Abstract
We compared facets of criminogenic needs between male young offenders and university students in Hong Kong and assessed the interrelationships between criminogenic needs across the samples using network analysis. The participants, aged between 18 and 23 years old, were 183 incarcerated first-time offenders (age =19.6 years), 214 incarcerated repeated offenders (age =19.8 years), and 113 university students (age =19.6 years). The results show that incarcerated emerging adults had significantly greater criminogenic needs as compared with university students, while no difference between first-time and repeated offenders was found. Gang membership did not have main or moderating effects on criminogenic needs. Regularized Graphical Gaussian Models revealed that the criminogenic needs were clustered comparably across the three groups, while the network comparison test showed that the first-time and repeated-offender networks had significant structure invariance compared with the non-offender network. Differences in highly central nodes in terms of expected influence and bridge expected influence between networks were revealed.
Owing to the biopsychosocial development, emerging adulthood, that is, those between ages 18 to 25, encompasses distinct vulnerabilities along with tremendous opportunities for growth, which serves as a critical window for the treatment and prevention of criminological proclivities (Cauffman et al., 2023). To fight crime, criminal justice systems labor to prevent conviction and reduce recidivism (Brantingham & Faust, 1976).
The present study endeavored to contribute to such twofold judicial goals and the examination of the mechanisms of criminal offenses by comparing the criminogenic needs between non-offenders (i.e., those who were never convicted of a crime), first-time offenders (those who were convicted and were incarcerated for the first time), and repeated offenders (those who were convicted and incarcerated more than once).
The aforementioned judicial goals render the longstanding questions of what contributes to first-time conviction and what contributes to reoffending regardless of past legal punishments (Orlando & Farrington, 2021). Developmental criminologists have conducted longitudinal studies and identified numerous individual, family, and social risk factors that predict convictions (Cauffman et al., 2023). However, when predicting persistent convictions, the results are less robust. Meta-analyses demonstrate that anti-social personality, criminogenic needs, distress, family criminality, and substance use are statistically significant predictors of recidivism but with modest effect sizes (Katsiyannis et al., 2018). Even executive functions, a well-known risk factor of offending and recidivism (de Ribera et al., 2021), have yielded nonsignificant results from a recent meta-analysis (Griffith et al., 2024). In addition, what differentiates first-time and repeated offenders appeared to be largely personal historical factors, for instance, offense history, family history, past educational record, substance use history, and socioeconomic status (Orlando & Farrington, 2021). As such, there is large room for uncertainty when considering what can be done to facilitate desistance among repeated offenders.
Empirical evidence advocates the uptake of the Risk– Need–Responsivity (RNR) model to guide assessment and rehabilitation to facilitate desistance (Andrews et al., 1990; Bonta & Andrews, 2007). The RNR model consists of three core principles: the risk principle, which advocates for identifying the risk and the level of intervention should match the risk of recidivism (Andrews & Bonta, 2010); the need principle, which focuses on addressing the specific criminogenic needs that contribute to the criminal behavior (Andrews et al., 2011); and the responsivity principle, which emphasizes the customization of interventions to the learning styles, motivations, and capacities of the offenders (Craig et al., 2013).
Focusing on the criminogenic needs, which encompass static and dynamic factors, static factors are predetermined and retrospective in nature, such as histories of criminal conduct, violence, and substance abuse. On the other hand, dynamic factors are relatively changeable and fluid in nature, which comprise antisocial personality patterns (e.g., impulsivity and irritability), pro-criminal attitudes, social supports for crime (e.g., criminal friends), and substance abuse (Bonta & Andrews, 2007; Wooditch et al., 2014). Given that dynamic factors can be targeted in interventions (Bonta & Andrews, 2007; Chan et al., 2016), the present study aimed to examine the cognitive respect of the dynamic criminogenic needs across three groups during emerging adulthood: non-offenders, first-time offenders, and repeated offenders. In particular, we focused on pro-criminal attitudes, aggression-related cognition, social problem-solving skills, and attitudes toward substance use.
Pro-criminal attitudes
Criminal cognition has long been postulated to be one of the differentiating characteristics between offenders and non-offenders (McGuire & Priestley, 1995; Walters, 1990; Yochelson & Samenow, 1976). Neutralizing techniques may help justify antisocial behaviors against the justice system, by condemnation of the condemners and appealing to a higher authority. Denial of responsibility and denial of the victim for example may increase perceived justification of criminal offenses as well (Sykes & Matza, 1957). In addition to cognitive processing, cognitive social learning may play a role, learning criminogenic cognitions through interaction with others (Sutherland & Cressey, 1978), and thereby becoming more tolerant of committing crimes. Criminal sentiment, in general, entails attitudes towards the justice system, tolerance towards violations, and identification with the criminal others.
There are, however, mixed findings in the postulated sentiment–conviction link. Some studies have found an association between criminal sentiments and serious self-reported antisocial behaviors (Riopka et al., 2015), while others reported no differences between forensic and non--forensic samples (Dadgardoust et al., 2022). One of the three facets, tolerance for law violations, was even found to be related to a lower number of convictions in one study (Chui & Cheng, 2017). The mixed finding may be in part accounted for by the varying degree of deviance in their participants across studies, from self-reported antisocial behaviors (Riopka et al., 2015) to convictions (Chui & Cheng, 2017) and incarceration (Dadgardoust et al., 2022). It is to be examined if criminal sentiments can indeed account for this wide continuum of antisocial behaviors. As such, other cognitive factors ought to be explored.
Aggression-related cognitions
The complexity of aggression lies in its context, multiple forms, and interrelation of thinking patterns and behaviors. Static criminogenic needs, for example, history of abuse, parental rejection, and low SES, contribute to what the General Strain Theory (GST; Agnew & White, 1992) refers to as goal blockages, which give rise to frustration. Young adults may then result in deviant behaviors as a form of coping (Brezina, 2017). Furthermore, while the manifestation of anger may take physical or verbal forms, some aggression may be instrumental and hence reinforce action schema and cognitive inflexibility (Henwood et al., 2015). Most individuals, however, do not resort to criminal coping in response to anger. The cognitive appraisal of anger may therefore be a key to explain the differential responses to the strains (Brezina, 2017). Hence, in evaluating aggressive cognitions in the forensic context, trait anger, normative beliefs, behavioral scripts, and maladaptive schema should be considered (Allen et al., 2018; Gilbert et al., 2013).
Dadgardoust et al. (2022) revealed that endorsement of criminal cognitions is correlated with aggressive behaviors. Yet, aggressive cognitions were not evaluated. A metaanalysis also showed that anger management interventions are effective in reducing risks of violent reconviction (Henwood et al., 2015). However, most studies on aggressive cognitions were conducted with incarcerated youth (Henwood et al., 2015). It is unclear to what extent aggression-related cognitions differ between forensic and community populations and whether this helps explain the behavioral differences between the two groups.
Social problem-solving skills
Consistent with both the GST and General Personality and Social Psychological Perspective on Criminal Conduct, problem-solving competence may be linked to adaptive behaviors, whereas a deficit in prosocial competence may be related to antisocial behaviors (Andrews & Bonta, 2010; Brezina, 2017; Hayes, 2019). Those high on prosocial competence tend to be better at resolving interpersonal conflicts effectively, have better self-control, and a lower likelihood of externalizing unresolved issues as deviant behaviors or impulsive aggression (Curran & Bull, 2009).
To our understanding, most studies that concurrently investigated criminal sentiments and social problem-solving skills focused on adolescents with special learning needs (Curran & Bull, 2009; Lau et al., 2020), that is, those with possibly compromised social and learning abilities. It is unclear how great the difference in social problem-solving capacity is between offenders and non-offenders and between first-time and repeated offenders.
Attitudes towards substance use
Despite a significant number of substance-involved offences, the drug–crime relationship is notably complicated to determine (McBride & McCoy, 1993). On one hand, substance use may increase and sustain criminal behaviors, with elevated identification of criminal others, and thereby criminal sentiments (Walters, 2021). On the other hand, recreational drug users may increase crime engagement for illegitimate access to drugs (Simpson, 2003). Few studies have addressed the differential cognitive appraisal among forensic and community populations on substance use (McMullin & Gehlhaar, 1990).
To the best of our knowledge, despite the interconnectedness of criminal offences, aggression, problem-solving skills, and attitudes towards drug abuse, no study has investigated all four cognitive constructs concurrently and systematically. Also unknown are the differences in the pattern and strength of relationships among these constructs within the community and forensic populations (Borsboom, 2017).
Gang membership
In addition to these cognitive risk factors, gang membership is also a criminogenic need especially relevant to emerging adults. Moffitt (2018) proposes early adulthood as the critical stage for desistance from the criminal career, implying repeated offenders at this stage are prone to be life-course persistent. Gang involvement facilitates association with deviant peers and isolation from the prosocial counterparts. Persistent participation in the gang network provides the resources, skills, motivation, and reinforcement to commit crimes (Sutherland, 1947; Wooditch et al., 2014). As social control theory suggests, attachment and commitment to delinquent peers are likely to result in criminal behaviors (Hirschi, 1969). Indeed, gang affiliation was found to increase the odds of delinquency and is one of the most robust predictors of recidivism (Chui & Khiatani, 2018; MacRae et al., 2011). The extent to which gang (known as triad in Hong Kong) membership augments or interacts with the cognitive criminogenic needs of emerging young adults is yet to be empirically examined.
The network approach
For decades, developmental criminologists have been searching for the biomarkers of criminal behaviors for crime prevention and reducing recidivism. However, the results are mixed at best (Wolpe, 2013). The failure to identify specific biomarkers in mental disorders has helped engender the network theory of psychopathology, which hypothesizes that mental disorders arise from the direct interactions between symptoms and not from a latent cause or syndromal structure (Borsboom & Cramer, 2013).
Applying the network analytical approach to criminal behaviors shows that convictions arise from direct interactions between risk factors (van den Berg et al., 2020). In network analysis, variables are represented as nodes, whereas the statistical associations between variables are represented as edges. From the network perspective, strongly connected nodes (i.e., dynamic risk factors) tend to co-occur and co-vary and are highly probable to form a self-sustaining feedback loop that maintains the anomalous states. Thus, individuals who are vulnerable to a certain phenomenon should possess a more densely connected network, as compared with those who are more resilient (Borsboom, 2017). Meanwhile, highly central nodes are likely to influence other nodes in the network, thereby changing the network structure, which makes them potential targets for intervention. Compared to traditional bivariate or multivariate approaches, network analysis may, therefore, offer a more comprehensive and dynamic understanding of the cognitive architecture of the populations in question and identify potential clusters of constructs and the central component(s) amongst them.
Recently, there has been an increasing number of studies that adopt network analysis to understand the underlying mechanisms of criminal behaviors (e.g., Verkade et al., 2023; Zhang et al., 2022). However, only a few studies made use of the network comparison test (NCT) to examine the differences in network properties (i.e., network structure, network connectivity, edge weight, and node centrality) between subpopulations. Among the few that adopted NCT, Van den Berg et al. (2020) made comparisons between different types of sex offenders, Tsang et al. (2020) compared offenders with different levels of risk, and Verkade et al. (2023) examined the differences between non-offenders and offenders. To further elucidate the mechanisms of what contributes to first-time conviction and recidivism, there is value in comparing the network properties between non-offenders, first-time offenders, and repeated offenders.
Current study
Taken together, past literature suggests some aspects of cognitive and attitudinal factors might represent criminogenic needs that explain the differences between high- and low-risk adults in general. To further elucidate the differential criminogenic needs in emerging adulthood, in this study we compared male incarcerated emerging adults between the ages of 18 and 23, including both first-time and repeated offenders, and university students of the same age with no record of a criminal conviction. We compared their criminal sentiments, attitudes toward substance abuse, aggression, and social problem-solving skills. The moderation role of triad membership and its correlation with identification with criminal others were also tested. We hypothesized that (a) there are differences in the above constructs between the incarcerated young adults and the university students and between first-time and repeated offenders. We also hypothesized that (b) the differences between the first-time and repeated offenders were moderated by triad (i.e., criminal organizations) affiliation, and (c) triad affiliation was significantly associated with identification with criminal others.
Moreover, this study explored the criminogenic network among university students, first-time offenders, and repeated offenders. The interrelationships, cluster-to-cluster connectivity, and the centrality of the criminogenic needs were estimated using network analysis. We also compared the aforementioned network properties across the three groups to further examine the differences in criminogenic needs from a network perspective. The question we sought to answer was whether the cognitive criminogenic needs were connected differently (i.e., more loosely connected) in the university population than its forensic counterparts, such that the presence of particular risk factors was less likely to activate the relatively closely related pro-criminal attitudes or cognitions, thereby mitigating the possibility of coactivation in the surrounding yet loosely connected constructs. This allows us to infer factors that contribute to the resilience against criminal conviction. By the same token, we also sought to learn whether the forensic population network was constituted of more closely connected pro-criminal attitudes and conditions, rendering higher susceptibility and elevated coactivation of the constructs upon exposure to triggering events.
Method
Participants
In line with Epskamp et al. (2017), a stable network analysis requires as many observations as the number of parameters. In the current network with 13 nodes, it requires at least 91 participants (13 nodes +13 × 12/2 possible interactions).
Forensic and university student data were collected from the Hong Kong Correctional Services Department (CSD) and the University of Hong Kong, respectively, from 2015 to 2017. Ethics approval was received by the two institutions before the data were collected and analyzed. The CSD sample consisted of 430 respondents. At the time of admission, their age range was 16 to 23 (M = 19.52, SD =1.19). They were all male, and 205 of them were first-time offenders. The university sample consisted of 502 respondents, 27% of whom were male. The age ranged from 17 to 30 (M =19.26, SD =1.72). To minimize demographic heterogeneity for the purpose of meaningful comparison, in the current study, we only included male participants between the ages of 18 and 23 in our analyses. The final sample for analyses included 183 first-time offenders (Mage=19.61, SDage=1.10), 214 repeated offenders (Mage=19.78, SDage=1.06), and 113 university participants (Mage=19.59, SDage=1.50).
Procedures
CSD sample. Participation was voluntary, and written consent was obtained from all respondents. Self-report questionnaires were distributed by prison officers and research assistants. For offenders with limited literacy, the self-report questionnaire was administered in an audio format by a tape recorder. An attention check item was included in the questionnaire for quality control. Ten participants with straight-lining responses detected by the R package careless were excluded from the analysis to ensure data quality.
University sample. Undergraduate students were recruited through a participant pool system and mass email in 2015. All participating students were asked to complete an online questionnaire in either English or Traditional Chinese of their choice. Once completed, students would receive either course credits or a monetary compensation of $30 HKD (∼$3.9 USD) for their participation. Two attention check items were included in the questionnaire. Participants had to report whether they have ever committed a crime, and the number of convictions (including non-admission crimes). All participants in the university sample reported no conviction, indicating the entire university sample were non-offenders. Participants who failed to check the correct answer to either item were removed from the analysis. Nine participants with straight-lining responses detected by the R package careless were excluded from the analysis to ensure data quality.
Measures
Participants from the CSD sample and the university sample responded to the same set of measures. The criminal sentiments, including criminal attitudes towards law-court-police (LCP), tolerance for law violation (TLV), and identification with criminal others (ICO), were measured with the Criminal Sentiments Scale-Modified (CSS-M; Simourd, 1997). Thoughts and attitudes toward substance abuse were measured by Attitudes towards Drug Abuse (ADA), which was developed based on the McMullan Addiction Thoughts Scale (McMullin & Gehlhaar, 1990). Physical aggression (PA), verbal aggression (VA), anger (AG), and hostility (HT) were measured by the Aggression Questionnaire (Buss & Perry, 1992). Lastly, positive problem orientation (PPO), negative problem orientation (NPO), rational problem solving (RPS), impulsiveness/carelessness style (ICS), and avoidant style (AS) were measured by the Social Problem solving Inventory-Revised (SPSI-R; D’Zurilla et al., 1996).
Statistical analysis
All statistical analyses were completed using Jamovi and R (Version 4.1.0; R Core Team, 2021). Group comparisons between the forensic sample and the university sample on the demographics and target variables were conducted with Welch’s t-test. Comparisons between non-offenders, first-time offenders, and repeated offenders were conducted with Felch’s one-way ANOVA and Tukey post hoc test when homogeneity and normality assumptions were met. When not, Kruskal–Wallis one-way ANOVA and Dwass– Steel–Critchlow–Fligner post hoc test were used. Two-way ANOVA was used to compute the differences between nonoffenders and two types of offenders, moderated by triad membership. A point-biserial correlation test was used to examine the relationship between triad membership and ICO. The current analyses were performed based on scales and subscales with dropped items to improve internal consistency (Table S1). Another version of analyses computed with complete items is reported in Supporting Information Table S6 to Table S8. The R codes are available on Open Science Framework (https://osf.io/sp3yk/?view_only=a96 431391b7b47a4b8ebd07b05ff35f9).
Network analysis
Three partial correlation networks were estimated, namely the university student network, first-time-offender network, and repeated-offender network. For all networks, the bootnet package was used to estimate the network structures. The networks were estimated with the Extended Bayesian Information Criteria (EBIC) model selection and regularized with the graphical Least Absolute Shrinkage and Selection Operator (LASSO) algorithm. The EBIC tunning parameter (gamma) is set to 0.05. To further investigate the network structure, the spinglass community detection algorithm from the R package igraph was used to identify the community within each network. To quantify the functional role of each node in the networks, the expected influence and bridge expected influence of each node were computed using R package qgraph and network-tools, respectively.
The network robustness and stability were examined by nonparametric and case-dropping bootstraps with 10,000 iterations using the bootnet package. The stability analyses consisted of an edge-weight difference test, a centrality difference test, an edge-weight accuracy test, and correlation stability (CS) coefficients. Stable centrality metrics should have CS-coefficient not lower than 0.25 and preferably higher than 0.5 (Epskamp et al., 2017).
The network comparisons between the university student network, the first-time-offender network, and the repeatedoffender network were performed by the NCT function of the R package networkComparisonTest. The differences between networks were estimated with 10,000 iterations and Bonferroni–Holm correction. Comparisons across networks were made in terms of (a) network structure invariance, (b) global strength invariance, (c) edge strength invariance, and (d) centrality invariance.
For a more detailed description of the participants’ demographics, measures, and statistical analysis, see the Supporting Information.
Results
The comparisons in demographics between forensic and university samples, network stability results, and topological overlap checks of the three estimated networks are presented in Supporting Information.
Comparisons between forensic and university samples
Criminal attitudes, aggression, and social problem solving. The independent sample t-test results that compared the forensic sample and the university sample across all measures are summarized in Table 1. Considering the sum-score of CSSM, the forensic sample showed significantly higher general criminal attitude over the university sample with a large effect size. Consistent differences with large effect sizes between the two groups were found in the subscales. The LCP, TLV, and ICO were significantly higher in the forensic sample than the university sample. Regarding drug issues, the forensic sample expressed a significantly more liberal view toward drug abuse to a large extent compared with the university sample.
In terms of aggression, the forensic sample showed a significantly higher level of general aggression, physical aggression, and anger with a large effect size. Yet, the levels of verbal aggression and hostility were significantly higher in the forensic than in the university sample with a small-to-moderate effect size.
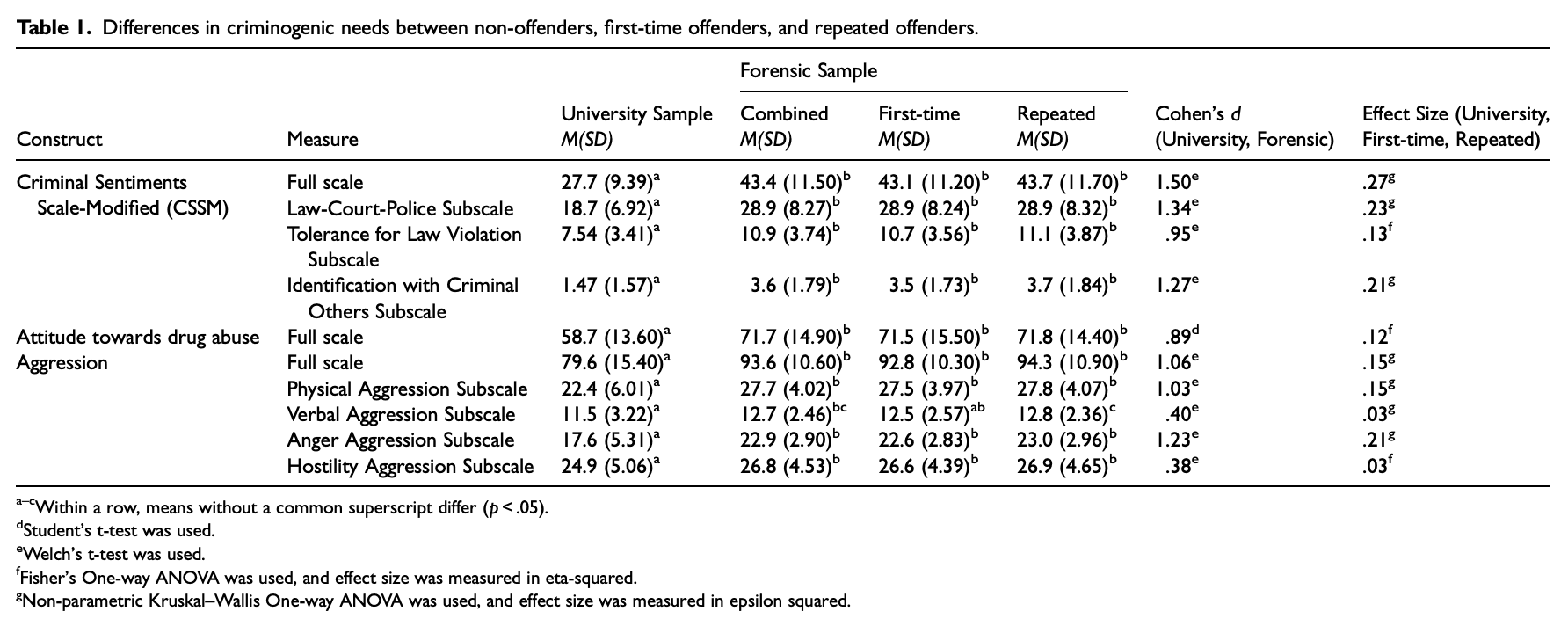
Comparisons between non-offenders, first-time offenders, and repeated offenders
Group differences are summarized in Table 1. There were significant and large differences across the three groups in terms of the sum-score of CSSM and the three subscale scores. Pairwise comparison post hoc tests showed that first-time offenders and repeated offenders were significantly higher in CSSM-sum score, LCP, TLV, and ICO, as compared with nonoffenders. However, there were no significant differences between first-time and repeated offenders regarding the sum-score of CSSM and its subscale. Similarly, there were significant differences with large effect sizes in ADA across three types of offending history. Whilst the first-time and repeated offenders showed significantly more liberal views toward drug abuse as compared with non-offenders, there was no significant difference between first-time and repeated offenders.
Regarding aggressiveness, there were significant differences with large effects across the three groups in terms of the sum-score of Aggression Questionnaire, physical aggression, and anger. Also, there were significant differences with small-to-moderate effect size across the three groups in verbal aggression and hostility. Pairwise comparison post hoc tests revealed that first-time and repeated offenders were significantly higher than non-offenders in Aggression Questionnaire sum-score, physical aggression, anger, and hostility. While there was no significant difference between non-offenders and first-time offenders in verbal aggression, repeated offenders reported significantly higher levels of verbal aggression than non-offenders. Lastly, there was no significant difference between first-time and repeated offenders regarding Aggression Questionnaire sum-score and its subscales.
Comparisons between first-time and repeated offenders, moderated by triad background
No main effect of triad membership on criminal attitudes, aggressions, and social problem solving was found. The two-way (offending history by triad membership) ANOVA results that compared first-time and repeated offenders, moderated by triad background, are summarized in Supporting Information Table S2. No group-by-membership interaction effect was found in criminal attitudes, aggressions, or social problem solving.
To further examine the role of triad background in firsttime and repeated offenders, point-biserial correlation test results indicated that there was no significant relationship between triad background and ICO in first-time offenders (r(166)=−.068, p=.385) or repeated offenders (r(205)= −.040, p=.569).
University student network
Network estimation. The regularized GGM network structure of the university sample is presented in Figure 1(a). The strongest edges appear between rational problem solving and positive problem orientation, followed by avoidant style and negative problem orientation. The spinglass community detection algorithm confirmed that the university student network was a disconnected network. As such, no community was identified from the network.
Centrality. Given that the university student network was a disconnected network, there was no identified community; thus, bridge expected influence was unavailable. The expected influence indices of all nodes are presented in Figure 2(a). Anger and avoidant style shared the highest expected influence, followed by physical aggression. LCP had the lowest expected influence among all nodes.
First-time-offender network
Network estimation. The regularized GGM network structure of the first-time offenders is presented in Figure 1(b). The strongest edges appear between Positive problem orientation and Rational problem solving, followed by LCP and TLV. The spinglass community detection algorithm identified four communities. The network consists of the criminal attitudes community (i.e., LCP, TLV, ICO, ADA), aggression community (i.e., physical aggression, verbal aggression, anger, hostility), adaptive problem solving (i.e., positive problem orientation, rational problem solving), and maladaptive problem solving (i.e., negative problem orientation, impulsiveness/carelessness style, avoidant style).
Centrality. The bridge expected influence indices of all nodes in the first-time-offender network are presented in Figure 3(a). Physical aggression and verbal aggression shared the highest bridge expected influence, followed by impulsiveness/carelessness style and ICO, whereas negative problem orientation had the lowest bridge expected influence. Moreover, the expected influence indices of all nodes in the network are presented in Figure 2(b). TLV also had the highest expected influence, followed by hostility. Again, LCP had the lowest expected influence among all nodes.
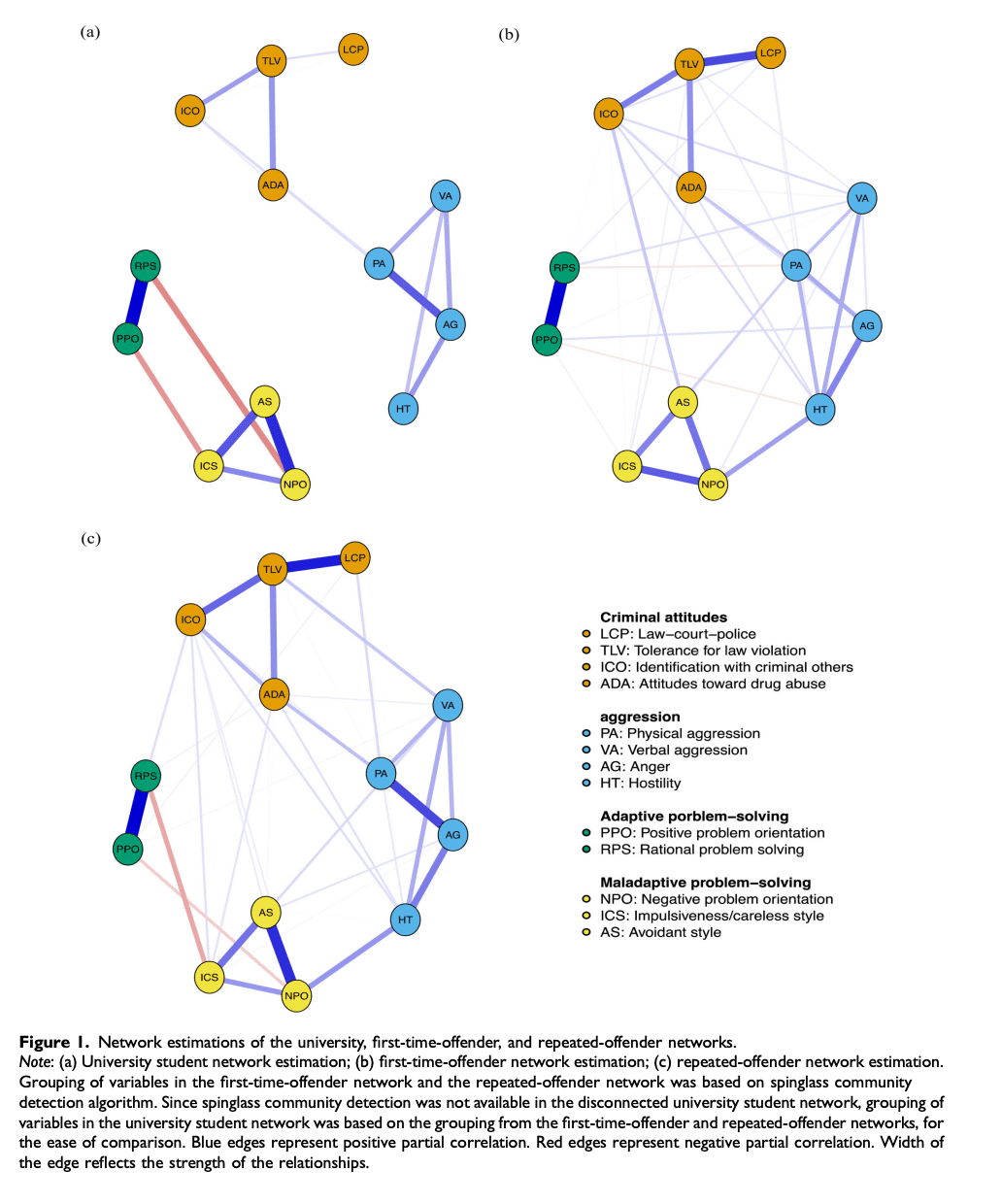
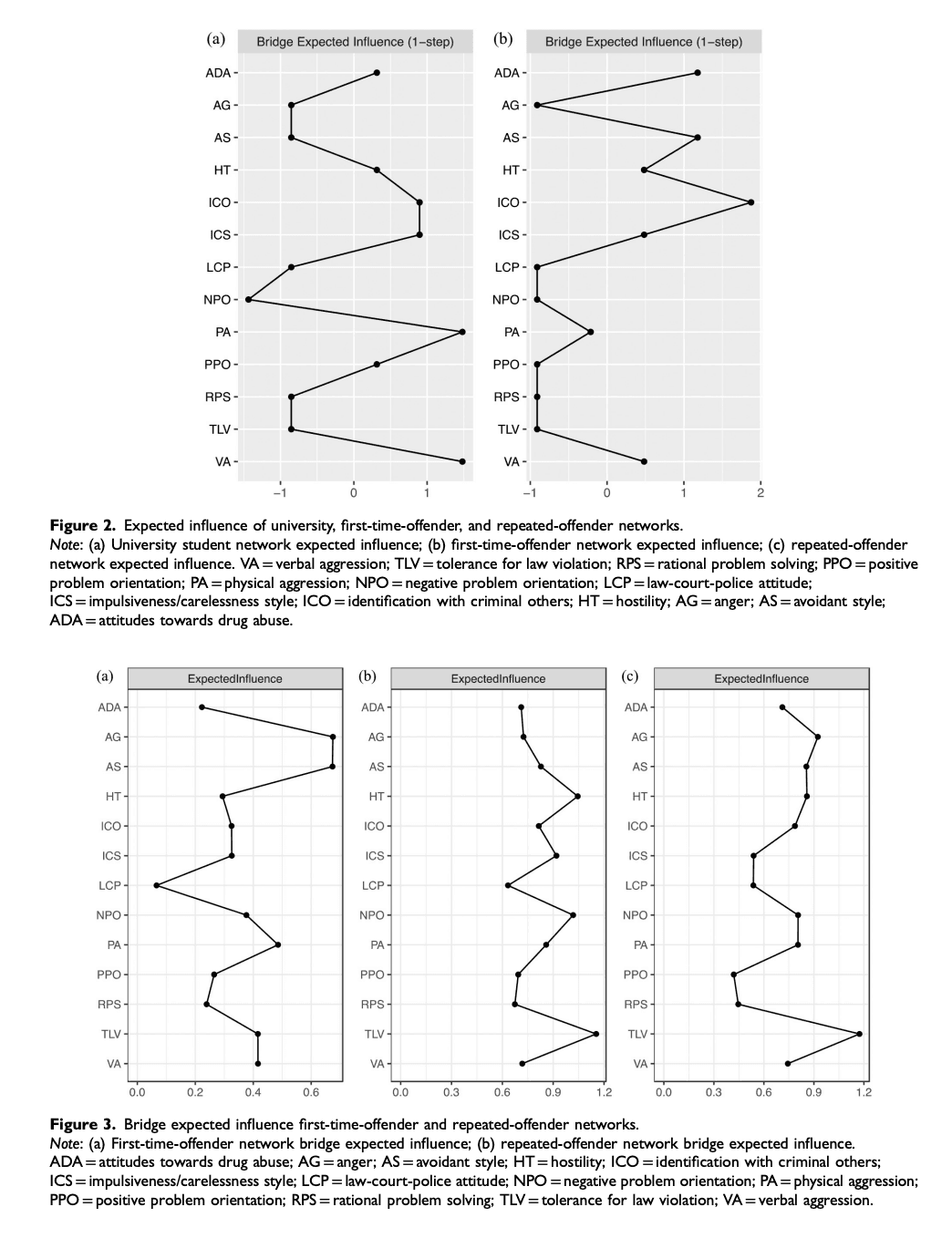
Repeated-offender network
Network estimation. The regularized GGM network structure of the repeated offenders is presented in Figure 1(c). The strongest edges appear between rational problem solving and positive problem orientation, LCP and TLV, as well as negative problem orientation and avoidant style. The spinglass community detection algorithm identified four communities with the same grouping of variables as in the first-time-offender network. The network consists of criminal attitudes community (i.e., LCP, TLV, ICO, ADA), aggression community (i.e., physical aggression, verbal aggression, anger, hostility), adaptive problem solving (i.e., positive problem orientation, rational problem solving), and maladaptive problem solving (i.e., negative problem orientation, impulsiveness/carelessness style, avoidant style).
Centrality. The bridge expected influence indices of all nodes in the repeated-offender network are presented in Figure 3(b). ICO had the highest bridge expected influence, followed by ADA and avoidant style. Moreover, the expected influence indices of all nodes in the network are presented in Figure 2(c). TLV had the highest expected influence, followed by anger. In addition, positive problem orientation had the lowest expected influence among all nodes.
Network comparisons
A summary of network results is presented in Table 2. Four aspects were used to evaluate the differences between the university student, first-time offender, and repeated-offender networks. First, the network structure invariance test revealed that the university student network had significant maximal edgeweight differences as compared with the first-time-offender (M = .384, p = .009) and the repeated-offender network (M = .421, p = .001), while no difference was obtained between first-time and repeated-offender networks (M =.183, p=.443).
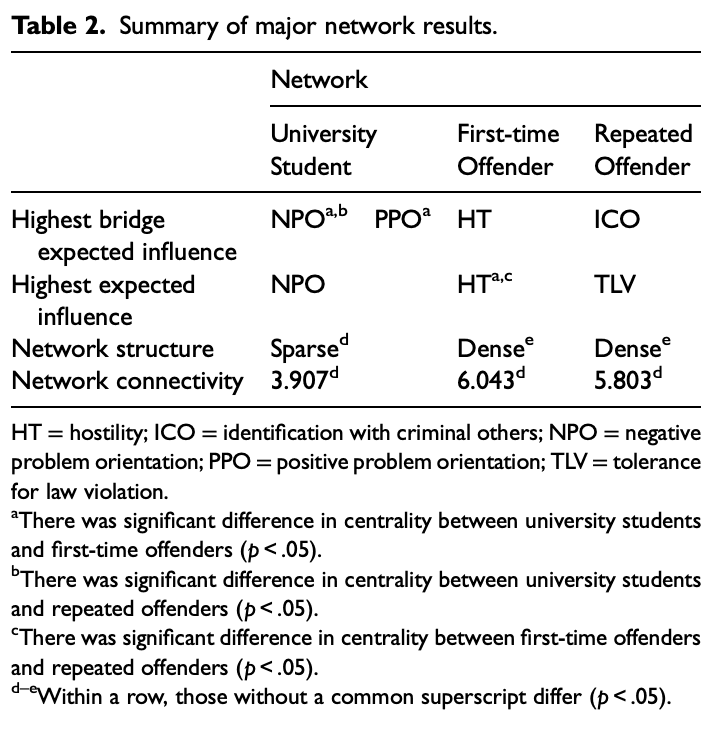
Second, the global strength invariance test indicated no significant difference in overall network connectivity between the university student network (global strength = 3.171), the first-time-offender network (global strength = 5.634), and the repeated-offender network (global strength=5.438).
Third, edge invariance test revealed that the edge between LCP and TLV, hostility and negative problem orientation, as well as positive problem orientation and impulsiveness/carelessness style, showed significantly stronger edge weight in the first-time and repeated-offender network than in the university student network. Meanwhile, the edge between negative problem orientation and rational problem solving was significantly stronger in the university student network than in the first-time and repeated-offender network. No significant edge invariance was identified between the first-time and repeatedoffender networks.
Fourth, centrality invariance test showed that LCP, TLV, positive problem orientation, negative problem orientation, rational problem solving, impulsiveness/carelessness style, and hostility had significantly lower expected influence in the university student network than in the first-time-offender network. However, no significant centrality invariance was identified between the non-offender network and repeatedoffender network, as well as between the first-time-offender network and the repeated-offender network.
Other details of the network comparison test results are summarized in Supporting Information Tables S3 to S5.
Discussion
This study examined the mean and network differences between incarcerated youths and same-age university students in terms of criminal sentiments, drug abuse attitudes, aggression, and social problem-solving style. The results revealed significant differences in all observed criminogenic needs between forensic and university samples, but there was no significant difference between first-time and repeated offenders. Triad membership did not moderate these differences.
Network analyses revealed the interrelationships between criminogenic needs among university students, first-time, and repeated offenders. Network comparison tests revealed invariance in network structure, several edge weights, and node centrality between the three networks, which suggest potential shifts in the functional role of criminogenic needs across the three populations.
Comparisons between forensic and university samples
Group differences between forensic and university samples were obtained on all the criminogenic needs included in this study. Not surprisingly, the forensic sample displayed a higher level of criminal sentiments, drug abuse attitudes, and aggression. These findings are consistent with the literature on criminal behaviors, in which offenders are characterized with a higher level of criminogenic needs as compared with nonoffenders, such as antisocial cognitions, and aggressionrelated personality traits (e.g., Banse et al., 2013; Grieger & Hosser, 2013; Skeem et al., 2014). Moreover, our findings also provide support to Chan’s (2019) results, which found that pro-criminal attitudes, deviant peer influences, liberal views toward drug abuse, and aggressiveness are related to offending. As highlighted by Taxman and Caudy (2015), individuals characterized by a high level of criminogenic needs and lifestyle destabilizers showed greater likelihood to offend, regardless of level of static risk. Thus, the current results provide further support for the notion that criminogenic needs can form a valid and reliable psychological profile of offenders.
Comparisons between university students, first-time offenders, and repeated offenders
This study segmented the forensic sample into first-time and repeated offenders, and compared between three samples on criminal sentiments, drug abuse attitudes, and aggression. No significant difference between first-time and repeated offenders was found. Inconsistent with Rajan and D’Souza’s (2018) findings that repeated offenders manifested higher levels of criminogenic needs than first-time offenders, our results showed that the two groups possessed similar levels of criminogenic needs. The current results suggest that not only repeated offenders but also first-time offenders require considerable attention if efforts were to be made in intervening in their criminogenic needs.
The non-differentiable level of criminogenic needs between first-time and repeated offenders may provide hints to the possible mechanism of conviction among young male offenders. According to the cumulative risk framework, criminal acts are the result of accumulated risk, including psychological and physiological predispositions and environmental adversities (Savolainen et al., 2018). As such, when people accumulate a certain level of risk, they are highly prone to offending, regardless of conviction history. In the context of this study, both firsttime and repeated offenders accumulated similar and high levels of criminogenic needs, resulting in offending behaviors.
The role of triad membership
Contrary to our prediction, triad membership had neither main nor interaction effects on the outcome variables and no significant relationship with ICO. In other words, firsttime and repeated offenders showed the same level of criminogenic needs, regardless of triad affiliations. Although it is well documented that triad membership is intimately linked to conviction and reconviction (MacRae et al., 2011; Pyrooz et al., 2015), our results suggest that triad membership does not exert a significant effect on young male offenders’ criminogenic needs. In addition, triad affiliations may only provide a platform to commit crime more accessibly, in which people with high criminogenic needs tend to offend and reoffend, regardless of the existence of attachments with any delinquent organization.
The nonsignificant effects of offending history and triad membership on the predisposing factors further strengthen the position that repeated offenders are not on a more extreme end of the criminogenic needs continuum as compared to first-time offenders. Given the high similarity between first-time and repeated offenders and a clear boundary between non-offenders and offenders, these results cohere with Sorge et al. (2015) that offenders may be separable from the continuum of antisocial behaviors among the university sample. On the other hand, it is also possible that the criminogenic needs included in this study have limited capacity in differentiating first-time and repeated offenders, as they may only capture the continuum of antisocial behaviors in the university sample, which can be helpful in identifying potential offenders. The findings also favor the dispositional theories of crime, as the fundamental differences between offenders and non-offenders were identified, while first-time and repeated offenders are indistinguishable. From the perspective of strain theory, the fundamental differences between offenders and non-offenders may result from the vast differences in life circumstances (Agnew & White, 1992), while offenders, regardless of conviction history, share similar life circumstances that cultivate similar attributes, thereby making first-time and repeated offenders less distinguishable from the psychological profile.
Network structure comparisons
Apart from differences in the level of criminogenic needs, this study investigated the interrelationships between the criminogenic needs of the three groups from a network perspective. The identified four communities were identical across the first-time and repeated-offender networks. Although no community can be statistically identified in the university student network given its disconnected nature, the university student network showed a similar pattern of connections with the forensic networks’ intracommunity connections. In general, all of the variables were grouped in a way that was consistent with their theoretical construct. Since attitudes toward drug abuse can reflect openness to the use of illegal substances (Wolff et al., 2013), it was intuitive that it was grouped into the criminal attitude community with other three criminal sentiments, the four types of aggression were grouped together, and the five problem-solving styles were separated into two communities in a way that match with their putatively adaptive and maladaptive nature. That the groupings of variables were identical across three networks reflects the fact that the criminogenic needs are meaningfully connected in the same pattern across two types of offenders.
The network structure invariance test revealed significant structural differences between the university student and first-time-offender networks, as well as the university student and repeated-offender network, while no significant structural differences were obtained between first-time and repeated-offender networks. As compared with the two offender networks, the university student network was disconnected and significantly sparser, in which there were only a few weak positive bridging edges between subgraphs (i.e., a group of connected nodes).1 Such a sparse network is similar to the resilient network of mental disorders presented by Borsboom (2017), in which a weakly connected network is resilient because the symptoms are less likely to be coactivated by external stimuli, and the symptoms are less likely to become self-sustaining even after the external stimuli are removed. In the university student network, criminogenic needs within the same subgraph may be coactivated, but not with criminogenic needs of other subgraphs. In this vein, even when certain criminogenic needs were triggered among university students, it is less probable that a series of cross-subgraphs of criminogenic needs become coactivated and self-sustaining to generate a strong intention to commit crimes or embedding criminal intentions into other aspects of cognitive functioning, such as problem-solving styles.
While there were significant network structure invariances between the three networks, no significant global strength invariance was obtained. These patterns of relationships imply that the intra-community edge strengths were highly similar across networks, and the combined weights of the new bridging edges do not produce significant differences in overall connectivity. Although there were more edges in the first-time and repeated-offender networks than the university student network, the edge-weight invariance test revealed that only four edges were significantly different across the networks. Three out of the four edges were bridging edges, which further supports the notion that the coactivations of cross-community criminogenic needs are differentiating offenders from university students. Meanwhile, the remaining edge between LCP and TLV was significantly stronger in the first-time offender and repeated-offender networks than in the university student network. As such, the increased strength of association between criminal attitudes may also characterize both first-time and repeated offenders.
Central nodes of networks
The centrality of the nodes in the three networks was computed to examine the functional role of each node. In the university student network, anger and avoidant style shared the highest expected influence as compared to other nodes. These results suggest that anger and avoidant style were the central characteristics of non-offenders’ criminogenic network. However, the problem-solving styles subgraph was not connected with the aggression and criminal attitude subgraph. Such a pattern of results suggested that possessing high criminal attitudes or aggressiveness may have limited or no influence on shaping certain types of problem-solving styles, and the adoption of either adaptive or maladaptive problem-solving styles appears to have limited capacity in triggering criminal attitudes and aggression. As a result, problem-solving styles, different forms of aggression, and criminal attitudes are unlikely to coactivate among university students, which may suggest that different clusters of criminogenic needs do not spill over and create strong motivations to commit crimes.
In the first-time-offender network, physical and verbal aggression shared the highest bridge expected influence, while TLV and hostility held the top two highest expected influences. These results suggest that first-time offenders are characterized by intense violence and aggression, while the TLV may play a role in normalizing the displays of aggressive behaviors. The first-time-offender network has numerous bridging edges, which suggests that activating one criminogenic need may lead to mass activations of the entire network of criminogenic needs, resulting in convictions. Notably, physical aggression and verbal aggression were positively linked to various maladaptive problem-solving strategies. As such, the display of aggression may be embedded into the problem-solving processes. Additionally, the centrality invariance test revealed that first-time offenders had significantly higher LCP, TLV, hostility, positive problem orientation, negative problem orientation, rational problem solving, and impulsiveness/ carelessness style than the university student network. These results may further support the notion that criminal attitudes, aggressions, and problem-solving styles are more likely to coactivate among first-time offenders compared with university students.
In the repeated-offender network, ICO obtained the highest bridge expected influence, which then appeared to be the central hub of criminogenic needs that connect with nodes in different communities. ICO also had a positive edge with rational problem solving, which may suggest that the decision to attached to deviant peers involves deliberate considerations, such as avoiding peer victimization and social alienation (Rudolph et al., 2014). On the other hand, ICO had no significant relationship with triad membership, which suggests that emotional attachment with criminals, as measured by ICO, and mere gang membership are not necessarily tied and that the former is potentially a more central predictor of recidivism than membership per se. This assertion, of course, warrants further investigation. ICO also had the strongest connection with TLV, and TLV was the node with the highest expected influence but the lowest bridge expected influence. These results suggest that TLV may not be responsible for coactivating other criminogenic needs but acts as a backbone of executing criminal behaviors, especially with deviant peers. In this vein, the criminal behaviors of repeated offenders may be largely influenced by deviant social relations and openness towards convictions.
Similarities and differences between first-time and repeated offenders
While profound differences were found between offenders and non-offenders, little differentiation was identified between first-time and repeated offenders. Such results may imply the networks of criminogenic needs can capture the tendency to offend, but little can be shown about the tendency to reoffend. Both types of offenders showed a network with multiple bridging edges, which suggests that convictions, regardless of quantities of convictions, can be driven by a densely positively connected criminogenic network. Meanwhile, both first-time and repeated offenders had a high expected influence in TLV, which may suggest that the rationalization and openness to law-breaking are important factors for convictions and reconvictions.
On the other hand, it appears that there was a shift in the relative importance of criminogenic needs across first-time offenders and repeated-offender networks. The mechanisms of criminal behaviors among first-time offenders were characterized by strong violence, whereas repeated offenders were characterized by identification with criminal others. These findings highlight the importance of addressing the strong aggressive impulses for preventing first conviction, and the cruciality of addressing the emotional attachment with criminal social relations for preventing recidivism. Unfortunately, given that four aspects of the network comparison test revealed no significant difference, there is scant evidence to distinctly differentiate between first-time and repeated offenders in the current study. Thus, such claims warrant further investigations.
Implications
To the best of our knowledge, this is the first study comparing the networks of criminogenic needs between university students, incarcerated first-time offenders, and incarcerated repeated offenders. A major finding of this study was the existence of inter-community (cross-clusters) connections between criminogenic needs that appear to differentiate offenders from university students. Moreover, we captured potential shifts between first-time and repeated offenders in central criminogenic needs from aggressive behaviors to identification with criminal others. Meanwhile, interventions targeting the inter-community connections between criminogenic needs, as well as the central criminogenic needs of each type of offender, may be more effective, but more replications and longitudinal studies are required to confirm these relationships and changes. Altogether, the current findings provide important insights for assessment accuracy and treatment directions for criminal behaviors.
Limitations and future directions
Despite the important theoretical and practical implications, this study has some limitations. First, there is limited information about offenders’ backgrounds, especially the type of offence that led to their incarceration. Although the current study captured the general trend and differences between offenders and university students, the findings may not be applicable to offenders with different types of offense. We did not have information regarding whether the offenders had completed the sentence at the time of responding to the survey, and any psychological interventions received during the course of sentence. Furthermore, we were unable to statistically account for the offenders’ demographic variables, such as educational level, socioeconomic status, and family background, as such information was not available. Given that different static risk factors and psychological interventions may have strong impacts on offenders’ psychological profiles, the current findings need to be interpreted cautiously.
Second, the small and disproportionate sample size may restrict the power of the network results and increase the chance of having false negative results. With a regularized network and several statistical adjustments, it is likely that our findings captured the existence of genuine relationships at the expense of a higher false negative rate, but the strength of the associations warrants cautious interpretation. In addition, some variables included in our study suffer from the problem of topological overlap (see Supporting Information, pp. S21, S28, S35). Although the variables are conceptually different, many of them are statistically similar, which requires special attention to the interpretations of detected associations, especially the intracommunity relationships.
Third, the cross-sectional nature of this study hinders us from drawing inferences regarding the directionality between different criminogenic needs and criminal behaviors. It remains uncertain whether the observed criminogenic needs are characteristics of offenders, predictors of convictions and reconvictions, or psychological outcomes of offending. Thus, future longitudinal studies with larger sample sizes on the transition processes from non-offenders to first-time offenders and repeated offenders are needed.
Lastly, the study may suffer from sampling biases. Regarding the incentive differences, the university students received research compensation (i.e., monetary reward or course credits) while the forensic sample did not receive any form of incentive, which may contribute to potential volunteer bias. In addition, given that this study heavily relied on self-reported information, potential self-report biases need to be considered.
Conclusion
This study endeavors to enrich the discussion of the nature of criminal behaviors by comparing the psychological profile between young non-offenders, first-time offenders, and repeated offenders. The results reveal that incarcerated offenders manifest a significantly higher level of criminogenic needs, and the network analyses revealed that they had more cross-community connections between criminogenic needs as compared with university students, while the boundary between first-time and repeated offenders is relatively blurry. These results underscore potential shifts in the functional roles of criminogenic needs. Preventive and intervention efforts can consider emphasizing on firsttime offenders’ aggressive behaviors, and repeated offenders’ criminal social networks.