Abstract
The likelihood of initiating addictive behaviors is higher during adolescence than during any other developmental period. The differential developmental trajectories of brain regions involved in motivation and control processes may lead to adolescents’ increased risk taking in general, which may be exacerbated by the neural consequences of drug use. Neuroimaging studies suggest that increased risk-taking behavior in adolescence is related to an imbalance between prefrontal cortical regions, associated with executive functions, and subcortical brain regions related to affect and motivation. Dual-process models of addictive behaviors are similarly concerned with difficulties in controlling abnormally strong motivational processes. We acknowledge concerns raised about dual-process models, but argue that they can be addressed by carefully considering levels of description: motivational processes and top-down biasing can be understood as intertwined, co-developing components of more versus less reflective states of processing. We illustrate this with a model that further emphasizes temporal dynamics. Finally, behavioral interventions for addiction are discussed. Insights in the development of control and motivation may help to better understand – and more efficiently intervene in – vulnerabilities involving control and motivation.
1. Introduction
Motivation, from a subjective point of view, appears to be a relatively simple process – I want something, and therefore I attempt to get it. It is when motivational processes “go wrong,” or when we ask how this apparently seamless relationship between wanting and doing arises, that the development of motivation and its relationship to behavior becomes a theoretically interesting question with significant practical importance. Adolescence and addiction exemplify situations in which the development of motivational processes can result in excessively risky or otherwise dysfunctional behavior. In this review, we will focus on symmetries between adolescence- and addiction-related changes in motivation and cognitive control that may help explain increased the likelihood of substance abuse and the onset of addiction in adolescence.
Adolescence is the developmental period between childhood and adulthood, shared by humans and other mammal species (Dahl, 2004, Spear, 2000). In humans, far-reaching changes in body appearance and function, including hormonal changes, are paralleled by important changes in behavior and psychological processes such as motivation, cognitive control, emotion, and social orientation (for overviews, see, e.g., Reyna and Farley, 2006, Spear and Varlinskaya, 2010, Steinberg, 2005). During adolescence, children increasingly master the ability to control their behavior for the benefit of longer-term goals (Best et al., 2009, Cragg and Nation, 2008, Crone et al., 2006a, Huizinga et al., 2006). These advances in self-regulation abilities in adolescence, however, are accompanied by pronounced changes in motivational processes. These can have profound consequences for health in adolescence, as the imbalance between relatively strong affective processes and still immature regulatory skills might contribute to the onset of developmental disorders (Ernst et al., 2006).
2. Risky decision making in adolescence
Adolescents are known to have a tendency to take more and greater risks than individuals in other age groups in many life domains, such as unprotected sex, criminal behavior, dangerous behavior in traffic, and experimenting with and using alcohol, tobacco, and legal or illegal drugs (Arnett, 1992). Typically, the occurrence of these risk-taking behaviors follows an inverted U-shape pattern across development, being relatively low in childhood, increasing and peaking in adolescence and young adulthood, and declining again thereafter (for overviews, see, e.g., Reyna and Farley, 2006, Steinberg, 2004). This phenomenon of adolescent risk-taking(i.e., an inverted U-shaped trajectory with a peak in adolescence and young adulthood) has received much attention, but only recently has research started to identify the triggers and circumstances that contribute to increased risk taking in adolescents under controlled laboratory conditions.
Despite the well-documented adolescent peak in risk taking from reports about everyday behaviors (such as accident and crime statistics), this pattern has only recently been demonstrated in experimental studies, which dissociated between “hot” affect-charged versus “cold” predominantly deliberative processes in risky choice and risk taking. Studies using the hot and cold versions of the Columbia Card Task (CCT; Figner et al., 2009a, Figner et al., 2009b, Figner and Voelki, 2004, Figner and Weber, in press; for a brief description, see Fig. 1) have shown that heightened involvement of affective processes in risky decision-making (in the hot CCT) leads to increased risk taking in adolescents (associated with impoverished use of risk-relevant information), compared to children and adults (Figner et al., 2009a, Figner et al., 2009b; see also Burnett et al., 2010). In contrast, when risky decisions are made involving mainly deliberative processes and no or little affect (cold CCT), adolescents show the same levels of risk taking as children and adults (Figner et al., 2009a, Figner et al., 2009b; see also van Duijvenvoorde et al., 2010, for similar hot/cold differences in adolescents). These findings show similarities to findings from research using a simulated driving game in which the presence of peers leads to increased risk taking in adolescents (but not adults) when making choices between driving through versus stopping at traffic intersections with a yellow light (Chein et al., 2011, Gardner and Steinberg, 2005; see also Hensley, 1977). This socio-emotional influence of peers on adolescents is not surprising given that much risk taking in adolescents occurs in the presence of peers (Gardner and Steinberg, 2005), and more broadly, given the changes in social motivations during this period, with adolescents achieving greater independence from their parents and, in turn, peers growing in importance (Blakemore, 2008, Brown, 2004)
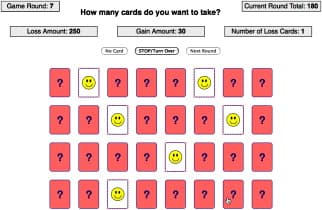
Fig. 1. Example display of a game round of the hot version of the Columbia Card Task (CCT; for more information, see http://www.columbiacardtask.org ). In the CCT, participants play multiple game rounds of a risky choice task. In each new game round, participants start with a score of 0 points and all 32 cards shown from the back. Participants turn over one card after the other, receiving feedback after each card whether the turned card was a gain card or a loss card. A game round continues (and points accumulate) until the player decides to stop or until he or she turns over a loss card. Turning over a loss card leads to a large loss of points and ends the current game round. The main variable of interest is how many cards participants turn over before they decide to stop. The number of cards chosen indicates risk taking because each decision to turn over an additional card increases the outcome variability, as the probability of a negative outcome (turning over a loss card) increases and the probability of a positive outcome (turning over a gain card) decreases. Across the multiple game rounds rounds, three variables systematically vary, (i) the gain magnitude (here 30 points per good card), (ii) the loss magnitude (here 250 points), and (iii) the probability to incur a gain or a loss (here 1 loss card). This factorial design is an advantage the CCT has over other, similar dynamic risky choice tasks, as it allows to for the assessment of how these three important factors influence an individual's risky choices and risk-taking levels, e.g., in the form of individual differences in gain sensitivity, loss sensitivity, and probability sensitivity. In the cold CCT, to reduce the involvement of affective processes (Figner et al., 2009a, Figner and Murphy, 2011), feedback is delayed until all game rounds are finished; in addition, instead of making multiple binary “take another card/stop” decisions as in the hot CCT, participants in the cold CCT make one single decision per game round, indicating how many cards they want to turn over.
However, it should be noted that the inverted U-shape observed in many everyday risk-taking behaviors remains somewhat elusive to assess with laboratory risky choice tasks. Besides the mentioned results with the hot CCT (Figner et al., 2009a, Figner et al., 2009b), only few studies found the pattern commonly observed in the “real world.” Burnett et al. (2010) found an inverted U-shape in risk taking with a peak in adolescents aged 12–15 years with a probabilistic task designed to evoke regret and relief (thus likely making it a relatively “hot” task), again pointing to the importance of affective processes in adolescent risk taking. Cauffman et al. (2010) also found an inverted U-shape in a risky choice task (the Iowa Gambling Task), but the measured behavior captured approach motivation (or sensitivity to expected value) rather than risk taking itself. Finally, Steinberg et al. (2008) found an inverted U-shape pattern with a self-report measure, but their behavioral measure (a task similar to the one used in Gardner and Steinberg, 2005) showed a developmental pattern resembling a non-inverted U-shape with adolescents apparently taking less risks than children and adults. Finally, several other laboratory studies have found evidence for monotonically decreasing risk-taking levels across childhood, adolescence, and adults, which may indicate that these tasks captured more the cognitive or cold processes involved in evaluating risks (e.g., Crone et al., 2008, Weller et al., 2010). Clearly, more research is needed to further investigate precisely which “ingredients” of a risky choice situation (besides substantial affective involvement and peer-presence) lead to increased risk taking in adolescence.
3. Neural developments underlying risky decision making
Insights from anatomical and functional changes in the rodent and the human brain have led to neurobiological models characterizing the “adolescent brain” by two interacting systems. The first is the relatively early maturing and “hot” affective-motivational bottom-up system and the second is the more slowly developing “cold” top-down control system (for overview papers, see, e.g., Casey et al., 2008, Casey and Jones, 2010, Ernst et al., 2006, Ernst et al., 2009, Galvan, 2010, Somerville et al., 2010a, Somerville et al., 2010b, Steinberg, 2008). The affective-motivational system involves subcortical brain areas, including the dopamine-rich areas in the midbrain and their subcortical and cortical targets, including the striatum and the medial prefrontal cortex (both of which have been implicated in the representation of rewards, e.g., Knutson et al., 2009, Tom et al., 2007). The cognitive top-down control system is thought to involve prefrontal regions, particularly the lateral prefrontal cortex, and posterior parietal brain regions, which have been implicated in self-control, planning, abstract thinking, working memory, and goal-directed behavior, for example enabling the individual to resist short-term temptations in exchange for longer-term benefits (Adleman et al., 2002, Crone et al., 2006b, Figner et al., 2010, Klingberg et al., 2002, Robbins, 2007). Indeed, the lateral prefrontal cortex has previously been causally implicated in self-control in risky (e.g., Knoch et al., 2006) and intertemporal choice (Figner et al., 2010). That is, transient disruption of the function of these brain region using transcranial magnetic stimulation has led to increased risk taking (Knoch et al., 2006) and increased impatience and impulsivity (Figner et al., 2010) in adult participants, providing causal support for the involvement of the lateral prefrontal cortex in control.
The hypothesis that these brain systems develop at a different pace during childhood and adolescence and into young adulthood has received support from anatomical brain development studies. Animal and human studies have shown converging evidence of neural changes in several brain areas during the transition from childhood to adulthood. These include changes in dopamine-receptor densities, changes in white matter (such as increasing myelinization and anatomical connectivity through white matter tracts), and changes in gray matter (for overviews, see, e.g., Casey and Jones, 2010, Fareri et al., 2008, Galvan, 2010, Somerville et al., 2010a, Somerville et al., 2010b, Spear and Varlinskaya, 2010). Human functional neuroimaging studies have supported the notion that subcortical brain regions and networks implicated in “bottom-up” affective-motivational processes—such as processing of rewards and approach motivation—mature around the onset of puberty and early adolescence (e.g., Galvan et al., 2006, Geier et al., 2010, Van Leijenhorst et al., 2010; however, see also Bjork et al., 2004, Bjork et al., 2010; for an overview and discussion, see Galvan, 2010). In contrast, cortical brain regions important for “top-down” control (Miller and Cohen, 2001) mature later in development, and more gradually into young adulthood (Crone and Ridderinkhof, 2011, Giedd, 2008). There is evidence for hyperactivity in the striatum during adolescence when anticipating and representing rewards (e.g., Ernst et al., 2005, Galvan et al., 2006, Van Leijenhorst et al., 2010; but see also Bjork et al., 2004, Bjork et al., 2010, for evidence of striatal hypoactivity; for overviews and discussion see Fareri et al., 2008, Galvan, 2010) and that it might be particularly difficult for adolescents (compared to children and adults) to inhibit approach behavior in the presence of appetitive salient cues (Somerville et al., 2010a). Similarly, a recent model-based fMRI study showed increased neural signals for positive prediction errors in the striatum in adolescents (compared to children and adults), again possibly contributing to increased risk-taking behaviors in this age group (Cohen et al., 2010). We note at this point that despite the above patterns of results, care must be taken not to assign cognitive functions to the striatum and prefrontal cortex too directly; for instance, both regions are involved in working memory processes (Bunge and Wright, 2007, Hazy et al., 2006). That is to say, the full network of neural mechanisms underlying functions defined at a cognitive or behavioral level may be more extensive than the regions that are differentially activated over conditions, so that while specific regions may be shown to play a (more prominent, consistent or extended) role in a certain function, care must be taken not to conclude that they can be considered to encapsulate that function.
The above frontostriatal model of adolescent decision-making thus describes a potential for imbalance in motivational bottom-up versus controlling top-down processes. For example, an adolescent encountering a salient reward during risky choice may experience strong temptation and approach motivation, which may result in a prepotent response to take a risk in order to achieve the potential reward. An adult in this situation may be better able than the adolescent to inhibit this prepotent response, resist the immediate temptation, perhaps wait before acting and think twice about it whether it is worth taking this risk. As research has demonstrated, this dynamic of strong motivational processes versus relatively weak prefrontal control can be particularly disadvantageous with respect to high and dangerous levels of risk taking when affective-motivational processes are strongly triggered, for example in affect-charged situations (Figner et al., 2009a, Figner et al., 2009b) or in the presence of peers (Chein et al., 2011, Gardner and Steinberg, 2005). Obviously, there are individual differences in the extent to which adolescents and adults are prone to take risks (Figner and Weber, in press, Galvan et al., 2006). Nonetheless, in adolescence individuals are more likely to take risks than in adulthood, and therefore especially in this phase of life, it is important to understand the triggers that may cause some adolescents to take unhealthy risks, such as in the case of addiction.
4. Addiction
Drug use is an example of risky behavior that is of particular concern in adolescence. Current neurobiological research has identified two broad types of neuro-adaptations that result from repeated alcohol and drug use. Both seem likely to interact with normal developmental risk factors of adolescence; indeed, most addictive behaviors start in adolescence, when there is a high incidence of experimenting with psychoactive substances such as alcohol, tobacco, and legal and illegal drugs. Most teenagers are incited to drink their first alcoholic beverage in (early) adolescence: 43% of European adolescents have tried alcohol before the age of 13 (Hibell et al., 2009). There is a robust association between age of onset and risk for subsequent alcohol and drug problems (Agrawal et al., 2009, Grant et al., 2006), and between heavy adolescent drinking and later alcohol problems (White et al., 2011).
First, neural sensitization leads to strong impulsive reactions (e.g., attentional biases and approach tendencies) to classically conditioned cues that signal alcohol or drugs (Robinson and Berridge, 2003), and there is evidence from animal research that this occurs more rapidly during adolescence (Brenhouse and Andersen, 2008, Brenhouse et al., 2008). In later phases of addiction, habitual responses become important, with cues automatically triggering approach-reactions, outside voluntary control (Everitt and Robbins, 2005). In addition, there is evidence that new initiation of drug use may be triggered by negative affect, which is then temporarily relieved (Koob and Le Moal, 2008a, Koob and Le Moal, 2008b). Such negative reinforcement processes are associated with severe drug dependence (Koob and Le Moal, 2008b, Koob and Volkow, 2010, Saraceno et al., 2009). Note that all of these neuro-adaptations have the effect of gradually decreasing voluntary control over substance use. Increased incentive salience may result in attentional capture (“attentional bias”) of substance-related cues (Robinson and Berridge, 2003); a habitual response that becomes increasingly hard to control (Everitt and Robbins, 2005). Finally, negative affect may trigger an urge to take drugs through associative processes (Baker et al., 2004).
Second, there is evidence that heavy alcohol and drug use results in impaired control functions, especially when heavy use takes place during adolescence (Clark et al., 2008). There are two mutually supportive lines of evidence for this thesis. First, there is relatively strong experimental evidence from animal research (Crews and Boettiger, 2009, Crews et al., 2006, Crews et al., 2007, Nasrallah et al., 2009), supporting the idea that binge drinking has strong effects on subsequent brain development involving cognitive and emotional regulatory processes. For example, Nasrallah and colleagues found that adolescent rats who had voluntarily consumed high levels of alcohol during adolescence, demonstrated greater risk preference in adulthood than control rats who had not consumed alcohol during adolescence. The difficulty with this line of evidence lies in the generalization to humans, where cognitive control over emotional processes may develop over a longer period and to a greater extent and where participants cannot be randomly assigned to conditions. The second line of evidence comes from human studies employing various neuroimaging techniques in cross-sectional samples. These studies have demonstrated that adolescent binge-drinking and heavy use of marijuana is associated with abnormalities, both regarding white matter structure (Bava et al., 2009, Jacobus et al., 2009, McQueeny et al., 2009), and regarding functional properties (Clark et al., 2008), including stronger cue-reactivity (Tapert et al., 2003) as well as impaired executive functions (Tapert et al., 2004, Tapert et al., 2007). However, these studies are cross-sectional, and can therefore not determine a causal effect of these substances on subsequent brain development (although dose-response relationships and the converging animal literature are suggestive of such a link).
Longitudinal human data using neuropsychological tests and parallel animal work confirm the assumption that binge drinking (especially multiple successive detoxifications) can result in brain damage in a variety of regions, including those involved in cognitive control (Loeber et al., 2009, Squeglia et al., 2009, Stephens and Duka, 2008). There are few longitudinal behavioral studies concerning the interplay between (adolescent) binge drinking and the development of cognitive control. One of the few existing studies (White et al., 2011) found that impulsive behaviors prospectively predicted binge drinking and also that binge drinking predicted increases in impulsive behaviors. Interestingly, it was also found that these effects may be remediated in some cases, when heavy drinking stops: adolescents who were heavy drinkers at age 14, but not anymore at age 15 and 16 moved from the increased impulsivity trajectory back to the normative development trajectory. Similar bidirectional relationships between binge-drinking and impulsive decision making have also been reported in young adults (Goudriaan et al., 2007, Goudriaan et al., 2011).
In summary, while most adolescents experiment with at least some addictive substances, only few of them become addicted. This highlights the importance of better understanding individual differences, especially in development, that form risk or protective factors for addiction. Further, there is mounting evidence that adolescent substance use may exaggerate the normal inverted U curve of risky behavior in adolescence, and may in some cases (related to heavy substance use and/or pre-morbid levels of impulsivity) postpone or even prevent the decrease in risky behaviors normally starting in early adulthood.
5. Dual-process models of addiction
The literature on adolescent risk-taking and addiction converges on the idea of dual processes, i.e., the existence of two qualitatively different types of process that underlie and may compete for control of behavior, prominent in many fields of psychological theory (Evans, 2008, Posner, 1980, Shiffrin and Schneider, 1977, Strack and Deutsch, 2004). These processes have been described using a variety of terms (Evans, 2008), e.g., impulsive versus reflective (Bechara, 2005, Hofmann et al., 2008, Strack and Deutsch, 2004, Wiers et al., 2007) or automatic versus controlled (Shiffrin and Schneider, 1977). These labels characterize the processes underlying observed behavior in terms of, for instance, less versus more aware, intentional, efficient and controllable (Bargh, 1994, Moors and De Houwer, 2006) or unconscious versus conscious, implicit versus explicit, low versus high effort, parallel versus sequential (Evans, 2008). In both adolescence and addiction, the literature suggests that risky behavior results from an inability of reflective processes to sufficiently modulate the effects of impulsive processes, for instance by modulating the salience of information in working memory (Finn, 2002). This imbalance is made explicit in dual-process models of addiction, which emphasize the importance of drug-related consequences on the relationship between impulsive and reflective processes (Bechara, 2005, Deutsch et al., 2006, Stacy et al., 2004, Wiers et al., 2007). Such models are supported by findings showing that higher working memory capacity (Grenard et al., 2008, Thush et al., 2008) and interference control capacity (Houben and Wiers, 2009, Wiers et al., 2009a) weaken the impact of alcohol-related automatic processes.
However, limitations of dual-processing models must be noted. First, it has long been acknowledged that the sets of binary characteristics that define impulsive/automatic versus reflective/controlled processing do not perfectly covary; e.g., a process may be efficient (a property of automatic processes) but still depend on intentions (a property of controlled processes) (Bargh, 1994, Evans, 2008, Moors and De Houwer, 2006). This suggests that a simple binary division of processes underlying behavior is untenable (see also Conrey et al., 2005). Second, models or metaphors in which dual processes are defined in terms of dual systems (rather than sets of essential properties) have been criticized as lacking strong evidence (Keren and Schul, 2009). For instance, the finding of brain activation differentially related to one or the other type of processing cannot logically be taken as evidence for separable processing systems. Third, it has been argued that there is a rather loose use of various theoretical terms such as working memory, executive control, cognitive control, control, and so forth (Keren and Schul, 2009) (the current paper so far not being an exception). Finally, the essential role of motivation in dual-process theories, that is, why controlled processing does what and when, is often unexplained. This risks implicitly introducing a motivational homunculus: that is, when controlled processing is required to “do the right thing” given a certain task, context, or set of long-term contingencies, it must be explained why the control exerted by the subject should be task-appropriate or have a long-term positive expected outcome. Evidently, motivation and control must be interwoven (Hazy et al., 2006, Kouneiher et al., 2009, Pessoa, 2009), as opposed to functioning as competing processes. Indeed, there has been increasing interest in the integration of motivation and reinforcement on the one hand and controlled processing on the other (Hazy et al., 2006, Kouneiher et al., 2009, Pessoa, 2009, Robbins, 2007).
Do such criticisms imply a fatal flaw in dual-process models? Not necessarily. First, a distinction can be made between specific top-down biasing processes, strongly associated with prefrontal cortex (Cohen et al., 1996, Egner and Hirsch, 2005, Hopfinger et al., 2000, Kouneiher et al., 2009), versus the stateof reflective processing, which must exist at a higher level of aggregation to include motivational aspects of control. Reflective processing can then be defined as the selection of top-down biasing functions based on an evaluation of their expected outcome (see Fig. 2). This levels-of-description redefinition of reflective processing as a state, versus top-down biasing as a process, can be seen as a generalization of, e.g., the iterative reprocessing model (Cunningham and Zelazo, 2007, Cunningham et al., 2007) or decision field theory (Busemeyer and Townsend, 1993). Automaticity, in contrast, would refer to the degree to which the evaluated selection of top-down biasing fails to play a role in response selection or decision-making.
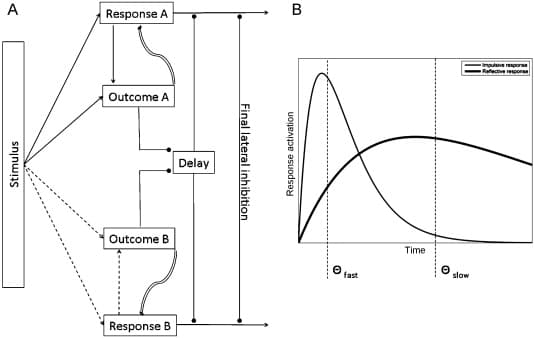
Fig. 2. A sketch of an implementation of the Reinforcement/Reprocessing model of Reflectivity (R3 model). Subfigure (A) Stimuli are proposed to activate associated responses, including generalized cognitive responses such as top-down biasing, which in turn activate expected outcomes via stimulus–response–outcome associations. Stronger and weaker associations are shown as continuous and dashed arrows, respectively. Associative processes determine the speed with which this activation builds up: responses will have varying association strengths with stimuli or outcomes. Outcomes are assumed to provide positive or negative feedback to responses during reprocessing. However, since a given outcome is not assumed to be uniquely coupled to a specific response, the association is considered to be more transient and flexible than the stimulus–response–outcome connections, as represented by the different line style (curved and shaded). For example, some form of temporal coding could be hypothesized, in which different response–outcome pairs are distinguished based on the timing of activation relative to the phase of a persistent background oscillation. A slowly activated response may eventually be found to provide a better ultimate outcome (i.e., match to cue-evoked goals) than a more immediately available response with a strongly associated payoff. The time allowed for the search for responses and outcomes is determined by the set of processes that defer response execution, represented here by the stop sign and its inhibitory effect on response execution (circle-headed arrows). Note that such a delay allows responses and outcomes that are yet to be activated, and hence will only be able to compete or cause conflict after some delay, to have a chance of influencing behavior. Responses may involve the delay itself: if an outcome has high value (such as the expected removal of a noxious stimulus), or if speed is important, the time to search further may be reduced. Note that reflective processing (or, here synonymously, controlled processing) exists here as a pattern of interactions between elements at lower levels of description, rather than as any single element of the model separable from reinforced associations or affective-motivational processing, or identifiable with neural systems involved with top-down biasing. Subfigure (B) shows an illustration of the activation of two responses, one of which would be selected given an early temporal threshold, while the other would require a late temporal threshold. The figure shows various points at which cognitive bias modification (Section 6) could be beneficial: training could aim to increase the strength of the association of (biasing) responses to risky stimuli, to change the (availability of) expected outcomes of responses, or to train individuals to increase the temporal threshold under risky circumstances. The model suggests that especially combining such changes could result in significant changes in the system's behavior.
Evidently, some form of prior learning must underlie the evaluation and selection of top-down biasing in reflective processing; we therefore label this perspective on dual-processing the Reinforcement/Reprocessing model of Reflectivity (R3 model), to emphasize that even highly reflective cognition is embedded in previously developed associations. As with top-down biasing, conditioning processes and learned associations exist at a different level of description than either reflective or automatic processing; that is, such processes do not need to be identified as either reflective or automatic, but could play a role in either. Similarly, specific components of working memory (e.g., the visuospatial sketch pad) do not appear to necessarily be linked exclusively to either reflective or automatic processing (except the central executive, which appears to conceptually overlap with reflective processing).
The primary aim of the model sketched above is to address the legitimate criticisms of dual-process models introduced previously. However, while the formulation of a detailed new cognitive neuroscientific model of dual processes is beyond the scope of this paper, it does appear that reflective processing needs to be explicitly integrated with affective-motivational processes in future models, including those focusing on addiction. The viewpoint developed here predicts that the reinforcement of top-down biasing functions and time factors are essential to reflective processing. The time required to activate previously reinforced biasing functions and to evaluate their outcomes may be an essential individual difference determining whether drug-related automatic processes can be controlled (Lewis, 2011). The time allowed for searching for better outcomes, and the internal criteria for settling on a currently preferred (cognitive) action may play similar roles. To illustrate, if in a highly simplified toy computational model (based on the schematic model in Fig. 2 for instance) 300 ms were set as the “reprocessing time” allotted to the search for an optimal response, then a weakly activated top-down biasing function might not be evaluated before a sub-optimal response is selected and executed. In particular during adolescence, if it is the case that prefrontal top-down biasing functions are yet to fully mature, then it appears almost unavoidable that there is a lag between the development of motivational processes and sufficient reinforcement of top-down biasing functions to be applied to them in novel situations. This may explain the difference between adolescent controlled processing in “hot” and “cold” situations. In “hot” situations, the top-down biasing functions may in fact be present, but not recruited because they are not strongly enough associated with the situation to compete with more immediate responses. Alternatively, the criterion for settling on a response is influenced by emotional or motivational salience in a particular task context: perhaps adolescents have yet to learn that stressful, emotional situations that may naturally evoke a tendency for fast responses may sometimes benefit from a more reflective state. Similarly, the relationships between stress and addictive behavior and drug-related biases may reflect a narrowing of the time window relative to the reprocessing time required to select a slowly activated response, or one in which the superior value of the expected outcome is slower to be retrieved. Incentive salience of alcohol cues, for example, could hypothetically be decomposed into a previously reinforced biasing response and a stimulus-goal association via the signaled availability of the substance. To avoid subsequent behavioral consequences, an alternative response would need to be effectively available, i.e., existing as a neural representation, associated with the stimulus, associated with alternative positive outcomes and selectable within the reprocessing time associated with the context.
Thus, if care is taken to disentangle theoretical constructs that may exist at different levels of description then dual-processing may still be considered a valid theoretical foundation for work on addiction and adolescence. Testable predictions must be derived from new models, specifying in particular relationships between control and motivation. Tests could draw on the behavioral methods and neuroimaging techniques discussed in previous sections. Further, electroencephalography (EEG) and magnetoencephalography(MEG) may form a potentially important method for testing hypotheses concerning the dynamics of reflective processing. Due to their high temporal resolution and their specific relationships to neural activity such methods can complement other neuroimaging modalities, allowing tests of the sub-second timing of events involved in reflective processing. Further, the very fine-grained temporal coding of neural activity (Roelfsema et al., 1997, Singer et al., 1996, Tallon-Baudry et al., 1999) could be of fundamental importance to brain function. In particular, EEG and MEG studies have revealed consistent relationships between controlled processing and time–frequency domain activity, i.e., event-related changes in the amplitude or phase of rhythmic neural firing patterns. Such high-resolution temporal relationships are as yet only beginning to be studied in relation to addiction (Rangaswamy et al., 2003), but may play a fundamental role in the mechanisms of overcoming effects of drug-related incentive salience, as oscillations in neural activity appear to be involved in working memory capacity (Benchenane et al., 2011, Kaminski et al., 2011, Moran et al., 2010), task set and movement preparation (Gladwin et al., 2006, Gladwin et al., 2008, Gladwin and De Jong, 2005) and the separation of subsets of information currently in working memory (Lisman, 2005, Sauseng et al., 2010).
One way to provide more insight into the decomposition of processes involved in dual-processing is by targeting the modulation of specific aspects of this model by training. Also from a clinical point of view, the obvious primary relevance of dual-processing models is their application to interventions. We therefore discuss in the next section training aimed at various elements of reflective and automatic processing.
6. Interventions
A number of novel interventions have recently been developed which attempt to influence the neurocognitive processes in addiction (Wiers et al., 2006, Wiers et al., 2008). One could wonder how realistic it is to somehow “train away” the consequences of possibly years of drug abuse (Wiers et al., 2004), or to attempt to compensate for biological immaturities. However, note that if controlled processing is indeed a dynamic set of interacting factors, then even small effects may have significant results; e.g., if participants learn to delay response selection even slightly, this may allow a qualitatively different set of responses to be selected and reinforced (Siegel, 1978). From the above perspective, interventions can be seen as beneficially biased learning environments, in which reinforcement can be provided that will either increase the chance of successful controlled processing, or reduce the need for it.
6.1. Working memory training
Regarding the first type of intervention, aimed at training the likelihood of successful control, there is mounting evidence for the efficacy of working memory (WM) training in groups with sub-optimal working memory, for example children with ADHD (Klingberg et al., 2005; see Klingberg, 2010). WM training has recently also been used successfully in addiction (Houben et al., 2011b), where it was found that it helped problem drinkers with strong positive memory associations for alcohol, presumably because it helped them to overcome the bias to approach alcohol, driven by these associations. A related method is the training of self-control, which has also shown beneficial effects on smoking cessation (Muraven, 2010).
Effects of WM training on the brain are only beginning to be determined, but it appears that generalized improvement may be due to increased frontoparietal connectivity and changes involving the basal ganglia (Klingberg, 2010). This suggests that the top-down biasing aspect of working memory, or executive control functions, as well as control of access to WM are trainable. The literature on the interplay between motivation and control processes suggests that the success of WM training may revolve around the synergy between such functions, and in particular the ability to appropriately delay responding.
In addition to behavioral WM training, there is also emerging evidence that low voltage electrical stimulation at the scalp (transcranial Direct Current Stimulation, or tDCS) can facilitate dorsolateral prefrontal activity, leading to enhanced working memory (Fregni et al., 2005) and reductions in craving for alcohol (Boggio et al., 2008), food (Fregni et al., 2008b) and cigarettes (Boggio et al., 2009, Fregni et al., 2008a). From the R3 perspective this could be explained in terms of increased ability to find an escape route from a craving cycle, as anodal prefrontal stimulation would be expected to facilitate the activation of necessary cognitive representations and top-down biasing. Since tDCS also influences neuronal plasticity (Nitsche et al., 2003, Paulus, 2004), a potentially important application would be the combination of tDCS with WM training or other methods. While the immediate effect of tDCS are temporary, its effects on concurrent training could be persistent, either via plasticity or by aiding the subject in performing the training task at a higher level of performance.
6.2. Cognitive bias modification
In addition to studies aimed at increasing the likelihood of successful controlled processing, research has focused on the development of novel interventions, termed cognitive bias modification (CBM), aimed at modulating biases that could underlie failures of controlled processing, including attentional biases (AB), approach biases, and evaluative memory-biases.
6.2.1. Attentional bias modification
Most work has been done on attentional bias modification, with promising clinical effects in anxiety and alcoholism. In this procedure, typically a modified version of an assessment instrument is used, with a contingency built in. For example, in a visual probe test, two stimuli (pictures or words; usually one related to the problem and one neutral) are presented at the same time, after which a probe appears on one of the two stimuli locations (e.g., an arrow pointing up or down). In an assessment instrument, the probe to which people react, appears equally often in the location of the disorder-related stimulus and the neutral stimulus. The attentional bias is then calculated by subtracting the reaction time on threat-trials from the reaction time to non-threat trials. In a manipulation or modification version of the task, a contingency is introduced, with the probe appearing less often on the location occupied by the disorder-related stimulus, while typically no contingency is built into the task in the control group. After promising preclinical studies (MacLeod et al., 2002), first successful clinical applications have been reported in targeted prevention (See et al., 2009) and in clinically anxious patient groups (Amir et al., 2009, Schmidt et al., 2009), and targeted prevention (See et al., 2009) with maintenance of clinical improvement reported four months after the training (Schmidt et al., 2009). In these clinical applications, typically multiple sessions of training are used, with a control group receiving continued assessment. A recent meta-analysis in the domain of anxiety found a medium effect size for this type of CBM (d = .61). The first controlled training CBM in the alcohol domain (Schoenmakers et al., 2010) found a change in patients’ attentional bias for alcohol after training (vs. irrelevant control training) and an effect on post-treatment abstinence after three months.
The first neurocognitive studies have been performed in this area, all in the domain of anxiety, investigating neurocognitive changes due to this type of training (Browning et al., 2010, Eldar and Bar-Haim, 2010). It appears that training attention towards or away from fear stimuli (angry faces) modifies relationships between lateral prefrontal and posterior (fusiform face area) regions, as evidenced by the need to recruit lateral prefrontal cortex to overcome the trained bias (Browning et al., 2010). From the perspective developed above, this increased activity suggests reprocessing due to the fast but incorrect attentional response caused by training. In general agreement with this finding, parieto-occipital P2 potentials evoked by task-irrelevant threatening stimuli have been found to be reduced by attentional training in anxious individuals (Eldar and Bar-Haim, 2010). This suggests an increase in the ability to disengage from, or to discontinue the reprocessing of, threatening information (Amenedo and Diaz, 1998). In upcoming studies we will investigate whether similar changes are found as a function of re-training in the addiction domain.
6.2.2. Approach bias modification
Recently, another cognitive bias in addiction has been addressed with re-training: an approach-bias for substance-related stimuli. This bias has been documented for a variety of substances with a variety of instruments: for cigarette-cues in smokers (Mogg et al., 2003), for marijuana-cues in marijuanausers (Field et al., 2006), and for alcohol in heavy drinkers (Field et al., 2008, Ostafin and Palfai, 2006, Palfai and Ostafin, 2003). Recently, Wiers et al. (2009b) developed the alcohol Approach Avoidance Task (alcohol-AAT), a task which since then has been adapted to be used as a re-training tool. Participants are required to respond by pushing or pulling a joystick, depending on a feature of the stimulus unrelated to the contents (e.g., picture format: landscape or portrait); for details, see the supporting information of Wiers et al. (2009b), online at http://onlinelibrary.wiley.com/doi/10.1111/j.1601-183X.2008.00454.x/suppinfo . The AAT contains a “zoom-feature” which has the effect that upon a pull movement, the picture size on the computer screen increases, and upon a push movement, it decreases, which generates a strong sense of approach and avoidance, respectively (Neumann and Strack, 2000), and disambiguates the meaning of pushing (is this encoded as “moving towards” or “pushing away?”) and pulling the joystick (Rinck and Becker, 2007). Using this task, it has been shown that both heavy drinkers (Wiers et al., 2009b) and alcoholic patients (Wiers et al., 2011) have an approach bias for alcohol. This bias was moderated by the g-allele of the OPRM1 gene: carriers of a g-allele demonstrated a particularly strong approach bias for alcohol, as well as for other appetitive stimuli (Wiers et al., 2009b). Using the same rationale as in attentional re-training, the alcohol-AAT was changed to a modification task, by changing the contingencies of the percentage of alcohol-related or control pictures that were presented in the format that was pulled or pushed. In a first preclinical study, a split design was used, with students being trained in one session to either approach alcohol (90% of the alcohol pictures came in the format to which a pull movement was made) or to avoid alcohol (90% came in the format to which a push movement was made) (Wiers et al., 2010). Generalized effects were found both in the same task (novel pictures, close generalization) and in a different task, employing words instead of pictures: an approach avoid alcohol Implicit Association Test (IAT; Greenwald et al., 1998). Hence, participants who had pulled most alcohol pictures (recall that the task instructions, however, only involved picture format) also became faster in pulling novel alcohol pictures and in categorizing alcohol with approach words, and the reverse was found for participants who had pushed most of the alcohol pictures. Those participants who demonstrated the change in approach bias in the expected direction drank less during a subsequent taste test. In a recent first clinical application of this novel approach-bias re-training paradigm (Wiers et al., 2011), 214 alcohol dependent patients were randomly assigned to one of two experimental conditions, in which they were explicitly or implicitly trained to make avoidance movements (pushing a joystick) in response to alcohol pictures, or to one of two control conditions, in which they received no training or sham training. Four brief sessions of experimental CBM preceded regular inpatient treatment (primarily cognitive behavioral therapy). In the experimental but not the control conditions, patients’ approach bias changed into an avoidance bias for alcohol. This effect generalized to untrained pictures in the task used in the CBM and to an IAT, in which alcohol and soft-drink words were categorized with approach and avoidance words. Patients in the experimental conditions showed better treatment outcomes a year later. Modeling work aimed at disentangling effects of training on controlled and automatic processing (cf. Conrey et al., 2005) is currently underway in our lab.
6.2.3. Further developments in intervention
In addition to these re-training paradigms, other paradigms have been developed which demonstrated promising results, such as evaluative conditioning (Houben et al., 2010), neurofeedback training (Sokhadze et al., 2008) and inhibition training in the context of problem-related stimuli (Houben et al., 2011a), but these procedures have as yet only been tested in student samples. Note that until now, all of these training-procedures, which yielded promising results in addiction, did so in adult samples (either students or adults with addiction problems; however, see Reyna, 2008, for training aimed at health-related decision-making in adolescents). One important issue, which is especially important in the context of adolescent addiction problems, is motivation to participate in a training (or more generally in any intervention). Remarkably, in most CBM approaches, patients both in the control group and in the experimental group think that they are in the control group (Beard, 2011). They find it hard to imagine that such a simple computer task can really help them with their problem. In adolescent substance use problems, the problem is even worse: first, most adolescents with substance use problems do not believe they have a problem (as an example, in one study Wiers and colleagues asked participants in the context of a family tree to indicate if they thought they had a drinking problem. While 75% of the sample of 96 late adolescents met criteria for substance use problems, only one individual indicated to have a problem, Wiers et al., 2005). Second, should they recognize that they have a problem (or at least that reducing substance use might not be a bad idea), they have to be convinced that it makes sense to do the training. There are different potential solutions to this problem. The first is to combine the training with more traditional motivational techniques. The second is to provide adolescents with global information about the efficacy of these training techniques. The third is to add game-like elements, such as a game-environment (as has been done successfully for working memory training in children with ADHD; Prins et al., 2011), and game elements, such as reaching higher levels (e.g., Fadardi and Cox, 2009). Clearly, these are promising new developments, but further research is needed to optimize these training techniques for adolescents.
7. Conclusion
The literature on adolescence dovetails with that on addiction, both in terms of the theoretical bases – imbalances are proposed that can be broadly described in terms of dual processes – but also in terms of likely substantial interactions: the vulnerabilities of adolescents map onto what makes addictive substances harmful. It was noted that concerns have been raised about the adequacy of dual-process models as a theoretical foundation. However, such concerns appear to be addressable in principle, as illustrated via the R3 model. In practical terms, dual-process models of addiction not only provide hypotheses why adolescents are at increased risk of substance abuse, but have also suggested interventions that have already been successfully applied in adults. Developments in dual-process models may provide stronger scientific grounding for cognitive bias modification as well as novel intervention targets, such as the conditioned delaying of response selection in risky situations. Future work should determine the efficacy of such interventions in adolescents, hopefully providing both clinical benefits and tests of novel models of decision making, reflective processing, adolescence, and addiction.
In conclusion, the integration of motivation and control appears critical not only to the development of individuals, but also to the further development of theory in cognitive neuroscience. In particular, the (mal-) adaptive learning processes leading to states of reflective and impulsive processing may be essential to understanding motivated behavior. This suggests a strong emphasis on data that not only demonstrate implicit motivation-related influences, but also reveal how they arise and hence perhaps how best to change them; and on studies that not only identify brain networks related to motivation and control, but that complement this information with the temporal dynamics between involved regions.