given that we found an intrinsic difference in some experimental conditions, a more parsimonious hypothesis is that there is a brain difference, even under those other circumstances, that fMRI cannot (so far) detect.
The Model Penal Code’s assumption that those whom it classifies differently based on their mental states actually differ psychologically has never before been directly tested. While we do not suggest that the results of a single study in any domain could ever lay a question to rest forever, our study should be seen as significantly increasing the likelihood that there are brain-based differences between people who are in knowing and reckless mental states. The main implication of our study is: whatever the relative merits of keeping or eliminating the distinction, calls for reform to eliminate the distinction are on considerably weaker ground, empirically, than they were previously.
Second, our results lend support for the idea that jurors need more help figuring out how to distinguish knowing from reckless mental states in real cases. Behavioral experiments in a separate set of published studies strongly suggest that jurors are quite poor at distinguishing between these two mental states in the way the Model Penal Code instructs they must. If our brain imaging results had found no differences between the two mental states, and people cannot reliably distinguish them anyway, then a concern for justice would recommend possible elimination of the distinction between the two. But if instead there are distinctions in the brain, and jurors have a hard time sorting defendants between the two mental states, this recommends that we find a way to do a better job at instructing jurors how to sort accurately. If we are going to keep a system that punishes people in the knowing category more than people in the reckless category, then we should ensure that jurors perform very significantly above chance (50%) when assigning defendants to one category or the other.
Third, our neuroscientific methods suggest the Model Penal Code mental state categories may not be nearly as unitary as currently supposed. That is, there may be important subcategories, and multiple subtypes, of culpable mental states. More specifically, our study suggests that the distinction between knowing and reckless mental states may be greatest when subjects perceive information about the presence or absence of an element of a crime after they learn information about the likelihood of being caught. The distinction may be less obvious, or absent, when subjects perceive risks in the reverse order.
This suggests, but does not prove, that the criminal justice system is on shakier ground, perhaps, in sorting some defendants into reckless and knowing compared to other defendants. Those who are mistaken, for instance, about the illegality of their conduct—they think what they are doing is legal and so not subject to punishment—at the time that they commit a crime are possibly worse targets for classification into the categories of knowing and reckless than those who know when they act that they are engaging in illegal activity, and so are at risk of being caught. There may be a far less meaningful distinction between knowing and reckless conduct when the actor is uncertain, or unaware, of the illegality of his conduct.
This, in turn, raises the question whether policymakers should consider keeping the knowing versus reckless bifurcation for some defined circumstances or types of crimes, and eliminating it for others. To be clear, we are not advocating this (or any other) legal reform; rather we are pointing out the possibility of such reform as a potential application of our findings.
Fourth, our team’s experiment provides a concrete example of how neuroscientific methods can open new avenues for discovering answers to some of the law’s enduring questions. On the one hand, we hasten to add that we are not zealots at the altar of a brain-scanning machine. We do not think that brain-scanning will entirely upend long-standing legal approaches to issues in either criminal law or civil law. We are pragmatists, observing the potential utility of new technology and associated methods. On the other hand, we believe this study clearly and amply demonstrates that there are some questions relevant to law as to which brain scanning can provide valuable new information. And the significance of this—entirely independent of the experiment’s value in the substantive context of mens rea—should not be underestimated.
B. Implications for Future Work
The implications of our study extend beyond the boundary between knowing and reckless mental states. Our study points the way toward future studies and avenues of research, each with distinct legal implications of their own. These avenues concern: (1) other lines between mental states, drawn by the Model Penal Code; (2) other defined elements of crimes; and (3) the interaction of mental illness and criminal mental states.
First, the line between knowing and reckless, which our experiment investigated, is only one mens rea line drawn by the Model Penal Code. Similar studies, therefore, could be done to determine whether purpose and knowledge can be distinguished based on brain data alone, or whether recklessness and negligence can be. Further, the Model Penal Code’s divisions are not the only mens rea distinctions drawn in American law. The Model Penal Code also equates awareness of high probability with knowledge under certain circumstances.23 Do we lose the ability to distinguish between those two under the special circumstances in which the Model Penal Code equates them, or not?
Second, when we move beyond the Model Penal Code’s mens rea regime we find various other questions that could be explored using the sort of tools we developed for this study. For instance, many jurisdictions in the United States reserve the most severe penalties for murders that are “willful, deliberate and premeditated.”24 Is it possible to distinguish acts performed with that frame of mind, from those that are not, solely based on brain data?
Third, our study specifically concerned knowledge and recklessness with respect to a circumstantial element of a crime—the presence or absence of contraband in the case, a fact that accompanies, but need not be caused by, the act of crossing the border. It is possible that we would not find the same, or any, brain-based difference even when it comes to other circumstantial elements of crimes.
Perhaps, for instance, the line between knowledge and recklessness when it comes to another’s consent—the absence of which can also be a circumstantial element of a crime—cannot be drawn neurally, or must be drawn differently. Further work could investigate different forms of potentially illegal behavior also involving circumstantial elements. Further work could also expand beyond circumstantial elements to result and act elements of crimes. We do not know whether our results would extend to mens rea at the time of the act with respect to future harms that the act might cause.
Fourth, with further development, our team’s work could be extended to investigate the interaction of mental illness with criminally-culpable mental states, about which we have almost no evidence-based knowledge. Except in those rare states that bar the use of evidence of mental disorder to negate mens rea,25 defendants routinely introduce evidence of the existence of certain recognized mental disorders—schizophrenia, post-traumatic stress disorder, autism spectrum disorder, and depression, for example—to raise reasonable doubt about the presence of the required mens rea element of the crime. But there are to date no studies that directly examine the impact of mental disorders on mens rea.
Fact finders receive some guidance from clinicians and forensic psychiatrists. But these experts’ judgments are not supported by systematic, experimental findings. It is not hard to see why: to investigate the question of whether PTSD sufferers, for example, are more likely than non-PTSD sufferers to know, as opposed to being reckless about, features of their environment that bear on their criminality, we would need a way of measuring which mental state they are in, under lab conditions. Our study shows that tools for making such measurements can be developed, from combining existing fMRI technology with methods of artificial intelligence.
For similar reasons, our study shows that these tools can help to measure the impact of intoxicants on mens rea. Although there are significant limitations on how voluntary intoxication can be used to negate mens rea, most states allow defendants to shield themselves from liability on the grounds that due to intoxication they failed to know something, even if they would have known it had they been sober.26 There are many different intoxicants, of course, and they vary enormously in their psychological effects. Yet there is no data-driven work, akin to the experiment we’ve just described, that investigates the differential impact of, for instance, alcohol, cocaine, methamphetamine or marijuana on the “knowing” mental state. There now could be.
III. CAUTIONS & CAVEATS
The brain imaging method we used—fMRI—is a fairly recent technological advance, and a remarkable technique for learning about brain activity in a relatively non-invasive way. For this reason, publication of MRI and fMRI studies from major universities (which can pay several million dollars for a high field-strength machine) has exploded. For instance, a literature search in the widely-used PubMed database revealed that although in 1987 fewer than 200 articles using these two methods were published each month, by 2014 that figure was typically greater than 2,000 per month27—a ten-fold increase in twenty-six years. Looking just at fMRI publications, a 2010 study in the same database found a rise in annual publications from effectively zero in 1992 to well over 2,000 annually in 2009.28
At the same time, we want readers to understand that we pitch down the middle—neither more zealous nor more skeptical about the technology than fMRI is due. Studies by our working group, by other working groups in our Research Network, and by other research teams around the world, have demonstrated that neuroscientific techniques can add value to law’s efforts. But brain-scanning is not magic. It has limitations, many of which we have helped to explore and detail.29 For this reason, we believe it is appropriate to lay on the table a variety of cautions that might help readers to strike the right balance between under- and over-interpreting the specific findings we describe here, as well as fMRI studies in general.30
A. General Cautions & Caveats
First, there is always a trade-off between how closely experimental conditions align with the real world and how many potential variables, any one of which might influence a subject’s behavior, can be controlled. Increased realism decreases confidence in conclusions about what actually caused what. Yet increased control over variables decreases confidence in the generalizability of a study’s findings, which might only hold true in identically controlled circumstances. Although we have no reason at present to think that brain activity differences between knowing and reckless frames of mind are only different inside the scanner, transparency requires that we at least mention the possibility.
Second, although our sample size of forty subjects is within the norm in fMRI brain imaging studies, for investigating brain activity with sufficient statistical power to publish findings in top peer-reviewed neuroscience journals, there is always the possibility that a larger study would find either more or fewer differences between the knowing and reckless mental states.
Third, sampling different demographic groups might yield different results. Our subjects are from the Roanoke/Blacksburg, Virginia area. Although there is at present no reason to believe that different groups will use their brains quite differently with respect to mens rea, we would be remiss not to mention the possibility that variables such as age, profession, education, sex, nationality, nutrition, health, or socioeconomic status could affect the results.
Fourth, fMRI is an indirect, rather than direct, measure of neuronal activity. Instead of measuring the electrical activity of individual neurons, or even a group of them, fMRI detects changes in blood oxygenation levels, over time, in discrete locations within a subject’s brain that include neurons, as well as other brain tissue. There is every physiological reason to believe that the more various neurons fire, the more resources (such as oxygen and glucose) they demand. Still, it is a little like distinguishing cities from countryside by measuring differential regional light outputs from space at night. In the same way that that would measure something very reliably associated with cities, but would not be a direct observation of cities themselves, fMRI measures something very reliably associated with neuronal activity, without measuring the neuronal firings themselves.
Fifth, fMRI cannot identify differences between the kinds of neurons that are active. fMRI compares total activity within voxels (which are, as mentioned earlier, cubic volumes of brain tissue). But each voxel contains a great many neurons in number—usually estimated as over 600,000—and can also contain many different types of neurons. Some neurons, for instance, fire in a way that activates other neurons. But some neurons fire in a way that inhibits the activation of other neurons. Because fMRI does not distinguish among these sometimes competing purposes of neurons, it is akin to recording the decibel level in a crowd of people, many of whom are yelling “go,” some of whom are yelling “no,” and some of whom are keeping quiet.
Sixth, the fMRI brain images that researchers present and publish are not like x-ray images, which are the direct result of imaging technology interacting with brain tissue. fMRI images are, instead, statistical parametric maps. Which means they are structural images (akin to an x-ray image) of a single, typical brain onto which has been overlaid a patchwork variety of colors, in various locations, that represent the voxels with the most statistically significant differences between conditions. (Such as, in our case, the knowing and reckless conditions.) The colors are calibrated to the range of greater and lesser differences.
Seventh, as tempting as it can be to laud the breakthrough capabilities of partnering machine-learning algorithms with brain-scanners, it is also important for legal thinkers to see their limitations with a clear eye, and to not succumb to temptation to overinterpret results. Specifically, we believe that although multivoxel pattern classification is a powerful tool for identifying the existence of salient brain differences, it will rarely provide strong support for claims about either (1) the precise function of any brain region; or (2) any brain region’s centrality to any particular and complex form of psychological functioning. Although algorithms are capable of learning to apply complex, disjunctive rules for classification, rules of that kind are not automatically useful for gaining insight into the basic psycho-physical laws that govern the relationship between brain activity and psychological states.
B. Specific Cautions & Caveats
There are a variety of things that our experiment could be taken to imply that it does not imply. And these are important to highlight.
First, and most importantly, scientific findings never provide automatic support for a change in policy (or, conversely, a continuation of existing policy). So our findings don’t either. Sound policymaking or policy-reform always requires that policy-makers view facts through the prism of values and consider them in light of fundamental normative principles. Put another way, there is no automatic pathway from description to prescription, or from explanation to justification. Facts warrant attention, of course. But whether they should inspire change depends on what it is that society is trying to accomplish, and what principles it must comply with in the e)ort. In context, that means that if a state’s statutory regime establishing different criminally culpable mental states is structured by and grounded on the assumption that there really are brain-based differences in those mental states, then facts supporting that assumption tend to increase our confidence in the regime. And facts inconsistent with that assumption tend to weaken it.
Neither the results of our experiment, nor the results of any experiment, can alone answer the question whether we should or should not keep four categories of mental state, much less the four particular categories defined in the Model Penal Code. The fact that our experiment has found a brain-based distinction between knowing and reckless mental states cannot automatically justify the continued division of those states in the law, any more than would the absence of such a finding demand the elimination of the distinction. To be clear, the implication of our finding is not that the law must retain the knowing-reckless distinction; it is, instead, that to the extent that the best policies require that the mens rea categories re#ect differences in brain states, our finding provides some support for maintaining the distinction. But whether the best policies require that is a profoundly difficult question that cannot be answered by doing experiments.
Second, our team’s neuroscientific techniques can discover brain-based differences between mental states that exist at the time of scanning, not at some prior time. Although we have developed and deployed a powerful tool for exploring whether such differences exist, it is not (at least not so far) a tool for reliably exploring what mental state a subject was in minutes, hours, days, or even years beforehand. Put another way, our current experiment has implications for criminal justice policy, but not for forensic evaluation of individual defendants.
Third, the extent to which our study read the minds of subjects should not be exaggerated. True, it is remarkable, frankly, that the algorithm could classify subjects as knowing or reckless taking only information about their brains, collected non-invasively, into account. Such a thing would have been inconceivable twenty-five years ago. But that does not imply we now have a general-purpose mind-reading capability. Instead, our experiment showed that there were su+ciently great differences between knowing and reckless brain activity that the combination of fMRI and artificial intelligence could learn that difference (not just—on its own—discover and name the difference). The crucial distinction, and the point we are emphasizing here, is that human instructors had to provide the algorithm with two potentially different conditions to examine, in the first place. Had we not asked the algorithm to look for differences in subjects between these two conditions, it would not, on its own, have looked for (or thereby found) any.
Fourth, it is worth noting that we have not yet said in this article what kinds of activities, in which particular brain structures, enabled our algorithm to distinguish between subjects in knowing and reckless states of mind. The reason we have said nothing about this so far is not that our study has nothing to say. It does.31 The region known as the dorsomedial prefrontal cortex, for instance (a region known to be involved in planning, analysis, and deliberation) was among the regions of the brain that behaved distinctively in subjects in states of knowledge. For purposes of our specific research question—whether knowing and reckless mental states are distinguishable in the brain—the locations of differences is simply less legally relevant than the fact that discernable differences exist. That is, the central result that we reached—distinguishing knowledge from recklessness solely on the basis of brain data—is significant quite independently of what aspects of the brain made the result possible.
Fifth, the stimuli we used in the lab to elicit the mental states to be studied may or may not elicit those mental states perfectly. We strongly believe that the essence of the distinction between knowing and reckless mental states, as envisaged under the Model Penal Code, reflects different probabilities—such that (1) judging there to be a 100% likelihood that something will happen is “knowing” that it will; and (2) lesser likelihoods re,ect lessening degrees of “recklessness.” Nevertheless, one could always argue that manipulating certainty and uncertainty in the laboratory misses something essential about the Model Penal Code mental states.
Sixth, context may matter. It is possible that the particular brain-based distinctions our team has identified between knowing and reckless decisions, when deciding whether or not to carry contraband, may not generalize to all knowing and reckless decisions, when deciding whether or not to engage in other kinds of activities.
Seventh, it seems appropriate to acknowledge that as researchers we can’t be certain what a subject actually “knew” in the scanner. We told subjects that one (and only one) case each trial would contain “valuable content.” And we also told them that each trial they would be presented with between one and five cases. Therefore, subjects shown only one case on a given trial could straightforwardly deduce that that case logically must contain the valuable content, because the probability that it would do so is 100%. When only one case was on offer, subjects indeed behaved as if they knew. But we cannot claim that all subjects actually knew what they clearly should have known.
CONCLUSION
Pick any weekday, and you will find hundreds of felony trials underway in America. With rare exceptions, the question is whether the accused did something prohibited while in a culpable state of mind. In the supermajority of states that follow the Model Penal Code, there are four culpable states of mind: purposeful, knowing, reckless, and negligent.
The law predicates differences in criminal liability on what the law supposes to be independently specifiable psychological differences that underlie and constitute differences in criminal culpability. But is this presupposition true? If there are such psychological differences, there must also be brain differences. Consequently, the moral legitimacy of the Model Penal Code’s taxonomy of culpable mental states—which punishes those in defined mental states di)erently—depends on whether those mental states actually correspond to different brain states in the way the Model Penal Code categorization assumes.
The experiment described here is the first to investigate whether one longstanding assumption underlying the Model Penal Code’s approach to culpable mental states stands up to empirical scrutiny. More specifically, we and our colleagues coupled fMRI brain imaging techniques and a machine learning algorithm (a form of artificial intelligence) to see if the brain activities during knowing and reckless states of mind can ever be reliably distinguished.
The answer is Yes. So our experiment provides a concrete example of how neuroscientific methods can contribute information relevant to legal policy. First and foremost, however, our experiment demonstrates that it is possible to predict, with high accuracy, which mental state a subject is in using brain imaging data alone. The results therefore provide the first empirical support for the law to draw a line between, and to establish separate punishment amounts for, knowing and reckless criminality. This discovery could be the first step toward legally defined mental states that reflect actual and detectable psychological states, grounded in neural activity within the brain.
Our results do not by themselves suggest that there should or shouldn’t be reform of the law of mens rea. We have provided evidence that knowing and reckless mental states are—at least in some contexts—different in the brain. We believe that information is valuable if one cares about whether or not the Model Penal Code’s approach to culpable mental states can bear the weight that the law asks it to. But our finding that the mental states can indeed re,ect di)erent brain activity does not mean the Model Penal Code distinction between knowing and reckless states should remain intact, any more than a contrary finding would mean that the Model Penal Code distinction between knowing and reckless mental states should be abandoned. Support for policy change comes from the intersection of values, facts, and fundamental principles, and not from the facts alone.
We should all be interested in evidence-based legal reforms. But such reforms require evidence on which they can be based. When it comes to the law of mens rea, the relevant source of such evidence is psychology, cognitive science and, thanks to increasingly sophisticated technology for measuring the brain, neuroscience. As we hope to have demonstrated here, when neuroscientific techniques are aimed directly at questions of legal relevance, they can provide exactly the kind of evidence that can aid, although not dictate, intelligent, thoughtful legal reform.